Choosing the first node in a decision tree, basic example2019 Community Moderator ElectionQuestion on decision tree in the book Programming Collective IntelligenceDecision Tree EnsemblingIs it acceptable to select a random child node when using a Decision Tree (trained via ID3) to predict if an unknown attribute value is encounteredWhy is my classification tree is showing only one node, and how to resolve this issue?Decision tree orderingHow are boosted decision stumps different from a decision tree?Do C 4.5 or C 5.0 perform multi way split or binary split?Decision Tree: Efficient splitting of nodes, minimize number of gini evaluationsDecision Trees How to Calculate Entropy Gain for Continuous Values for split point?
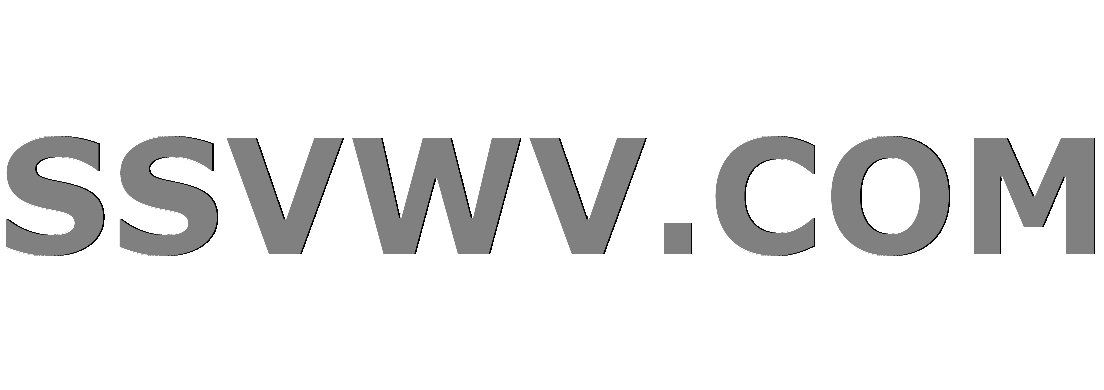
Multi tool use
Can a planet have a different gravitational pull depending on its location in orbit around its sun?
Is "plugging out" electronic devices an American expression?
Are objects structures and/or vice versa?
How to move the player while also allowing forces to affect it
How can I plot a Farey diagram?
What does 'script /dev/null' do?
Unbreakable Formation vs. Cry of the Carnarium
What to wear for invited talk in Canada
New order #4: World
Typesetting a double Over Dot on top of a symbol
Why do we use polarized capacitors?
Is there a way to make member function NOT callable from constructor?
Can I legally use front facing blue light in the UK?
Is this food a bread or a loaf?
What causes the sudden spool-up sound from an F-16 when enabling afterburner?
Domain expired, GoDaddy holds it and is asking more money
Symmetry in quantum mechanics
aging parents with no investments
"My colleague's body is amazing"
Filling an area between two curves
What is the meaning of "of trouble" in the following sentence?
Is Social Media Science Fiction?
How can I fix this gap between bookcases I made?
LWC and complex parameters
Choosing the first node in a decision tree, basic example
2019 Community Moderator ElectionQuestion on decision tree in the book Programming Collective IntelligenceDecision Tree EnsemblingIs it acceptable to select a random child node when using a Decision Tree (trained via ID3) to predict if an unknown attribute value is encounteredWhy is my classification tree is showing only one node, and how to resolve this issue?Decision tree orderingHow are boosted decision stumps different from a decision tree?Do C 4.5 or C 5.0 perform multi way split or binary split?Decision Tree: Efficient splitting of nodes, minimize number of gini evaluationsDecision Trees How to Calculate Entropy Gain for Continuous Values for split point?
$begingroup$
I'm wondering whether I'm understanding the process of choosing a node correctly and would like to see if this example makes sense.
using the following data :
> df
Y A B C
1 1 0 1 1
2 0 0 0 1
3 1 1 1 0
4 1 0 1 0
5 1 1 0 1
6 1 0 0 1
I will split the data on A,B,C and evaluate the entropy of each split, where the entropy is computed using
$$
p ; log_2(p) + (1-p); log_2(1-p)
$$
where $p$ is the proportion of successes for a particular split.
When splitting on A I have
Y A B C
3 1 1 1 0
5 1 1 0 1
and
Y A B C
1 1 0 1 1
2 0 0 0 1
4 1 0 1 0
6 1 0 0 1
For $A = 1$ (the first data table) I have $p = 1$, then entropy is 0.
for $A=0$ (second table) I have $p=0.75$ and entropy 0.81
So for splitting on $A$ I would state that the entropy is
$$
0 + 0.81 = 0.81
$$
This is then carried out in a similar manner for $B,C$.
For $B=1$ I find $p=1$ so entropy = 1
For $B=0$ I find $p=0.66$ so entropy = 0.91
Then the entropy for splitting on $B$ is
$$
0 + 0.91 = 0.91
$$
For $C=1$ I find $p=0.75$ so entropy = 0.81
For $C=0$ I find $p=1$ so entropy = 0
Then the entropy for splitting on $C$ is
$$
0.81 + 0 = 0.81
$$
Given the above the split which has the highest entropy is $B$, therefore I would choose to split on $B$ first.
I now have a decision tree with one node, and need to select the next node for each branch of $B = 1$ and $B=0$.
This selection is carried out in the same manner as the above.
Is the computation and reasoning in the above valid?
machine-learning decision-trees information-theory
$endgroup$
add a comment |
$begingroup$
I'm wondering whether I'm understanding the process of choosing a node correctly and would like to see if this example makes sense.
using the following data :
> df
Y A B C
1 1 0 1 1
2 0 0 0 1
3 1 1 1 0
4 1 0 1 0
5 1 1 0 1
6 1 0 0 1
I will split the data on A,B,C and evaluate the entropy of each split, where the entropy is computed using
$$
p ; log_2(p) + (1-p); log_2(1-p)
$$
where $p$ is the proportion of successes for a particular split.
When splitting on A I have
Y A B C
3 1 1 1 0
5 1 1 0 1
and
Y A B C
1 1 0 1 1
2 0 0 0 1
4 1 0 1 0
6 1 0 0 1
For $A = 1$ (the first data table) I have $p = 1$, then entropy is 0.
for $A=0$ (second table) I have $p=0.75$ and entropy 0.81
So for splitting on $A$ I would state that the entropy is
$$
0 + 0.81 = 0.81
$$
This is then carried out in a similar manner for $B,C$.
For $B=1$ I find $p=1$ so entropy = 1
For $B=0$ I find $p=0.66$ so entropy = 0.91
Then the entropy for splitting on $B$ is
$$
0 + 0.91 = 0.91
$$
For $C=1$ I find $p=0.75$ so entropy = 0.81
For $C=0$ I find $p=1$ so entropy = 0
Then the entropy for splitting on $C$ is
$$
0.81 + 0 = 0.81
$$
Given the above the split which has the highest entropy is $B$, therefore I would choose to split on $B$ first.
I now have a decision tree with one node, and need to select the next node for each branch of $B = 1$ and $B=0$.
This selection is carried out in the same manner as the above.
Is the computation and reasoning in the above valid?
machine-learning decision-trees information-theory
$endgroup$
add a comment |
$begingroup$
I'm wondering whether I'm understanding the process of choosing a node correctly and would like to see if this example makes sense.
using the following data :
> df
Y A B C
1 1 0 1 1
2 0 0 0 1
3 1 1 1 0
4 1 0 1 0
5 1 1 0 1
6 1 0 0 1
I will split the data on A,B,C and evaluate the entropy of each split, where the entropy is computed using
$$
p ; log_2(p) + (1-p); log_2(1-p)
$$
where $p$ is the proportion of successes for a particular split.
When splitting on A I have
Y A B C
3 1 1 1 0
5 1 1 0 1
and
Y A B C
1 1 0 1 1
2 0 0 0 1
4 1 0 1 0
6 1 0 0 1
For $A = 1$ (the first data table) I have $p = 1$, then entropy is 0.
for $A=0$ (second table) I have $p=0.75$ and entropy 0.81
So for splitting on $A$ I would state that the entropy is
$$
0 + 0.81 = 0.81
$$
This is then carried out in a similar manner for $B,C$.
For $B=1$ I find $p=1$ so entropy = 1
For $B=0$ I find $p=0.66$ so entropy = 0.91
Then the entropy for splitting on $B$ is
$$
0 + 0.91 = 0.91
$$
For $C=1$ I find $p=0.75$ so entropy = 0.81
For $C=0$ I find $p=1$ so entropy = 0
Then the entropy for splitting on $C$ is
$$
0.81 + 0 = 0.81
$$
Given the above the split which has the highest entropy is $B$, therefore I would choose to split on $B$ first.
I now have a decision tree with one node, and need to select the next node for each branch of $B = 1$ and $B=0$.
This selection is carried out in the same manner as the above.
Is the computation and reasoning in the above valid?
machine-learning decision-trees information-theory
$endgroup$
I'm wondering whether I'm understanding the process of choosing a node correctly and would like to see if this example makes sense.
using the following data :
> df
Y A B C
1 1 0 1 1
2 0 0 0 1
3 1 1 1 0
4 1 0 1 0
5 1 1 0 1
6 1 0 0 1
I will split the data on A,B,C and evaluate the entropy of each split, where the entropy is computed using
$$
p ; log_2(p) + (1-p); log_2(1-p)
$$
where $p$ is the proportion of successes for a particular split.
When splitting on A I have
Y A B C
3 1 1 1 0
5 1 1 0 1
and
Y A B C
1 1 0 1 1
2 0 0 0 1
4 1 0 1 0
6 1 0 0 1
For $A = 1$ (the first data table) I have $p = 1$, then entropy is 0.
for $A=0$ (second table) I have $p=0.75$ and entropy 0.81
So for splitting on $A$ I would state that the entropy is
$$
0 + 0.81 = 0.81
$$
This is then carried out in a similar manner for $B,C$.
For $B=1$ I find $p=1$ so entropy = 1
For $B=0$ I find $p=0.66$ so entropy = 0.91
Then the entropy for splitting on $B$ is
$$
0 + 0.91 = 0.91
$$
For $C=1$ I find $p=0.75$ so entropy = 0.81
For $C=0$ I find $p=1$ so entropy = 0
Then the entropy for splitting on $C$ is
$$
0.81 + 0 = 0.81
$$
Given the above the split which has the highest entropy is $B$, therefore I would choose to split on $B$ first.
I now have a decision tree with one node, and need to select the next node for each branch of $B = 1$ and $B=0$.
This selection is carried out in the same manner as the above.
Is the computation and reasoning in the above valid?
machine-learning decision-trees information-theory
machine-learning decision-trees information-theory
asked Mar 29 at 10:37


baxxbaxx
1314
1314
add a comment |
add a comment |
1 Answer
1
active
oldest
votes
$begingroup$
Some corrections in the above:
- While calculating entropy of a split, you should take into account the weights - fraction of instances in each branch:
$$
entropy(A) = 0 * (2/6) + 0.81 * (4/6) = 0.54\
entropy(B) = 0 * (3/6) + 0.91 * (3/6) = 0.45\
entropy(C) = 0 * (2/6) + 0.81 * (4/6) = 0.54
$$
- While choosing an attribute to split, you should choose the one with lowest entropy after the split. Entropy is the uncertainty, so you want to minimise it. As an example, if the split was perfect (all 0's on one side, and all 1's on the other), then entropy will be 0.
After selection of one node, the tree is built out recursively, as you mention.
$endgroup$
add a comment |
Your Answer
StackExchange.ifUsing("editor", function ()
return StackExchange.using("mathjaxEditing", function ()
StackExchange.MarkdownEditor.creationCallbacks.add(function (editor, postfix)
StackExchange.mathjaxEditing.prepareWmdForMathJax(editor, postfix, [["$", "$"], ["\\(","\\)"]]);
);
);
, "mathjax-editing");
StackExchange.ready(function()
var channelOptions =
tags: "".split(" "),
id: "557"
;
initTagRenderer("".split(" "), "".split(" "), channelOptions);
StackExchange.using("externalEditor", function()
// Have to fire editor after snippets, if snippets enabled
if (StackExchange.settings.snippets.snippetsEnabled)
StackExchange.using("snippets", function()
createEditor();
);
else
createEditor();
);
function createEditor()
StackExchange.prepareEditor(
heartbeatType: 'answer',
autoActivateHeartbeat: false,
convertImagesToLinks: false,
noModals: true,
showLowRepImageUploadWarning: true,
reputationToPostImages: null,
bindNavPrevention: true,
postfix: "",
imageUploader:
brandingHtml: "Powered by u003ca class="icon-imgur-white" href="https://imgur.com/"u003eu003c/au003e",
contentPolicyHtml: "User contributions licensed under u003ca href="https://creativecommons.org/licenses/by-sa/3.0/"u003ecc by-sa 3.0 with attribution requiredu003c/au003e u003ca href="https://stackoverflow.com/legal/content-policy"u003e(content policy)u003c/au003e",
allowUrls: true
,
onDemand: true,
discardSelector: ".discard-answer"
,immediatelyShowMarkdownHelp:true
);
);
Sign up or log in
StackExchange.ready(function ()
StackExchange.helpers.onClickDraftSave('#login-link');
);
Sign up using Google
Sign up using Facebook
Sign up using Email and Password
Post as a guest
Required, but never shown
StackExchange.ready(
function ()
StackExchange.openid.initPostLogin('.new-post-login', 'https%3a%2f%2fdatascience.stackexchange.com%2fquestions%2f48208%2fchoosing-the-first-node-in-a-decision-tree-basic-example%23new-answer', 'question_page');
);
Post as a guest
Required, but never shown
1 Answer
1
active
oldest
votes
1 Answer
1
active
oldest
votes
active
oldest
votes
active
oldest
votes
$begingroup$
Some corrections in the above:
- While calculating entropy of a split, you should take into account the weights - fraction of instances in each branch:
$$
entropy(A) = 0 * (2/6) + 0.81 * (4/6) = 0.54\
entropy(B) = 0 * (3/6) + 0.91 * (3/6) = 0.45\
entropy(C) = 0 * (2/6) + 0.81 * (4/6) = 0.54
$$
- While choosing an attribute to split, you should choose the one with lowest entropy after the split. Entropy is the uncertainty, so you want to minimise it. As an example, if the split was perfect (all 0's on one side, and all 1's on the other), then entropy will be 0.
After selection of one node, the tree is built out recursively, as you mention.
$endgroup$
add a comment |
$begingroup$
Some corrections in the above:
- While calculating entropy of a split, you should take into account the weights - fraction of instances in each branch:
$$
entropy(A) = 0 * (2/6) + 0.81 * (4/6) = 0.54\
entropy(B) = 0 * (3/6) + 0.91 * (3/6) = 0.45\
entropy(C) = 0 * (2/6) + 0.81 * (4/6) = 0.54
$$
- While choosing an attribute to split, you should choose the one with lowest entropy after the split. Entropy is the uncertainty, so you want to minimise it. As an example, if the split was perfect (all 0's on one side, and all 1's on the other), then entropy will be 0.
After selection of one node, the tree is built out recursively, as you mention.
$endgroup$
add a comment |
$begingroup$
Some corrections in the above:
- While calculating entropy of a split, you should take into account the weights - fraction of instances in each branch:
$$
entropy(A) = 0 * (2/6) + 0.81 * (4/6) = 0.54\
entropy(B) = 0 * (3/6) + 0.91 * (3/6) = 0.45\
entropy(C) = 0 * (2/6) + 0.81 * (4/6) = 0.54
$$
- While choosing an attribute to split, you should choose the one with lowest entropy after the split. Entropy is the uncertainty, so you want to minimise it. As an example, if the split was perfect (all 0's on one side, and all 1's on the other), then entropy will be 0.
After selection of one node, the tree is built out recursively, as you mention.
$endgroup$
Some corrections in the above:
- While calculating entropy of a split, you should take into account the weights - fraction of instances in each branch:
$$
entropy(A) = 0 * (2/6) + 0.81 * (4/6) = 0.54\
entropy(B) = 0 * (3/6) + 0.91 * (3/6) = 0.45\
entropy(C) = 0 * (2/6) + 0.81 * (4/6) = 0.54
$$
- While choosing an attribute to split, you should choose the one with lowest entropy after the split. Entropy is the uncertainty, so you want to minimise it. As an example, if the split was perfect (all 0's on one side, and all 1's on the other), then entropy will be 0.
After selection of one node, the tree is built out recursively, as you mention.
answered Mar 29 at 14:23
raghuraghu
45633
45633
add a comment |
add a comment |
Thanks for contributing an answer to Data Science Stack Exchange!
- Please be sure to answer the question. Provide details and share your research!
But avoid …
- Asking for help, clarification, or responding to other answers.
- Making statements based on opinion; back them up with references or personal experience.
Use MathJax to format equations. MathJax reference.
To learn more, see our tips on writing great answers.
Sign up or log in
StackExchange.ready(function ()
StackExchange.helpers.onClickDraftSave('#login-link');
);
Sign up using Google
Sign up using Facebook
Sign up using Email and Password
Post as a guest
Required, but never shown
StackExchange.ready(
function ()
StackExchange.openid.initPostLogin('.new-post-login', 'https%3a%2f%2fdatascience.stackexchange.com%2fquestions%2f48208%2fchoosing-the-first-node-in-a-decision-tree-basic-example%23new-answer', 'question_page');
);
Post as a guest
Required, but never shown
Sign up or log in
StackExchange.ready(function ()
StackExchange.helpers.onClickDraftSave('#login-link');
);
Sign up using Google
Sign up using Facebook
Sign up using Email and Password
Post as a guest
Required, but never shown
Sign up or log in
StackExchange.ready(function ()
StackExchange.helpers.onClickDraftSave('#login-link');
);
Sign up using Google
Sign up using Facebook
Sign up using Email and Password
Post as a guest
Required, but never shown
Sign up or log in
StackExchange.ready(function ()
StackExchange.helpers.onClickDraftSave('#login-link');
);
Sign up using Google
Sign up using Facebook
Sign up using Email and Password
Sign up using Google
Sign up using Facebook
Sign up using Email and Password
Post as a guest
Required, but never shown
Required, but never shown
Required, but never shown
Required, but never shown
Required, but never shown
Required, but never shown
Required, but never shown
Required, but never shown
Required, but never shown
aY9tfsXKbPe2httuX0sDwwTLoN8TFaimLM2YD4rGbN,3jR7j1hTtQoIvIVmfD7fd oLDx9cKX4ljqOEw,POBJs,9Ck