RL Advantage function why A = Q-V instead of A=V-Q?2019 Community Moderator ElectionDueling DQN - can't understand its mechanismWhat is the Q function and what is the V function in reinforcement learning?Understanding advantage functionsWhat is the difference between “expected return” and “expected reward” in the context of RL?Q-learning why do we subtract the Q(s, a) term during update?RL Policy Gradient: How to deal with rewards that are strictly positive?Why are policy gradients on-policy?Why is Distributional DQN faster than vanilla DQN?Why not use max(returns) instead of average(returns) in off-policy Monte Carlo control?Reinforcement learning for continuous state and action spaceEvaluating value functions in RL
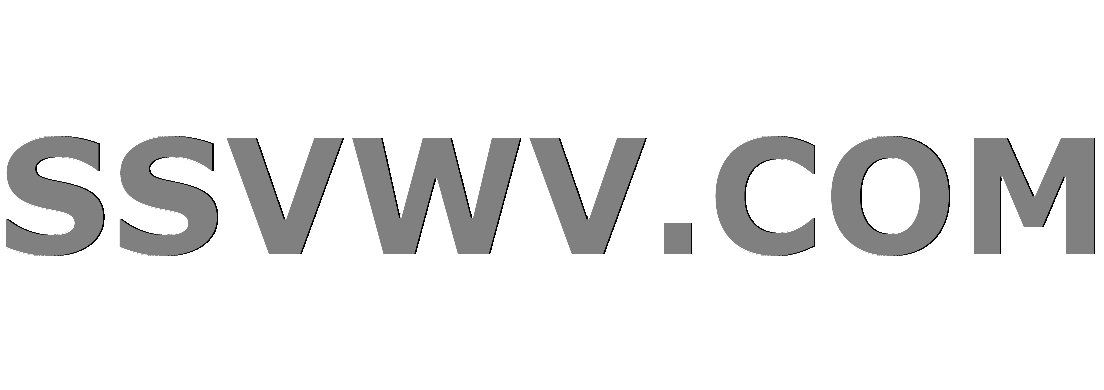
Multi tool use
How to safely derail a train during transit?
What happens if you roll doubles 3 times then land on "Go to jail?"
How to pronounce the slash sign
Lay out the Carpet
What is the intuitive meaning of having a linear relationship between the logs of two variables?
Customer Requests (Sometimes) Drive Me Bonkers!
What does "I’d sit this one out, Cap," imply or mean in the context?
Short story about space worker geeks who zone out by 'listening' to radiation from stars
How easy is it to start Magic from scratch?
Under what conditions does the function C = f(A,B) satisfy H(C|A) = H(B)?
Do sorcerers' Subtle Spells require a skill check to be unseen?
Opposite of a diet
Why didn't Theresa May consult with Parliament before negotiating a deal with the EU?
Tiptoe or tiphoof? Adjusting words to better fit fantasy races
Describing a person. What needs to be mentioned?
Avoiding estate tax by giving multiple gifts
Is HostGator storing my password in plaintext?
Number of words that can be made using all the letters of the word W, if Os as well as Is are separated is?
India just shot down a satellite from the ground. At what altitude range is the resulting debris field?
What grammatical function is や performing here?
How did Doctor Strange see the winning outcome in Avengers: Infinity War?
How do I go from 300 unfinished/half written blog posts, to published posts?
Large drywall patch supports
How to be diplomatic in refusing to write code that breaches the privacy of our users
RL Advantage function why A = Q-V instead of A=V-Q?
2019 Community Moderator ElectionDueling DQN - can't understand its mechanismWhat is the Q function and what is the V function in reinforcement learning?Understanding advantage functionsWhat is the difference between “expected return” and “expected reward” in the context of RL?Q-learning why do we subtract the Q(s, a) term during update?RL Policy Gradient: How to deal with rewards that are strictly positive?Why are policy gradients on-policy?Why is Distributional DQN faster than vanilla DQN?Why not use max(returns) instead of average(returns) in off-policy Monte Carlo control?Reinforcement learning for continuous state and action spaceEvaluating value functions in RL
$begingroup$
In RL Course by David Silver - Lecture 7: Policy Gradient Methods, David explains what an Advantage function is, and how it's the difference between Q(s,a) and the V(s)
Preliminary, from this post:
First recall that a policy $pi$ is a mapping from each state, $s$, action $a$, to the probability $pi(a mid s)$ of taking action $a$ when in state $s$.
The state value function, $V^pi(s)$, is the expected return when starting in state $s$ and following $pi$ thereafter.
Similarly, the state-action value function, $Q^pi(s, a)$, is the expected return of when starting in state $s$, taking action $a$, and following policy $pi$ thereafter.
In my understanding, $V(s)$ is always larger than $Q(s, a)$, because the function $V$ includes the reward for the current state $s$, unlike $Q$. So, why is the advantage function defined as $A = V - Q$ rather than $A = Q - V$ (at minute 1:12:29 in the video)?
Actually, V might not be larger than Q, because $s$ might actually contain a negative reward. In such a case how can we be certain what to subtract from what, such that our Advantage is always positive?
$Q(s, a)$ returns a value of entire total reward that's expected ultimately, after we pick an action $a$. $V(s)$ is the same, just with an extra reward from current state $s$ as well.
I don't see why a value of $Q - V$ would be useful. On the other hand, $V - Q$ would be useful because it would tell us the reward we would get on $s_t+1$ if we took the action $a$.
reinforcement-learning q-learning variance
$endgroup$
add a comment |
$begingroup$
In RL Course by David Silver - Lecture 7: Policy Gradient Methods, David explains what an Advantage function is, and how it's the difference between Q(s,a) and the V(s)
Preliminary, from this post:
First recall that a policy $pi$ is a mapping from each state, $s$, action $a$, to the probability $pi(a mid s)$ of taking action $a$ when in state $s$.
The state value function, $V^pi(s)$, is the expected return when starting in state $s$ and following $pi$ thereafter.
Similarly, the state-action value function, $Q^pi(s, a)$, is the expected return of when starting in state $s$, taking action $a$, and following policy $pi$ thereafter.
In my understanding, $V(s)$ is always larger than $Q(s, a)$, because the function $V$ includes the reward for the current state $s$, unlike $Q$. So, why is the advantage function defined as $A = V - Q$ rather than $A = Q - V$ (at minute 1:12:29 in the video)?
Actually, V might not be larger than Q, because $s$ might actually contain a negative reward. In such a case how can we be certain what to subtract from what, such that our Advantage is always positive?
$Q(s, a)$ returns a value of entire total reward that's expected ultimately, after we pick an action $a$. $V(s)$ is the same, just with an extra reward from current state $s$ as well.
I don't see why a value of $Q - V$ would be useful. On the other hand, $V - Q$ would be useful because it would tell us the reward we would get on $s_t+1$ if we took the action $a$.
reinforcement-learning q-learning variance
$endgroup$
add a comment |
$begingroup$
In RL Course by David Silver - Lecture 7: Policy Gradient Methods, David explains what an Advantage function is, and how it's the difference between Q(s,a) and the V(s)
Preliminary, from this post:
First recall that a policy $pi$ is a mapping from each state, $s$, action $a$, to the probability $pi(a mid s)$ of taking action $a$ when in state $s$.
The state value function, $V^pi(s)$, is the expected return when starting in state $s$ and following $pi$ thereafter.
Similarly, the state-action value function, $Q^pi(s, a)$, is the expected return of when starting in state $s$, taking action $a$, and following policy $pi$ thereafter.
In my understanding, $V(s)$ is always larger than $Q(s, a)$, because the function $V$ includes the reward for the current state $s$, unlike $Q$. So, why is the advantage function defined as $A = V - Q$ rather than $A = Q - V$ (at minute 1:12:29 in the video)?
Actually, V might not be larger than Q, because $s$ might actually contain a negative reward. In such a case how can we be certain what to subtract from what, such that our Advantage is always positive?
$Q(s, a)$ returns a value of entire total reward that's expected ultimately, after we pick an action $a$. $V(s)$ is the same, just with an extra reward from current state $s$ as well.
I don't see why a value of $Q - V$ would be useful. On the other hand, $V - Q$ would be useful because it would tell us the reward we would get on $s_t+1$ if we took the action $a$.
reinforcement-learning q-learning variance
$endgroup$
In RL Course by David Silver - Lecture 7: Policy Gradient Methods, David explains what an Advantage function is, and how it's the difference between Q(s,a) and the V(s)
Preliminary, from this post:
First recall that a policy $pi$ is a mapping from each state, $s$, action $a$, to the probability $pi(a mid s)$ of taking action $a$ when in state $s$.
The state value function, $V^pi(s)$, is the expected return when starting in state $s$ and following $pi$ thereafter.
Similarly, the state-action value function, $Q^pi(s, a)$, is the expected return of when starting in state $s$, taking action $a$, and following policy $pi$ thereafter.
In my understanding, $V(s)$ is always larger than $Q(s, a)$, because the function $V$ includes the reward for the current state $s$, unlike $Q$. So, why is the advantage function defined as $A = V - Q$ rather than $A = Q - V$ (at minute 1:12:29 in the video)?
Actually, V might not be larger than Q, because $s$ might actually contain a negative reward. In such a case how can we be certain what to subtract from what, such that our Advantage is always positive?
$Q(s, a)$ returns a value of entire total reward that's expected ultimately, after we pick an action $a$. $V(s)$ is the same, just with an extra reward from current state $s$ as well.
I don't see why a value of $Q - V$ would be useful. On the other hand, $V - Q$ would be useful because it would tell us the reward we would get on $s_t+1$ if we took the action $a$.
reinforcement-learning q-learning variance
reinforcement-learning q-learning variance
edited Mar 22 at 20:28
Kari
asked Sep 1 '18 at 3:08


KariKari
614423
614423
add a comment |
add a comment |
1 Answer
1
active
oldest
votes
$begingroup$
In my understanding, $V(s)$ is always larger than $Q(s,a)$, because the function $V$ includes the reward for the current state $s$, unlike $Q$
This is incorrect. There is not really such a thing as "the reward for current state" in the general case of a MDP. If you mean the $V(S_t)$ should include the value of $R_t$, then this is still wrong, given David Silver's use of the conventions for time step indexing. It is possible to associate immediate reward with either the current time step, leading to sequence $S_0, A_0, R_0, S_1, A_1, R_1$ etc or you can use the convention of immediate reward being on next time step $S_0, A_0, R_1, S_1, A_1, R_2$ etc. David Silver (and Sutton & Barto's book) uses the latter convention.
Under that convention:
$$V(s) = mathbbE_pi[sum_k=0^infty
gamma^kR_t+k+1|S_t=s]$$
$$Q(s,a) = mathbbE_pi[sum_k=0^infty gamma^kR_t+k+1|S_t=s, A_t=a]$$
You can see that the first term in the expansion of the sum for both Q(s,a) and V(s) is $R_t+1$. If you changed the convention, then both would include the equivalent value, but would be labelled $R_t$ in any formula.
Q and V do not differ in which time steps they sum reward over. They may differ in the value of $R_t+1$ because $V(s)$ assumes following the policy $pi$ when selecting $A_t$ whilst $Q(s,a)$ uses the value $a$ supplied as a parameter for $A_t$, which can be different.
how can we be certain what to subtract from what, such that our Advantage is always positive?
Advantage can be negative, that is fine. It means that the action $a$ in $A(s,a)$ is a worse choice than the current policy's.
I don't see why a value of $(Q−V)$ would be useful. On the other hand, $(V−Q)$ would be useful
Both would be equally useful, it is mainly convention that we work with finding maximum Advantage representing the benefit of choosing a specific action instead of following the current policy, as opposed to finding the minimum "Disadvantage". However, the concept of Advantage in this context is arguably the more natural view.
because it would tell us the reward we would get on $s_t+1$ if we took the action $a$
As explained above, this is wrong. The value in $A(s,a)$ expresses the potential benefit we would get for changing the policy $pi(s)$. That might include changes to $R_t+1$, but is not limited to a single time step.
Some RL approaches do create a predictive function for expected immediate reward $hatr(s,a)$ - typically this is a secondary component, used to help refine parameters for other function approximators.
$endgroup$
1
$begingroup$
Got it! So my intuition is correct, with one adjustment. As you've explained, V(s) and Q(s,a) include rewards from the next timesteps, onwards. It's just that V(s) promises that we will act according to the policy, even the current timestep, while Q(s,a) allows to select the action a once, right now, and then will follow the policy
$endgroup$
– Kari
Sep 2 '18 at 13:35
$begingroup$
Taking such an action $a$ might mean violating the policy once, but allows us to see if such a violation resulted in better total reward (might have placed us on a completely different train of states, with larger rewards). And a difference with $V(s)$ in other words "with total remaining rewards were we to follow that old policy", is exactly the advantage. So $Q - V$ indeed makes sense. Thanks!!
$endgroup$
– Kari
Sep 2 '18 at 13:35
1
$begingroup$
@Kari: Yes, that's it.
$endgroup$
– Neil Slater
Sep 2 '18 at 17:47
add a comment |
Your Answer
StackExchange.ifUsing("editor", function ()
return StackExchange.using("mathjaxEditing", function ()
StackExchange.MarkdownEditor.creationCallbacks.add(function (editor, postfix)
StackExchange.mathjaxEditing.prepareWmdForMathJax(editor, postfix, [["$", "$"], ["\\(","\\)"]]);
);
);
, "mathjax-editing");
StackExchange.ready(function()
var channelOptions =
tags: "".split(" "),
id: "557"
;
initTagRenderer("".split(" "), "".split(" "), channelOptions);
StackExchange.using("externalEditor", function()
// Have to fire editor after snippets, if snippets enabled
if (StackExchange.settings.snippets.snippetsEnabled)
StackExchange.using("snippets", function()
createEditor();
);
else
createEditor();
);
function createEditor()
StackExchange.prepareEditor(
heartbeatType: 'answer',
autoActivateHeartbeat: false,
convertImagesToLinks: false,
noModals: true,
showLowRepImageUploadWarning: true,
reputationToPostImages: null,
bindNavPrevention: true,
postfix: "",
imageUploader:
brandingHtml: "Powered by u003ca class="icon-imgur-white" href="https://imgur.com/"u003eu003c/au003e",
contentPolicyHtml: "User contributions licensed under u003ca href="https://creativecommons.org/licenses/by-sa/3.0/"u003ecc by-sa 3.0 with attribution requiredu003c/au003e u003ca href="https://stackoverflow.com/legal/content-policy"u003e(content policy)u003c/au003e",
allowUrls: true
,
onDemand: true,
discardSelector: ".discard-answer"
,immediatelyShowMarkdownHelp:true
);
);
Sign up or log in
StackExchange.ready(function ()
StackExchange.helpers.onClickDraftSave('#login-link');
);
Sign up using Google
Sign up using Facebook
Sign up using Email and Password
Post as a guest
Required, but never shown
StackExchange.ready(
function ()
StackExchange.openid.initPostLogin('.new-post-login', 'https%3a%2f%2fdatascience.stackexchange.com%2fquestions%2f37673%2frl-advantage-function-why-a-q-v-instead-of-a-v-q%23new-answer', 'question_page');
);
Post as a guest
Required, but never shown
1 Answer
1
active
oldest
votes
1 Answer
1
active
oldest
votes
active
oldest
votes
active
oldest
votes
$begingroup$
In my understanding, $V(s)$ is always larger than $Q(s,a)$, because the function $V$ includes the reward for the current state $s$, unlike $Q$
This is incorrect. There is not really such a thing as "the reward for current state" in the general case of a MDP. If you mean the $V(S_t)$ should include the value of $R_t$, then this is still wrong, given David Silver's use of the conventions for time step indexing. It is possible to associate immediate reward with either the current time step, leading to sequence $S_0, A_0, R_0, S_1, A_1, R_1$ etc or you can use the convention of immediate reward being on next time step $S_0, A_0, R_1, S_1, A_1, R_2$ etc. David Silver (and Sutton & Barto's book) uses the latter convention.
Under that convention:
$$V(s) = mathbbE_pi[sum_k=0^infty
gamma^kR_t+k+1|S_t=s]$$
$$Q(s,a) = mathbbE_pi[sum_k=0^infty gamma^kR_t+k+1|S_t=s, A_t=a]$$
You can see that the first term in the expansion of the sum for both Q(s,a) and V(s) is $R_t+1$. If you changed the convention, then both would include the equivalent value, but would be labelled $R_t$ in any formula.
Q and V do not differ in which time steps they sum reward over. They may differ in the value of $R_t+1$ because $V(s)$ assumes following the policy $pi$ when selecting $A_t$ whilst $Q(s,a)$ uses the value $a$ supplied as a parameter for $A_t$, which can be different.
how can we be certain what to subtract from what, such that our Advantage is always positive?
Advantage can be negative, that is fine. It means that the action $a$ in $A(s,a)$ is a worse choice than the current policy's.
I don't see why a value of $(Q−V)$ would be useful. On the other hand, $(V−Q)$ would be useful
Both would be equally useful, it is mainly convention that we work with finding maximum Advantage representing the benefit of choosing a specific action instead of following the current policy, as opposed to finding the minimum "Disadvantage". However, the concept of Advantage in this context is arguably the more natural view.
because it would tell us the reward we would get on $s_t+1$ if we took the action $a$
As explained above, this is wrong. The value in $A(s,a)$ expresses the potential benefit we would get for changing the policy $pi(s)$. That might include changes to $R_t+1$, but is not limited to a single time step.
Some RL approaches do create a predictive function for expected immediate reward $hatr(s,a)$ - typically this is a secondary component, used to help refine parameters for other function approximators.
$endgroup$
1
$begingroup$
Got it! So my intuition is correct, with one adjustment. As you've explained, V(s) and Q(s,a) include rewards from the next timesteps, onwards. It's just that V(s) promises that we will act according to the policy, even the current timestep, while Q(s,a) allows to select the action a once, right now, and then will follow the policy
$endgroup$
– Kari
Sep 2 '18 at 13:35
$begingroup$
Taking such an action $a$ might mean violating the policy once, but allows us to see if such a violation resulted in better total reward (might have placed us on a completely different train of states, with larger rewards). And a difference with $V(s)$ in other words "with total remaining rewards were we to follow that old policy", is exactly the advantage. So $Q - V$ indeed makes sense. Thanks!!
$endgroup$
– Kari
Sep 2 '18 at 13:35
1
$begingroup$
@Kari: Yes, that's it.
$endgroup$
– Neil Slater
Sep 2 '18 at 17:47
add a comment |
$begingroup$
In my understanding, $V(s)$ is always larger than $Q(s,a)$, because the function $V$ includes the reward for the current state $s$, unlike $Q$
This is incorrect. There is not really such a thing as "the reward for current state" in the general case of a MDP. If you mean the $V(S_t)$ should include the value of $R_t$, then this is still wrong, given David Silver's use of the conventions for time step indexing. It is possible to associate immediate reward with either the current time step, leading to sequence $S_0, A_0, R_0, S_1, A_1, R_1$ etc or you can use the convention of immediate reward being on next time step $S_0, A_0, R_1, S_1, A_1, R_2$ etc. David Silver (and Sutton & Barto's book) uses the latter convention.
Under that convention:
$$V(s) = mathbbE_pi[sum_k=0^infty
gamma^kR_t+k+1|S_t=s]$$
$$Q(s,a) = mathbbE_pi[sum_k=0^infty gamma^kR_t+k+1|S_t=s, A_t=a]$$
You can see that the first term in the expansion of the sum for both Q(s,a) and V(s) is $R_t+1$. If you changed the convention, then both would include the equivalent value, but would be labelled $R_t$ in any formula.
Q and V do not differ in which time steps they sum reward over. They may differ in the value of $R_t+1$ because $V(s)$ assumes following the policy $pi$ when selecting $A_t$ whilst $Q(s,a)$ uses the value $a$ supplied as a parameter for $A_t$, which can be different.
how can we be certain what to subtract from what, such that our Advantage is always positive?
Advantage can be negative, that is fine. It means that the action $a$ in $A(s,a)$ is a worse choice than the current policy's.
I don't see why a value of $(Q−V)$ would be useful. On the other hand, $(V−Q)$ would be useful
Both would be equally useful, it is mainly convention that we work with finding maximum Advantage representing the benefit of choosing a specific action instead of following the current policy, as opposed to finding the minimum "Disadvantage". However, the concept of Advantage in this context is arguably the more natural view.
because it would tell us the reward we would get on $s_t+1$ if we took the action $a$
As explained above, this is wrong. The value in $A(s,a)$ expresses the potential benefit we would get for changing the policy $pi(s)$. That might include changes to $R_t+1$, but is not limited to a single time step.
Some RL approaches do create a predictive function for expected immediate reward $hatr(s,a)$ - typically this is a secondary component, used to help refine parameters for other function approximators.
$endgroup$
1
$begingroup$
Got it! So my intuition is correct, with one adjustment. As you've explained, V(s) and Q(s,a) include rewards from the next timesteps, onwards. It's just that V(s) promises that we will act according to the policy, even the current timestep, while Q(s,a) allows to select the action a once, right now, and then will follow the policy
$endgroup$
– Kari
Sep 2 '18 at 13:35
$begingroup$
Taking such an action $a$ might mean violating the policy once, but allows us to see if such a violation resulted in better total reward (might have placed us on a completely different train of states, with larger rewards). And a difference with $V(s)$ in other words "with total remaining rewards were we to follow that old policy", is exactly the advantage. So $Q - V$ indeed makes sense. Thanks!!
$endgroup$
– Kari
Sep 2 '18 at 13:35
1
$begingroup$
@Kari: Yes, that's it.
$endgroup$
– Neil Slater
Sep 2 '18 at 17:47
add a comment |
$begingroup$
In my understanding, $V(s)$ is always larger than $Q(s,a)$, because the function $V$ includes the reward for the current state $s$, unlike $Q$
This is incorrect. There is not really such a thing as "the reward for current state" in the general case of a MDP. If you mean the $V(S_t)$ should include the value of $R_t$, then this is still wrong, given David Silver's use of the conventions for time step indexing. It is possible to associate immediate reward with either the current time step, leading to sequence $S_0, A_0, R_0, S_1, A_1, R_1$ etc or you can use the convention of immediate reward being on next time step $S_0, A_0, R_1, S_1, A_1, R_2$ etc. David Silver (and Sutton & Barto's book) uses the latter convention.
Under that convention:
$$V(s) = mathbbE_pi[sum_k=0^infty
gamma^kR_t+k+1|S_t=s]$$
$$Q(s,a) = mathbbE_pi[sum_k=0^infty gamma^kR_t+k+1|S_t=s, A_t=a]$$
You can see that the first term in the expansion of the sum for both Q(s,a) and V(s) is $R_t+1$. If you changed the convention, then both would include the equivalent value, but would be labelled $R_t$ in any formula.
Q and V do not differ in which time steps they sum reward over. They may differ in the value of $R_t+1$ because $V(s)$ assumes following the policy $pi$ when selecting $A_t$ whilst $Q(s,a)$ uses the value $a$ supplied as a parameter for $A_t$, which can be different.
how can we be certain what to subtract from what, such that our Advantage is always positive?
Advantage can be negative, that is fine. It means that the action $a$ in $A(s,a)$ is a worse choice than the current policy's.
I don't see why a value of $(Q−V)$ would be useful. On the other hand, $(V−Q)$ would be useful
Both would be equally useful, it is mainly convention that we work with finding maximum Advantage representing the benefit of choosing a specific action instead of following the current policy, as opposed to finding the minimum "Disadvantage". However, the concept of Advantage in this context is arguably the more natural view.
because it would tell us the reward we would get on $s_t+1$ if we took the action $a$
As explained above, this is wrong. The value in $A(s,a)$ expresses the potential benefit we would get for changing the policy $pi(s)$. That might include changes to $R_t+1$, but is not limited to a single time step.
Some RL approaches do create a predictive function for expected immediate reward $hatr(s,a)$ - typically this is a secondary component, used to help refine parameters for other function approximators.
$endgroup$
In my understanding, $V(s)$ is always larger than $Q(s,a)$, because the function $V$ includes the reward for the current state $s$, unlike $Q$
This is incorrect. There is not really such a thing as "the reward for current state" in the general case of a MDP. If you mean the $V(S_t)$ should include the value of $R_t$, then this is still wrong, given David Silver's use of the conventions for time step indexing. It is possible to associate immediate reward with either the current time step, leading to sequence $S_0, A_0, R_0, S_1, A_1, R_1$ etc or you can use the convention of immediate reward being on next time step $S_0, A_0, R_1, S_1, A_1, R_2$ etc. David Silver (and Sutton & Barto's book) uses the latter convention.
Under that convention:
$$V(s) = mathbbE_pi[sum_k=0^infty
gamma^kR_t+k+1|S_t=s]$$
$$Q(s,a) = mathbbE_pi[sum_k=0^infty gamma^kR_t+k+1|S_t=s, A_t=a]$$
You can see that the first term in the expansion of the sum for both Q(s,a) and V(s) is $R_t+1$. If you changed the convention, then both would include the equivalent value, but would be labelled $R_t$ in any formula.
Q and V do not differ in which time steps they sum reward over. They may differ in the value of $R_t+1$ because $V(s)$ assumes following the policy $pi$ when selecting $A_t$ whilst $Q(s,a)$ uses the value $a$ supplied as a parameter for $A_t$, which can be different.
how can we be certain what to subtract from what, such that our Advantage is always positive?
Advantage can be negative, that is fine. It means that the action $a$ in $A(s,a)$ is a worse choice than the current policy's.
I don't see why a value of $(Q−V)$ would be useful. On the other hand, $(V−Q)$ would be useful
Both would be equally useful, it is mainly convention that we work with finding maximum Advantage representing the benefit of choosing a specific action instead of following the current policy, as opposed to finding the minimum "Disadvantage". However, the concept of Advantage in this context is arguably the more natural view.
because it would tell us the reward we would get on $s_t+1$ if we took the action $a$
As explained above, this is wrong. The value in $A(s,a)$ expresses the potential benefit we would get for changing the policy $pi(s)$. That might include changes to $R_t+1$, but is not limited to a single time step.
Some RL approaches do create a predictive function for expected immediate reward $hatr(s,a)$ - typically this is a secondary component, used to help refine parameters for other function approximators.
edited Sep 1 '18 at 10:49
answered Sep 1 '18 at 8:40
Neil SlaterNeil Slater
17.4k33063
17.4k33063
1
$begingroup$
Got it! So my intuition is correct, with one adjustment. As you've explained, V(s) and Q(s,a) include rewards from the next timesteps, onwards. It's just that V(s) promises that we will act according to the policy, even the current timestep, while Q(s,a) allows to select the action a once, right now, and then will follow the policy
$endgroup$
– Kari
Sep 2 '18 at 13:35
$begingroup$
Taking such an action $a$ might mean violating the policy once, but allows us to see if such a violation resulted in better total reward (might have placed us on a completely different train of states, with larger rewards). And a difference with $V(s)$ in other words "with total remaining rewards were we to follow that old policy", is exactly the advantage. So $Q - V$ indeed makes sense. Thanks!!
$endgroup$
– Kari
Sep 2 '18 at 13:35
1
$begingroup$
@Kari: Yes, that's it.
$endgroup$
– Neil Slater
Sep 2 '18 at 17:47
add a comment |
1
$begingroup$
Got it! So my intuition is correct, with one adjustment. As you've explained, V(s) and Q(s,a) include rewards from the next timesteps, onwards. It's just that V(s) promises that we will act according to the policy, even the current timestep, while Q(s,a) allows to select the action a once, right now, and then will follow the policy
$endgroup$
– Kari
Sep 2 '18 at 13:35
$begingroup$
Taking such an action $a$ might mean violating the policy once, but allows us to see if such a violation resulted in better total reward (might have placed us on a completely different train of states, with larger rewards). And a difference with $V(s)$ in other words "with total remaining rewards were we to follow that old policy", is exactly the advantage. So $Q - V$ indeed makes sense. Thanks!!
$endgroup$
– Kari
Sep 2 '18 at 13:35
1
$begingroup$
@Kari: Yes, that's it.
$endgroup$
– Neil Slater
Sep 2 '18 at 17:47
1
1
$begingroup$
Got it! So my intuition is correct, with one adjustment. As you've explained, V(s) and Q(s,a) include rewards from the next timesteps, onwards. It's just that V(s) promises that we will act according to the policy, even the current timestep, while Q(s,a) allows to select the action a once, right now, and then will follow the policy
$endgroup$
– Kari
Sep 2 '18 at 13:35
$begingroup$
Got it! So my intuition is correct, with one adjustment. As you've explained, V(s) and Q(s,a) include rewards from the next timesteps, onwards. It's just that V(s) promises that we will act according to the policy, even the current timestep, while Q(s,a) allows to select the action a once, right now, and then will follow the policy
$endgroup$
– Kari
Sep 2 '18 at 13:35
$begingroup$
Taking such an action $a$ might mean violating the policy once, but allows us to see if such a violation resulted in better total reward (might have placed us on a completely different train of states, with larger rewards). And a difference with $V(s)$ in other words "with total remaining rewards were we to follow that old policy", is exactly the advantage. So $Q - V$ indeed makes sense. Thanks!!
$endgroup$
– Kari
Sep 2 '18 at 13:35
$begingroup$
Taking such an action $a$ might mean violating the policy once, but allows us to see if such a violation resulted in better total reward (might have placed us on a completely different train of states, with larger rewards). And a difference with $V(s)$ in other words "with total remaining rewards were we to follow that old policy", is exactly the advantage. So $Q - V$ indeed makes sense. Thanks!!
$endgroup$
– Kari
Sep 2 '18 at 13:35
1
1
$begingroup$
@Kari: Yes, that's it.
$endgroup$
– Neil Slater
Sep 2 '18 at 17:47
$begingroup$
@Kari: Yes, that's it.
$endgroup$
– Neil Slater
Sep 2 '18 at 17:47
add a comment |
Thanks for contributing an answer to Data Science Stack Exchange!
- Please be sure to answer the question. Provide details and share your research!
But avoid …
- Asking for help, clarification, or responding to other answers.
- Making statements based on opinion; back them up with references or personal experience.
Use MathJax to format equations. MathJax reference.
To learn more, see our tips on writing great answers.
Sign up or log in
StackExchange.ready(function ()
StackExchange.helpers.onClickDraftSave('#login-link');
);
Sign up using Google
Sign up using Facebook
Sign up using Email and Password
Post as a guest
Required, but never shown
StackExchange.ready(
function ()
StackExchange.openid.initPostLogin('.new-post-login', 'https%3a%2f%2fdatascience.stackexchange.com%2fquestions%2f37673%2frl-advantage-function-why-a-q-v-instead-of-a-v-q%23new-answer', 'question_page');
);
Post as a guest
Required, but never shown
Sign up or log in
StackExchange.ready(function ()
StackExchange.helpers.onClickDraftSave('#login-link');
);
Sign up using Google
Sign up using Facebook
Sign up using Email and Password
Post as a guest
Required, but never shown
Sign up or log in
StackExchange.ready(function ()
StackExchange.helpers.onClickDraftSave('#login-link');
);
Sign up using Google
Sign up using Facebook
Sign up using Email and Password
Post as a guest
Required, but never shown
Sign up or log in
StackExchange.ready(function ()
StackExchange.helpers.onClickDraftSave('#login-link');
);
Sign up using Google
Sign up using Facebook
Sign up using Email and Password
Sign up using Google
Sign up using Facebook
Sign up using Email and Password
Post as a guest
Required, but never shown
Required, but never shown
Required, but never shown
Required, but never shown
Required, but never shown
Required, but never shown
Required, but never shown
Required, but never shown
Required, but never shown
Jq,w4aEHv vZLgSSwLWPSXmb,7 QNQ7J1ddSDM0XXMhvhz6,UC9k8I,rR24Quonqvvkw0EBssKIYhMy2I,i78V