Nested cross validation in combination with filter based feature selection Announcing the arrival of Valued Associate #679: Cesar Manara Planned maintenance scheduled April 17/18, 2019 at 00:00UTC (8:00pm US/Eastern) 2019 Moderator Election Q&A - Questionnaire 2019 Community Moderator Election ResultsDoes modeling with Random Forests require cross-validation?Sklearn feature selection stopping criterion (SelectFromModel)Variance in cross validation score / model selectionFeature selection in R too large datasetFeature selection: Information leaking if done before CV-split?k-fold cross-validation: model selection or variation in models when using k-fold cross validationFeature selectionSome confusions on Model selection using cross-validation approachNested cross-validation for regression over small datasetTarget encoding with cross validation
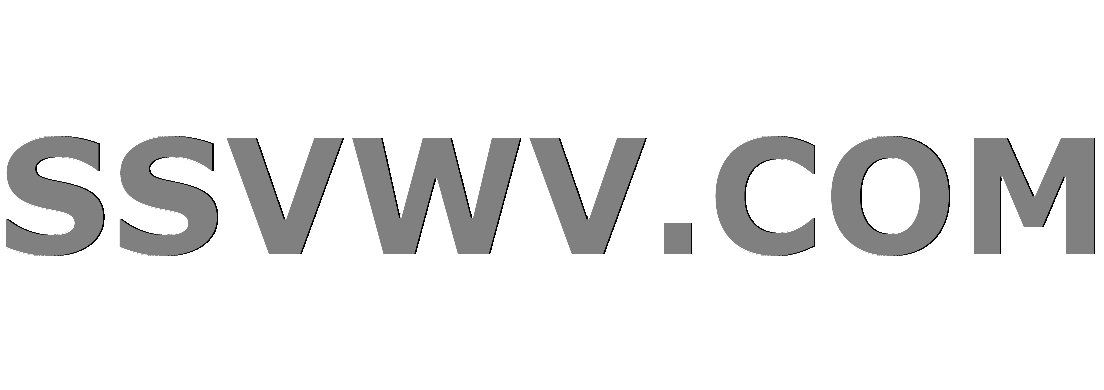
Multi tool use
What do you call a phrase that's not an idiom yet?
When to stop saving and start investing?
If 'B is more likely given A', then 'A is more likely given B'
I am not a queen, who am I?
Antler Helmet: Can it work?
Do you forfeit tax refunds/credits if you aren't required to and don't file by April 15?
When is phishing education going too far?
Were Kohanim forbidden from serving in King David's army?
What is the correct way to use the pinch test for dehydration?
What would be the ideal power source for a cybernetic eye?
Super Attribute Position on Product Page Magento 1
Dominant seventh chord in the major scale contains diminished triad of the seventh?
What is the longest distance a 13th-level monk can jump while attacking on the same turn?
Why don't the Weasley twins use magic outside of school if the Trace can only find the location of spells cast?
Gastric acid as a weapon
Check which numbers satisfy the condition [A*B*C = A! + B! + C!]
Letter Boxed validator
Why aren't air breathing engines used as small first stages
What are the motives behind Cersei's orders given to Bronn?
How discoverable are IPv6 addresses and AAAA names by potential attackers?
How do I stop a creek from eroding my steep embankment?
How can players work together to take actions that are otherwise impossible?
Why does Python start at index -1 when indexing a list from the end?
How to find all the available tools in macOS terminal?
Nested cross validation in combination with filter based feature selection
Announcing the arrival of Valued Associate #679: Cesar Manara
Planned maintenance scheduled April 17/18, 2019 at 00:00UTC (8:00pm US/Eastern)
2019 Moderator Election Q&A - Questionnaire
2019 Community Moderator Election ResultsDoes modeling with Random Forests require cross-validation?Sklearn feature selection stopping criterion (SelectFromModel)Variance in cross validation score / model selectionFeature selection in R too large datasetFeature selection: Information leaking if done before CV-split?k-fold cross-validation: model selection or variation in models when using k-fold cross validationFeature selectionSome confusions on Model selection using cross-validation approachNested cross-validation for regression over small datasetTarget encoding with cross validation
$begingroup$
So I have come across this paper that has defined nested cross validation as follows:
"Further, when one needs to use CV both for parameter selection (including feature selection) and for estimating the accuracy of the learned model, the CV procedure should be nested. That is, on each round of CV (outer CV), where the data is split into a training set consisting of K − 1 folds and the test set formed from the remaining fold, one performs also CV on this training set (inner CV) in order to select the learner parameters"
Here is a link to the paper this is provided in the supplementary materials
https://journals.plos.org/plosgenetics/article?id=10.1371/journal.pgen.1004754
I am particularly confused about the following about "when one needs to use CV both for parameter selection (including feature selection) and for estimating the accuracy of the learned model, the CV procedure should be nested."
So first here is how I understand nested cross validation to work without feature selection
1. Divide the data into K subsets.
2. Hold out one subset (testing) and use the K-1 subsets for model training
3. For a given parameter combination train the model on k-2 subsets and evaluate the performance on the remaining subset (validation).
4. Repeat this for all K-1 splits
5. repeat steps 3 and 4 for all parameter combinations
6. select the parameter combination that gives the best average performance on all k-1 datasets
7. Estimate training error on the hold out dataset
8. repeat steps 2-7 for all K subsets
Now lets say I want to incorporate some kind of filter based feature selection method such as the mutual information between a given feature and the target output.
So my inclination is to modify the above steps as follows
1. Divide the data into K subsets.
2. Hold out one subset for testing and use the K-1 subsets for model training
3. Select features on the K-1 training subsets
4. For a given parameter combination train the model on k-2 subsets and evaluate the performance on the remaining subset (validations set).
5. Repeat this for all K-1 splits in the training set
6. repeat steps 3 and 4 for all parameter combinations
7. select the parameter combination that gives the best average performance on all k-1 datasets
8. Estimate training error on the hold out dataset
9. repeat steps 2-8 for all K subsets
but the way it is described almost sounds like feature selection has to be done on the all k-2 subsets in step 4. This does not make much sense to me. First it is computationally inefficient, second how do you select optimal features when the features will change for every k-1 validation sets?
I do not know if may interpretation of the text is wrong or if there is a fault in my logic. Any help would be much appreciated.
feature-selection cross-validation parameter-estimation
$endgroup$
add a comment |
$begingroup$
So I have come across this paper that has defined nested cross validation as follows:
"Further, when one needs to use CV both for parameter selection (including feature selection) and for estimating the accuracy of the learned model, the CV procedure should be nested. That is, on each round of CV (outer CV), where the data is split into a training set consisting of K − 1 folds and the test set formed from the remaining fold, one performs also CV on this training set (inner CV) in order to select the learner parameters"
Here is a link to the paper this is provided in the supplementary materials
https://journals.plos.org/plosgenetics/article?id=10.1371/journal.pgen.1004754
I am particularly confused about the following about "when one needs to use CV both for parameter selection (including feature selection) and for estimating the accuracy of the learned model, the CV procedure should be nested."
So first here is how I understand nested cross validation to work without feature selection
1. Divide the data into K subsets.
2. Hold out one subset (testing) and use the K-1 subsets for model training
3. For a given parameter combination train the model on k-2 subsets and evaluate the performance on the remaining subset (validation).
4. Repeat this for all K-1 splits
5. repeat steps 3 and 4 for all parameter combinations
6. select the parameter combination that gives the best average performance on all k-1 datasets
7. Estimate training error on the hold out dataset
8. repeat steps 2-7 for all K subsets
Now lets say I want to incorporate some kind of filter based feature selection method such as the mutual information between a given feature and the target output.
So my inclination is to modify the above steps as follows
1. Divide the data into K subsets.
2. Hold out one subset for testing and use the K-1 subsets for model training
3. Select features on the K-1 training subsets
4. For a given parameter combination train the model on k-2 subsets and evaluate the performance on the remaining subset (validations set).
5. Repeat this for all K-1 splits in the training set
6. repeat steps 3 and 4 for all parameter combinations
7. select the parameter combination that gives the best average performance on all k-1 datasets
8. Estimate training error on the hold out dataset
9. repeat steps 2-8 for all K subsets
but the way it is described almost sounds like feature selection has to be done on the all k-2 subsets in step 4. This does not make much sense to me. First it is computationally inefficient, second how do you select optimal features when the features will change for every k-1 validation sets?
I do not know if may interpretation of the text is wrong or if there is a fault in my logic. Any help would be much appreciated.
feature-selection cross-validation parameter-estimation
$endgroup$
$begingroup$
Could you elaborate on where do you propose to apply "filter based feature selection" in your second approach? Step 3?
$endgroup$
– user12075
Apr 1 at 20:45
$begingroup$
"Could you elaborate on where do you propose to apply "filter based feature selection" in your second approach? Step 3?" Yes, the feature selection is performed on the union of all k-1 subsets before any parameter optimization is done. In other words feature selection is done on the whole training set while parameter optimization is only done on k-2 subsets and validated on the remaining subsets using all k-1 subsets to estimate the performance of a given parameter combination.
$endgroup$
– Joshua Mannheimer
Apr 1 at 20:51
add a comment |
$begingroup$
So I have come across this paper that has defined nested cross validation as follows:
"Further, when one needs to use CV both for parameter selection (including feature selection) and for estimating the accuracy of the learned model, the CV procedure should be nested. That is, on each round of CV (outer CV), where the data is split into a training set consisting of K − 1 folds and the test set formed from the remaining fold, one performs also CV on this training set (inner CV) in order to select the learner parameters"
Here is a link to the paper this is provided in the supplementary materials
https://journals.plos.org/plosgenetics/article?id=10.1371/journal.pgen.1004754
I am particularly confused about the following about "when one needs to use CV both for parameter selection (including feature selection) and for estimating the accuracy of the learned model, the CV procedure should be nested."
So first here is how I understand nested cross validation to work without feature selection
1. Divide the data into K subsets.
2. Hold out one subset (testing) and use the K-1 subsets for model training
3. For a given parameter combination train the model on k-2 subsets and evaluate the performance on the remaining subset (validation).
4. Repeat this for all K-1 splits
5. repeat steps 3 and 4 for all parameter combinations
6. select the parameter combination that gives the best average performance on all k-1 datasets
7. Estimate training error on the hold out dataset
8. repeat steps 2-7 for all K subsets
Now lets say I want to incorporate some kind of filter based feature selection method such as the mutual information between a given feature and the target output.
So my inclination is to modify the above steps as follows
1. Divide the data into K subsets.
2. Hold out one subset for testing and use the K-1 subsets for model training
3. Select features on the K-1 training subsets
4. For a given parameter combination train the model on k-2 subsets and evaluate the performance on the remaining subset (validations set).
5. Repeat this for all K-1 splits in the training set
6. repeat steps 3 and 4 for all parameter combinations
7. select the parameter combination that gives the best average performance on all k-1 datasets
8. Estimate training error on the hold out dataset
9. repeat steps 2-8 for all K subsets
but the way it is described almost sounds like feature selection has to be done on the all k-2 subsets in step 4. This does not make much sense to me. First it is computationally inefficient, second how do you select optimal features when the features will change for every k-1 validation sets?
I do not know if may interpretation of the text is wrong or if there is a fault in my logic. Any help would be much appreciated.
feature-selection cross-validation parameter-estimation
$endgroup$
So I have come across this paper that has defined nested cross validation as follows:
"Further, when one needs to use CV both for parameter selection (including feature selection) and for estimating the accuracy of the learned model, the CV procedure should be nested. That is, on each round of CV (outer CV), where the data is split into a training set consisting of K − 1 folds and the test set formed from the remaining fold, one performs also CV on this training set (inner CV) in order to select the learner parameters"
Here is a link to the paper this is provided in the supplementary materials
https://journals.plos.org/plosgenetics/article?id=10.1371/journal.pgen.1004754
I am particularly confused about the following about "when one needs to use CV both for parameter selection (including feature selection) and for estimating the accuracy of the learned model, the CV procedure should be nested."
So first here is how I understand nested cross validation to work without feature selection
1. Divide the data into K subsets.
2. Hold out one subset (testing) and use the K-1 subsets for model training
3. For a given parameter combination train the model on k-2 subsets and evaluate the performance on the remaining subset (validation).
4. Repeat this for all K-1 splits
5. repeat steps 3 and 4 for all parameter combinations
6. select the parameter combination that gives the best average performance on all k-1 datasets
7. Estimate training error on the hold out dataset
8. repeat steps 2-7 for all K subsets
Now lets say I want to incorporate some kind of filter based feature selection method such as the mutual information between a given feature and the target output.
So my inclination is to modify the above steps as follows
1. Divide the data into K subsets.
2. Hold out one subset for testing and use the K-1 subsets for model training
3. Select features on the K-1 training subsets
4. For a given parameter combination train the model on k-2 subsets and evaluate the performance on the remaining subset (validations set).
5. Repeat this for all K-1 splits in the training set
6. repeat steps 3 and 4 for all parameter combinations
7. select the parameter combination that gives the best average performance on all k-1 datasets
8. Estimate training error on the hold out dataset
9. repeat steps 2-8 for all K subsets
but the way it is described almost sounds like feature selection has to be done on the all k-2 subsets in step 4. This does not make much sense to me. First it is computationally inefficient, second how do you select optimal features when the features will change for every k-1 validation sets?
I do not know if may interpretation of the text is wrong or if there is a fault in my logic. Any help would be much appreciated.
feature-selection cross-validation parameter-estimation
feature-selection cross-validation parameter-estimation
asked Apr 1 at 20:26
Joshua MannheimerJoshua Mannheimer
1
1
$begingroup$
Could you elaborate on where do you propose to apply "filter based feature selection" in your second approach? Step 3?
$endgroup$
– user12075
Apr 1 at 20:45
$begingroup$
"Could you elaborate on where do you propose to apply "filter based feature selection" in your second approach? Step 3?" Yes, the feature selection is performed on the union of all k-1 subsets before any parameter optimization is done. In other words feature selection is done on the whole training set while parameter optimization is only done on k-2 subsets and validated on the remaining subsets using all k-1 subsets to estimate the performance of a given parameter combination.
$endgroup$
– Joshua Mannheimer
Apr 1 at 20:51
add a comment |
$begingroup$
Could you elaborate on where do you propose to apply "filter based feature selection" in your second approach? Step 3?
$endgroup$
– user12075
Apr 1 at 20:45
$begingroup$
"Could you elaborate on where do you propose to apply "filter based feature selection" in your second approach? Step 3?" Yes, the feature selection is performed on the union of all k-1 subsets before any parameter optimization is done. In other words feature selection is done on the whole training set while parameter optimization is only done on k-2 subsets and validated on the remaining subsets using all k-1 subsets to estimate the performance of a given parameter combination.
$endgroup$
– Joshua Mannheimer
Apr 1 at 20:51
$begingroup$
Could you elaborate on where do you propose to apply "filter based feature selection" in your second approach? Step 3?
$endgroup$
– user12075
Apr 1 at 20:45
$begingroup$
Could you elaborate on where do you propose to apply "filter based feature selection" in your second approach? Step 3?
$endgroup$
– user12075
Apr 1 at 20:45
$begingroup$
"Could you elaborate on where do you propose to apply "filter based feature selection" in your second approach? Step 3?" Yes, the feature selection is performed on the union of all k-1 subsets before any parameter optimization is done. In other words feature selection is done on the whole training set while parameter optimization is only done on k-2 subsets and validated on the remaining subsets using all k-1 subsets to estimate the performance of a given parameter combination.
$endgroup$
– Joshua Mannheimer
Apr 1 at 20:51
$begingroup$
"Could you elaborate on where do you propose to apply "filter based feature selection" in your second approach? Step 3?" Yes, the feature selection is performed on the union of all k-1 subsets before any parameter optimization is done. In other words feature selection is done on the whole training set while parameter optimization is only done on k-2 subsets and validated on the remaining subsets using all k-1 subsets to estimate the performance of a given parameter combination.
$endgroup$
– Joshua Mannheimer
Apr 1 at 20:51
add a comment |
0
active
oldest
votes
Your Answer
StackExchange.ready(function()
var channelOptions =
tags: "".split(" "),
id: "557"
;
initTagRenderer("".split(" "), "".split(" "), channelOptions);
StackExchange.using("externalEditor", function()
// Have to fire editor after snippets, if snippets enabled
if (StackExchange.settings.snippets.snippetsEnabled)
StackExchange.using("snippets", function()
createEditor();
);
else
createEditor();
);
function createEditor()
StackExchange.prepareEditor(
heartbeatType: 'answer',
autoActivateHeartbeat: false,
convertImagesToLinks: false,
noModals: true,
showLowRepImageUploadWarning: true,
reputationToPostImages: null,
bindNavPrevention: true,
postfix: "",
imageUploader:
brandingHtml: "Powered by u003ca class="icon-imgur-white" href="https://imgur.com/"u003eu003c/au003e",
contentPolicyHtml: "User contributions licensed under u003ca href="https://creativecommons.org/licenses/by-sa/3.0/"u003ecc by-sa 3.0 with attribution requiredu003c/au003e u003ca href="https://stackoverflow.com/legal/content-policy"u003e(content policy)u003c/au003e",
allowUrls: true
,
onDemand: true,
discardSelector: ".discard-answer"
,immediatelyShowMarkdownHelp:true
);
);
Sign up or log in
StackExchange.ready(function ()
StackExchange.helpers.onClickDraftSave('#login-link');
);
Sign up using Google
Sign up using Facebook
Sign up using Email and Password
Post as a guest
Required, but never shown
StackExchange.ready(
function ()
StackExchange.openid.initPostLogin('.new-post-login', 'https%3a%2f%2fdatascience.stackexchange.com%2fquestions%2f48373%2fnested-cross-validation-in-combination-with-filter-based-feature-selection%23new-answer', 'question_page');
);
Post as a guest
Required, but never shown
0
active
oldest
votes
0
active
oldest
votes
active
oldest
votes
active
oldest
votes
Thanks for contributing an answer to Data Science Stack Exchange!
- Please be sure to answer the question. Provide details and share your research!
But avoid …
- Asking for help, clarification, or responding to other answers.
- Making statements based on opinion; back them up with references or personal experience.
Use MathJax to format equations. MathJax reference.
To learn more, see our tips on writing great answers.
Sign up or log in
StackExchange.ready(function ()
StackExchange.helpers.onClickDraftSave('#login-link');
);
Sign up using Google
Sign up using Facebook
Sign up using Email and Password
Post as a guest
Required, but never shown
StackExchange.ready(
function ()
StackExchange.openid.initPostLogin('.new-post-login', 'https%3a%2f%2fdatascience.stackexchange.com%2fquestions%2f48373%2fnested-cross-validation-in-combination-with-filter-based-feature-selection%23new-answer', 'question_page');
);
Post as a guest
Required, but never shown
Sign up or log in
StackExchange.ready(function ()
StackExchange.helpers.onClickDraftSave('#login-link');
);
Sign up using Google
Sign up using Facebook
Sign up using Email and Password
Post as a guest
Required, but never shown
Sign up or log in
StackExchange.ready(function ()
StackExchange.helpers.onClickDraftSave('#login-link');
);
Sign up using Google
Sign up using Facebook
Sign up using Email and Password
Post as a guest
Required, but never shown
Sign up or log in
StackExchange.ready(function ()
StackExchange.helpers.onClickDraftSave('#login-link');
);
Sign up using Google
Sign up using Facebook
Sign up using Email and Password
Sign up using Google
Sign up using Facebook
Sign up using Email and Password
Post as a guest
Required, but never shown
Required, but never shown
Required, but never shown
Required, but never shown
Required, but never shown
Required, but never shown
Required, but never shown
Required, but never shown
Required, but never shown
tQ9ebEfB9n
$begingroup$
Could you elaborate on where do you propose to apply "filter based feature selection" in your second approach? Step 3?
$endgroup$
– user12075
Apr 1 at 20:45
$begingroup$
"Could you elaborate on where do you propose to apply "filter based feature selection" in your second approach? Step 3?" Yes, the feature selection is performed on the union of all k-1 subsets before any parameter optimization is done. In other words feature selection is done on the whole training set while parameter optimization is only done on k-2 subsets and validated on the remaining subsets using all k-1 subsets to estimate the performance of a given parameter combination.
$endgroup$
– Joshua Mannheimer
Apr 1 at 20:51