Training cycles Announcing the arrival of Valued Associate #679: Cesar Manara Planned maintenance scheduled April 17/18, 2019 at 00:00UTC (8:00pm US/Eastern) 2019 Moderator Election Q&A - Questionnaire 2019 Community Moderator Election ResultsWhy is learning rate causing my neural network's weights to skyrocket?Neural Network accuracy and loss guarantees?Convolution Neural Network Loss and performanceloss = function(iteration) gets super wobbly once it gets near the bottomNeural network only converges when data cloud is close to 0Why do we use gradients instead of residuals in Gradient Boosting?Deep learning with Tensorflow: training with big data setsDeep advantage learning: how to predict the valueMachine learning - 'train_test_split' function in scikit-learn: should I repeat it several times?CNN not learning properly
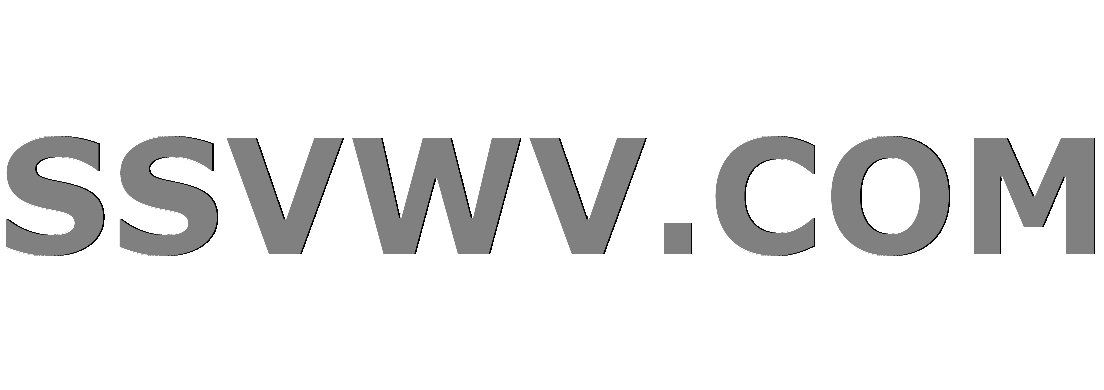
Multi tool use
Withdrew £2800, but only £2000 shows as withdrawn on online banking; what are my obligations?
How do I stop a creek from eroding my steep embankment?
Given a circle and line equations what are all possible solutions for k where the line sits tangent to the circle?
How to remove list items depending on predecessor in python
How to deal with a team lead who never gives me credit?
How do pianists reach extremely loud dynamics?
Why am I getting the error "non-boolean type specified in a context where a condition is expected" for this request?
A binary hook-length formula?
Where is the concept of Prapatti/Saranagati mentioned in the mukhya upanishads, as per the Sri Vaishnava interpretation?
Novel: non-telepath helps overthrow rule by telepaths
Why was the term "discrete" used in discrete logarithm?
String `!23` is replaced with `docker` in command line
Is it ethical to give a final exam after the professor has quit before teaching the remaining chapters of the course?
Seeking colloquialism for “just because”
How to react to hostile behavior from a senior developer?
Why are both D and D# fitting into my E minor key?
Why didn't Eitri join the fight?
How to compare two different files line by line in unix?
Why did the US and UK choose different solutions to the problem of an undemocratic upper house?
What causes the vertical darker bands in my photo?
What does the "x" in "x86" represent?
Fundamental Solution of the Pell Equation
Do square wave exist?
Check which numbers satisfy the condition [A*B*C = A! + B! + C!]
Training cycles
Announcing the arrival of Valued Associate #679: Cesar Manara
Planned maintenance scheduled April 17/18, 2019 at 00:00UTC (8:00pm US/Eastern)
2019 Moderator Election Q&A - Questionnaire
2019 Community Moderator Election ResultsWhy is learning rate causing my neural network's weights to skyrocket?Neural Network accuracy and loss guarantees?Convolution Neural Network Loss and performanceloss = function(iteration) gets super wobbly once it gets near the bottomNeural network only converges when data cloud is close to 0Why do we use gradients instead of residuals in Gradient Boosting?Deep learning with Tensorflow: training with big data setsDeep advantage learning: how to predict the valueMachine learning - 'train_test_split' function in scikit-learn: should I repeat it several times?CNN not learning properly
$begingroup$
In the context of Machine Learning, I encounter often the fact that a correction step does not occur after each training step, but only every n learning steps.
Citing from the Deep Learning with Python book:
This is the training loop, which, repeated a sufficient number of times (typically tens of iterations over thousands of examples), yields weight values that minimize the loss function
Why don't we correct at every step, but typically only once every 100 learning samples?
I assume, but I am not sure, that this might be because of efficiency, and also to smooth the correction "path" (e.g. integrating a correction step that is an average of the last 100 loss function values).
Thank you in advance!
machine-learning deep-learning
$endgroup$
add a comment |
$begingroup$
In the context of Machine Learning, I encounter often the fact that a correction step does not occur after each training step, but only every n learning steps.
Citing from the Deep Learning with Python book:
This is the training loop, which, repeated a sufficient number of times (typically tens of iterations over thousands of examples), yields weight values that minimize the loss function
Why don't we correct at every step, but typically only once every 100 learning samples?
I assume, but I am not sure, that this might be because of efficiency, and also to smooth the correction "path" (e.g. integrating a correction step that is an average of the last 100 loss function values).
Thank you in advance!
machine-learning deep-learning
$endgroup$
add a comment |
$begingroup$
In the context of Machine Learning, I encounter often the fact that a correction step does not occur after each training step, but only every n learning steps.
Citing from the Deep Learning with Python book:
This is the training loop, which, repeated a sufficient number of times (typically tens of iterations over thousands of examples), yields weight values that minimize the loss function
Why don't we correct at every step, but typically only once every 100 learning samples?
I assume, but I am not sure, that this might be because of efficiency, and also to smooth the correction "path" (e.g. integrating a correction step that is an average of the last 100 loss function values).
Thank you in advance!
machine-learning deep-learning
$endgroup$
In the context of Machine Learning, I encounter often the fact that a correction step does not occur after each training step, but only every n learning steps.
Citing from the Deep Learning with Python book:
This is the training loop, which, repeated a sufficient number of times (typically tens of iterations over thousands of examples), yields weight values that minimize the loss function
Why don't we correct at every step, but typically only once every 100 learning samples?
I assume, but I am not sure, that this might be because of efficiency, and also to smooth the correction "path" (e.g. integrating a correction step that is an average of the last 100 loss function values).
Thank you in advance!
machine-learning deep-learning
machine-learning deep-learning
asked Mar 4 at 10:22
SomeoneUnknownSomeoneUnknown
1
1
add a comment |
add a comment |
1 Answer
1
active
oldest
votes
$begingroup$
This is called batch updation which is the most popular method of updating weights. We also call it BatchSGD in context of SGD. Yes, what you mentioned is true. If we have 1000's of weights which we typically have in deep neural networks. It is not efficient to calculate partial derviatives at each weights for every input. Instead, We do the batch updation by which we will aggregate all the loss for last 100 inputs(as in your case) and at the end of 100th input, we take the average fo the losses and update the weight of the network. Keep in mind that calculation partial derivatives is one of the most compute intensive tasks that we perform in neural networks. So making 100 updates to Just 1 save a lot of compute. Hope it helps
$endgroup$
add a comment |
Your Answer
StackExchange.ready(function()
var channelOptions =
tags: "".split(" "),
id: "557"
;
initTagRenderer("".split(" "), "".split(" "), channelOptions);
StackExchange.using("externalEditor", function()
// Have to fire editor after snippets, if snippets enabled
if (StackExchange.settings.snippets.snippetsEnabled)
StackExchange.using("snippets", function()
createEditor();
);
else
createEditor();
);
function createEditor()
StackExchange.prepareEditor(
heartbeatType: 'answer',
autoActivateHeartbeat: false,
convertImagesToLinks: false,
noModals: true,
showLowRepImageUploadWarning: true,
reputationToPostImages: null,
bindNavPrevention: true,
postfix: "",
imageUploader:
brandingHtml: "Powered by u003ca class="icon-imgur-white" href="https://imgur.com/"u003eu003c/au003e",
contentPolicyHtml: "User contributions licensed under u003ca href="https://creativecommons.org/licenses/by-sa/3.0/"u003ecc by-sa 3.0 with attribution requiredu003c/au003e u003ca href="https://stackoverflow.com/legal/content-policy"u003e(content policy)u003c/au003e",
allowUrls: true
,
onDemand: true,
discardSelector: ".discard-answer"
,immediatelyShowMarkdownHelp:true
);
);
Sign up or log in
StackExchange.ready(function ()
StackExchange.helpers.onClickDraftSave('#login-link');
);
Sign up using Google
Sign up using Facebook
Sign up using Email and Password
Post as a guest
Required, but never shown
StackExchange.ready(
function ()
StackExchange.openid.initPostLogin('.new-post-login', 'https%3a%2f%2fdatascience.stackexchange.com%2fquestions%2f46630%2ftraining-cycles%23new-answer', 'question_page');
);
Post as a guest
Required, but never shown
1 Answer
1
active
oldest
votes
1 Answer
1
active
oldest
votes
active
oldest
votes
active
oldest
votes
$begingroup$
This is called batch updation which is the most popular method of updating weights. We also call it BatchSGD in context of SGD. Yes, what you mentioned is true. If we have 1000's of weights which we typically have in deep neural networks. It is not efficient to calculate partial derviatives at each weights for every input. Instead, We do the batch updation by which we will aggregate all the loss for last 100 inputs(as in your case) and at the end of 100th input, we take the average fo the losses and update the weight of the network. Keep in mind that calculation partial derivatives is one of the most compute intensive tasks that we perform in neural networks. So making 100 updates to Just 1 save a lot of compute. Hope it helps
$endgroup$
add a comment |
$begingroup$
This is called batch updation which is the most popular method of updating weights. We also call it BatchSGD in context of SGD. Yes, what you mentioned is true. If we have 1000's of weights which we typically have in deep neural networks. It is not efficient to calculate partial derviatives at each weights for every input. Instead, We do the batch updation by which we will aggregate all the loss for last 100 inputs(as in your case) and at the end of 100th input, we take the average fo the losses and update the weight of the network. Keep in mind that calculation partial derivatives is one of the most compute intensive tasks that we perform in neural networks. So making 100 updates to Just 1 save a lot of compute. Hope it helps
$endgroup$
add a comment |
$begingroup$
This is called batch updation which is the most popular method of updating weights. We also call it BatchSGD in context of SGD. Yes, what you mentioned is true. If we have 1000's of weights which we typically have in deep neural networks. It is not efficient to calculate partial derviatives at each weights for every input. Instead, We do the batch updation by which we will aggregate all the loss for last 100 inputs(as in your case) and at the end of 100th input, we take the average fo the losses and update the weight of the network. Keep in mind that calculation partial derivatives is one of the most compute intensive tasks that we perform in neural networks. So making 100 updates to Just 1 save a lot of compute. Hope it helps
$endgroup$
This is called batch updation which is the most popular method of updating weights. We also call it BatchSGD in context of SGD. Yes, what you mentioned is true. If we have 1000's of weights which we typically have in deep neural networks. It is not efficient to calculate partial derviatives at each weights for every input. Instead, We do the batch updation by which we will aggregate all the loss for last 100 inputs(as in your case) and at the end of 100th input, we take the average fo the losses and update the weight of the network. Keep in mind that calculation partial derivatives is one of the most compute intensive tasks that we perform in neural networks. So making 100 updates to Just 1 save a lot of compute. Hope it helps
answered Mar 4 at 11:56


karthikeyan mgkarthikeyan mg
305111
305111
add a comment |
add a comment |
Thanks for contributing an answer to Data Science Stack Exchange!
- Please be sure to answer the question. Provide details and share your research!
But avoid …
- Asking for help, clarification, or responding to other answers.
- Making statements based on opinion; back them up with references or personal experience.
Use MathJax to format equations. MathJax reference.
To learn more, see our tips on writing great answers.
Sign up or log in
StackExchange.ready(function ()
StackExchange.helpers.onClickDraftSave('#login-link');
);
Sign up using Google
Sign up using Facebook
Sign up using Email and Password
Post as a guest
Required, but never shown
StackExchange.ready(
function ()
StackExchange.openid.initPostLogin('.new-post-login', 'https%3a%2f%2fdatascience.stackexchange.com%2fquestions%2f46630%2ftraining-cycles%23new-answer', 'question_page');
);
Post as a guest
Required, but never shown
Sign up or log in
StackExchange.ready(function ()
StackExchange.helpers.onClickDraftSave('#login-link');
);
Sign up using Google
Sign up using Facebook
Sign up using Email and Password
Post as a guest
Required, but never shown
Sign up or log in
StackExchange.ready(function ()
StackExchange.helpers.onClickDraftSave('#login-link');
);
Sign up using Google
Sign up using Facebook
Sign up using Email and Password
Post as a guest
Required, but never shown
Sign up or log in
StackExchange.ready(function ()
StackExchange.helpers.onClickDraftSave('#login-link');
);
Sign up using Google
Sign up using Facebook
Sign up using Email and Password
Sign up using Google
Sign up using Facebook
Sign up using Email and Password
Post as a guest
Required, but never shown
Required, but never shown
Required, but never shown
Required, but never shown
Required, but never shown
Required, but never shown
Required, but never shown
Required, but never shown
Required, but never shown
72QmSE,ourYrdaGziWEwa7tTIs,x9kLyF,OmrFokwE3 hpwADHev0 XDgFPs,32eMIa1C 98EWl6