Decovolution function Announcing the arrival of Valued Associate #679: Cesar Manara Planned maintenance scheduled April 23, 2019 at 00:00UTC (8:00pm US/Eastern) 2019 Moderator Election Q&A - Questionnaire 2019 Community Moderator Election ResultsDeconvolutional Network in Semantic SegmentationPrepending Input layer to pre-trained modelUsing deconvolution in practiceIn a convolutional neural network (CNN), when convolving the image, is the operation used the dot product or the sum of element-wise multiplication?I still don't know how deconvolution works after watching CS231 lecture, I need helpDeep learning with Tensorflow: training with big data setsAppending to numpy array for creating datasetTranspose convolution math not working outCalculating sin function with neural network using python
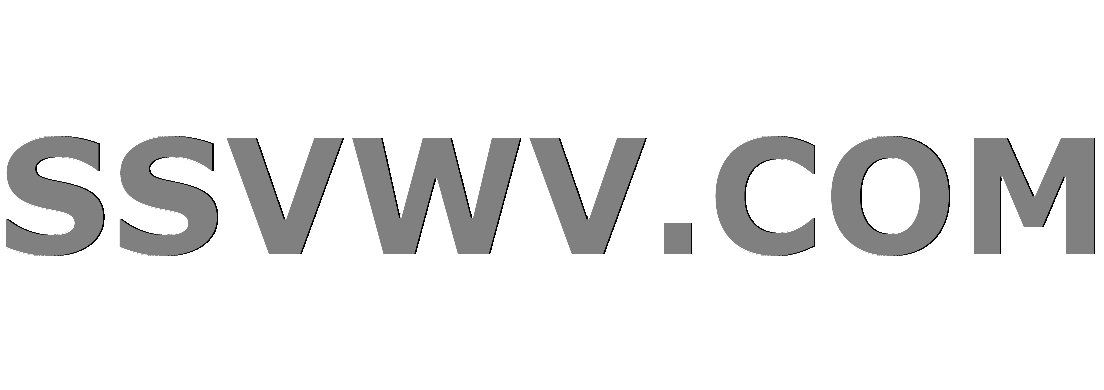
Multi tool use
Is there hard evidence that the grant peer review system performs significantly better than random?
One-one communication
Random body shuffle every night—can we still function?
How does light 'choose' between wave and particle behaviour?
How could we fake a moon landing now?
What is an "asse" in Elizabethan English?
Is it possible to force a specific program to remain in memory after closing it?
Why does it sometimes sound good to play a grace note as a lead in to a note in a melody?
An adverb for when you're not exaggerating
How were pictures turned from film to a big picture in a picture frame before digital scanning?
How does Belgium enforce obligatory attendance in elections?
Maximum summed subsequences with non-adjacent items
Trademark violation for app?
What does Turing mean by this statement?
What happened to Thoros of Myr's flaming sword?
File name problem(?)
How to write capital alpha?
Is CEO the "profession" with the most psychopaths?
A letter with no particular backstory
Central Vacuuming: Is it worth it, and how does it compare to normal vacuuming?
What does it mean that physics no longer uses mechanical models to describe phenomena?
Should a wizard buy fine inks every time he want to copy spells into his spellbook?
What would you call this weird metallic apparatus that allows you to lift people?
Most bit efficient text communication method?
Decovolution function
Announcing the arrival of Valued Associate #679: Cesar Manara
Planned maintenance scheduled April 23, 2019 at 00:00UTC (8:00pm US/Eastern)
2019 Moderator Election Q&A - Questionnaire
2019 Community Moderator Election ResultsDeconvolutional Network in Semantic SegmentationPrepending Input layer to pre-trained modelUsing deconvolution in practiceIn a convolutional neural network (CNN), when convolving the image, is the operation used the dot product or the sum of element-wise multiplication?I still don't know how deconvolution works after watching CS231 lecture, I need helpDeep learning with Tensorflow: training with big data setsAppending to numpy array for creating datasetTranspose convolution math not working outCalculating sin function with neural network using python
$begingroup$
I have an image (for example (7x7x3) and a filter (3x3x3)). I convolved the image with the filter and it became a (3x3) output. If I want to do the inverse operation and want it to become the image from the output and the filter. How can I implement this operation in Python with Numpy?
I don't know which operation I should use with the filter (inverse or transpose)?
Here is my code for the Deconvolution:
import numpy as np
def deConv(Z, cashe):
'''
deConv calculate the transpoe Convoultion between the output of the ConvNet and the filter
Arguments:
Z-- Output of the ConvNet Layer, an array of the shape()
'''
# Retrieve information from "cache"
(X_prev, W, b, s, p) = cashe
# Retrieve dimensions from X_prev's shape
(m, n_H_prev, n_W_prev, n_C_prev) = X_prev.shape
# Retrieve dimensions from W's shape
(f, f, n_C_prev, n_C) = W.shape
# Retrieve dimensions from Z's shape
(m, n_H, n_W, n_C) = Z.shape
#create initial array for the output of the Deconvolution
X_curr = np.zeros((m, n_H_prev, n_W_prev, n_C_prev))
#loop over the Training examples
for i in range (m):
#loop over the vertical of the output
for h in range(n_H):
#loop over the horizontal of the output
for w in range(n_W):
#loop over the
for c in range (n_C):
#loop over the color channels
for x in range(n_C_prev):
#inverse_W = np.linalg.pinv(W[:, :, x, c])
transpose_W = np.transpose(W[:,:,x,c])
#X_curr[i, h*s:h*s+f, w*s:w*s+f, x] += Z[i, h, w, c] * inverse_W
X_curr[i, h*s:h*s+f, w*s:w*s+f, x] += Z[i, h, w, c] * transpose_W
X_curr[i, h*s:h*s+f, w*s:w*s+f, :] += b[:,:,:,c]
X_curr = relu(X_curr)
return X_curr
python deep-learning convolution numpy
$endgroup$
add a comment |
$begingroup$
I have an image (for example (7x7x3) and a filter (3x3x3)). I convolved the image with the filter and it became a (3x3) output. If I want to do the inverse operation and want it to become the image from the output and the filter. How can I implement this operation in Python with Numpy?
I don't know which operation I should use with the filter (inverse or transpose)?
Here is my code for the Deconvolution:
import numpy as np
def deConv(Z, cashe):
'''
deConv calculate the transpoe Convoultion between the output of the ConvNet and the filter
Arguments:
Z-- Output of the ConvNet Layer, an array of the shape()
'''
# Retrieve information from "cache"
(X_prev, W, b, s, p) = cashe
# Retrieve dimensions from X_prev's shape
(m, n_H_prev, n_W_prev, n_C_prev) = X_prev.shape
# Retrieve dimensions from W's shape
(f, f, n_C_prev, n_C) = W.shape
# Retrieve dimensions from Z's shape
(m, n_H, n_W, n_C) = Z.shape
#create initial array for the output of the Deconvolution
X_curr = np.zeros((m, n_H_prev, n_W_prev, n_C_prev))
#loop over the Training examples
for i in range (m):
#loop over the vertical of the output
for h in range(n_H):
#loop over the horizontal of the output
for w in range(n_W):
#loop over the
for c in range (n_C):
#loop over the color channels
for x in range(n_C_prev):
#inverse_W = np.linalg.pinv(W[:, :, x, c])
transpose_W = np.transpose(W[:,:,x,c])
#X_curr[i, h*s:h*s+f, w*s:w*s+f, x] += Z[i, h, w, c] * inverse_W
X_curr[i, h*s:h*s+f, w*s:w*s+f, x] += Z[i, h, w, c] * transpose_W
X_curr[i, h*s:h*s+f, w*s:w*s+f, :] += b[:,:,:,c]
X_curr = relu(X_curr)
return X_curr
python deep-learning convolution numpy
$endgroup$
add a comment |
$begingroup$
I have an image (for example (7x7x3) and a filter (3x3x3)). I convolved the image with the filter and it became a (3x3) output. If I want to do the inverse operation and want it to become the image from the output and the filter. How can I implement this operation in Python with Numpy?
I don't know which operation I should use with the filter (inverse or transpose)?
Here is my code for the Deconvolution:
import numpy as np
def deConv(Z, cashe):
'''
deConv calculate the transpoe Convoultion between the output of the ConvNet and the filter
Arguments:
Z-- Output of the ConvNet Layer, an array of the shape()
'''
# Retrieve information from "cache"
(X_prev, W, b, s, p) = cashe
# Retrieve dimensions from X_prev's shape
(m, n_H_prev, n_W_prev, n_C_prev) = X_prev.shape
# Retrieve dimensions from W's shape
(f, f, n_C_prev, n_C) = W.shape
# Retrieve dimensions from Z's shape
(m, n_H, n_W, n_C) = Z.shape
#create initial array for the output of the Deconvolution
X_curr = np.zeros((m, n_H_prev, n_W_prev, n_C_prev))
#loop over the Training examples
for i in range (m):
#loop over the vertical of the output
for h in range(n_H):
#loop over the horizontal of the output
for w in range(n_W):
#loop over the
for c in range (n_C):
#loop over the color channels
for x in range(n_C_prev):
#inverse_W = np.linalg.pinv(W[:, :, x, c])
transpose_W = np.transpose(W[:,:,x,c])
#X_curr[i, h*s:h*s+f, w*s:w*s+f, x] += Z[i, h, w, c] * inverse_W
X_curr[i, h*s:h*s+f, w*s:w*s+f, x] += Z[i, h, w, c] * transpose_W
X_curr[i, h*s:h*s+f, w*s:w*s+f, :] += b[:,:,:,c]
X_curr = relu(X_curr)
return X_curr
python deep-learning convolution numpy
$endgroup$
I have an image (for example (7x7x3) and a filter (3x3x3)). I convolved the image with the filter and it became a (3x3) output. If I want to do the inverse operation and want it to become the image from the output and the filter. How can I implement this operation in Python with Numpy?
I don't know which operation I should use with the filter (inverse or transpose)?
Here is my code for the Deconvolution:
import numpy as np
def deConv(Z, cashe):
'''
deConv calculate the transpoe Convoultion between the output of the ConvNet and the filter
Arguments:
Z-- Output of the ConvNet Layer, an array of the shape()
'''
# Retrieve information from "cache"
(X_prev, W, b, s, p) = cashe
# Retrieve dimensions from X_prev's shape
(m, n_H_prev, n_W_prev, n_C_prev) = X_prev.shape
# Retrieve dimensions from W's shape
(f, f, n_C_prev, n_C) = W.shape
# Retrieve dimensions from Z's shape
(m, n_H, n_W, n_C) = Z.shape
#create initial array for the output of the Deconvolution
X_curr = np.zeros((m, n_H_prev, n_W_prev, n_C_prev))
#loop over the Training examples
for i in range (m):
#loop over the vertical of the output
for h in range(n_H):
#loop over the horizontal of the output
for w in range(n_W):
#loop over the
for c in range (n_C):
#loop over the color channels
for x in range(n_C_prev):
#inverse_W = np.linalg.pinv(W[:, :, x, c])
transpose_W = np.transpose(W[:,:,x,c])
#X_curr[i, h*s:h*s+f, w*s:w*s+f, x] += Z[i, h, w, c] * inverse_W
X_curr[i, h*s:h*s+f, w*s:w*s+f, x] += Z[i, h, w, c] * transpose_W
X_curr[i, h*s:h*s+f, w*s:w*s+f, :] += b[:,:,:,c]
X_curr = relu(X_curr)
return X_curr
python deep-learning convolution numpy
python deep-learning convolution numpy
edited Apr 7 at 0:27


Stephen Rauch♦
1,52551330
1,52551330
asked Apr 6 at 22:39


Edward AlhanounEdward Alhanoun
33
33
add a comment |
add a comment |
1 Answer
1
active
oldest
votes
$begingroup$
This is probably irreversible operation unless the pre-convolution data was not full rank. But note that you reduced the dimensions of your signal, so the convolution was probably cropped to "valid" information (which doesn't need padding)
If you have at least the image or the filter, recovery might be possible, since convolution can be inverted using deconvolution but note that:
Convolution is defined as
$$ f(x) circledast g(x) = h(x) = int_-infty^inftyf(x-t)g(t)dt $$The some integral transforms (such as Fourier and Laplace) have the property that:
$$ Tf(x) circledast g(x)(s) = Th(x)(s) = Tf(x)(s) times Tg(x)(s) $$
- This is true for the Discrete Fourier Transform and Discrete Convolution as well, so to find your image $i$ from the filtered image $j$ by a filter $h$ given $I$,$J$ and $H$ as the Discrete Fourier transform of $i$,$j$ and $h$, respectively. Let $F.$ denote the discrete fourier transform and $F^-1.$ denote its inverse transformation:
$$ i = F^-1I = F^-1fracJH $$
- The discrete fourier transform is implemented efficiently by SciPy(signal module), FFTW, NumPy(fft module) and probably Theano. Having a lot of wrappers arround.
Note 1: Is important to notice that you will need at least an estimation of your filter, there are a lot of algorithms to do so.
Note 2: Deconvolution is very sensitive to noise, you can check on this class on Digital Image Processing to understand image filtering, mainly the part on Wiener filters.
Note 3: Image Deconvolution is implemented on scikit-image (e.g. Unsupervised Wiener) and on OpenCV using many algorithms (also on matlab in Image Processing Toolbox).
$endgroup$
add a comment |
Your Answer
StackExchange.ready(function()
var channelOptions =
tags: "".split(" "),
id: "557"
;
initTagRenderer("".split(" "), "".split(" "), channelOptions);
StackExchange.using("externalEditor", function()
// Have to fire editor after snippets, if snippets enabled
if (StackExchange.settings.snippets.snippetsEnabled)
StackExchange.using("snippets", function()
createEditor();
);
else
createEditor();
);
function createEditor()
StackExchange.prepareEditor(
heartbeatType: 'answer',
autoActivateHeartbeat: false,
convertImagesToLinks: false,
noModals: true,
showLowRepImageUploadWarning: true,
reputationToPostImages: null,
bindNavPrevention: true,
postfix: "",
imageUploader:
brandingHtml: "Powered by u003ca class="icon-imgur-white" href="https://imgur.com/"u003eu003c/au003e",
contentPolicyHtml: "User contributions licensed under u003ca href="https://creativecommons.org/licenses/by-sa/3.0/"u003ecc by-sa 3.0 with attribution requiredu003c/au003e u003ca href="https://stackoverflow.com/legal/content-policy"u003e(content policy)u003c/au003e",
allowUrls: true
,
onDemand: true,
discardSelector: ".discard-answer"
,immediatelyShowMarkdownHelp:true
);
);
Sign up or log in
StackExchange.ready(function ()
StackExchange.helpers.onClickDraftSave('#login-link');
);
Sign up using Google
Sign up using Facebook
Sign up using Email and Password
Post as a guest
Required, but never shown
StackExchange.ready(
function ()
StackExchange.openid.initPostLogin('.new-post-login', 'https%3a%2f%2fdatascience.stackexchange.com%2fquestions%2f48776%2fdecovolution-function%23new-answer', 'question_page');
);
Post as a guest
Required, but never shown
1 Answer
1
active
oldest
votes
1 Answer
1
active
oldest
votes
active
oldest
votes
active
oldest
votes
$begingroup$
This is probably irreversible operation unless the pre-convolution data was not full rank. But note that you reduced the dimensions of your signal, so the convolution was probably cropped to "valid" information (which doesn't need padding)
If you have at least the image or the filter, recovery might be possible, since convolution can be inverted using deconvolution but note that:
Convolution is defined as
$$ f(x) circledast g(x) = h(x) = int_-infty^inftyf(x-t)g(t)dt $$The some integral transforms (such as Fourier and Laplace) have the property that:
$$ Tf(x) circledast g(x)(s) = Th(x)(s) = Tf(x)(s) times Tg(x)(s) $$
- This is true for the Discrete Fourier Transform and Discrete Convolution as well, so to find your image $i$ from the filtered image $j$ by a filter $h$ given $I$,$J$ and $H$ as the Discrete Fourier transform of $i$,$j$ and $h$, respectively. Let $F.$ denote the discrete fourier transform and $F^-1.$ denote its inverse transformation:
$$ i = F^-1I = F^-1fracJH $$
- The discrete fourier transform is implemented efficiently by SciPy(signal module), FFTW, NumPy(fft module) and probably Theano. Having a lot of wrappers arround.
Note 1: Is important to notice that you will need at least an estimation of your filter, there are a lot of algorithms to do so.
Note 2: Deconvolution is very sensitive to noise, you can check on this class on Digital Image Processing to understand image filtering, mainly the part on Wiener filters.
Note 3: Image Deconvolution is implemented on scikit-image (e.g. Unsupervised Wiener) and on OpenCV using many algorithms (also on matlab in Image Processing Toolbox).
$endgroup$
add a comment |
$begingroup$
This is probably irreversible operation unless the pre-convolution data was not full rank. But note that you reduced the dimensions of your signal, so the convolution was probably cropped to "valid" information (which doesn't need padding)
If you have at least the image or the filter, recovery might be possible, since convolution can be inverted using deconvolution but note that:
Convolution is defined as
$$ f(x) circledast g(x) = h(x) = int_-infty^inftyf(x-t)g(t)dt $$The some integral transforms (such as Fourier and Laplace) have the property that:
$$ Tf(x) circledast g(x)(s) = Th(x)(s) = Tf(x)(s) times Tg(x)(s) $$
- This is true for the Discrete Fourier Transform and Discrete Convolution as well, so to find your image $i$ from the filtered image $j$ by a filter $h$ given $I$,$J$ and $H$ as the Discrete Fourier transform of $i$,$j$ and $h$, respectively. Let $F.$ denote the discrete fourier transform and $F^-1.$ denote its inverse transformation:
$$ i = F^-1I = F^-1fracJH $$
- The discrete fourier transform is implemented efficiently by SciPy(signal module), FFTW, NumPy(fft module) and probably Theano. Having a lot of wrappers arround.
Note 1: Is important to notice that you will need at least an estimation of your filter, there are a lot of algorithms to do so.
Note 2: Deconvolution is very sensitive to noise, you can check on this class on Digital Image Processing to understand image filtering, mainly the part on Wiener filters.
Note 3: Image Deconvolution is implemented on scikit-image (e.g. Unsupervised Wiener) and on OpenCV using many algorithms (also on matlab in Image Processing Toolbox).
$endgroup$
add a comment |
$begingroup$
This is probably irreversible operation unless the pre-convolution data was not full rank. But note that you reduced the dimensions of your signal, so the convolution was probably cropped to "valid" information (which doesn't need padding)
If you have at least the image or the filter, recovery might be possible, since convolution can be inverted using deconvolution but note that:
Convolution is defined as
$$ f(x) circledast g(x) = h(x) = int_-infty^inftyf(x-t)g(t)dt $$The some integral transforms (such as Fourier and Laplace) have the property that:
$$ Tf(x) circledast g(x)(s) = Th(x)(s) = Tf(x)(s) times Tg(x)(s) $$
- This is true for the Discrete Fourier Transform and Discrete Convolution as well, so to find your image $i$ from the filtered image $j$ by a filter $h$ given $I$,$J$ and $H$ as the Discrete Fourier transform of $i$,$j$ and $h$, respectively. Let $F.$ denote the discrete fourier transform and $F^-1.$ denote its inverse transformation:
$$ i = F^-1I = F^-1fracJH $$
- The discrete fourier transform is implemented efficiently by SciPy(signal module), FFTW, NumPy(fft module) and probably Theano. Having a lot of wrappers arround.
Note 1: Is important to notice that you will need at least an estimation of your filter, there are a lot of algorithms to do so.
Note 2: Deconvolution is very sensitive to noise, you can check on this class on Digital Image Processing to understand image filtering, mainly the part on Wiener filters.
Note 3: Image Deconvolution is implemented on scikit-image (e.g. Unsupervised Wiener) and on OpenCV using many algorithms (also on matlab in Image Processing Toolbox).
$endgroup$
This is probably irreversible operation unless the pre-convolution data was not full rank. But note that you reduced the dimensions of your signal, so the convolution was probably cropped to "valid" information (which doesn't need padding)
If you have at least the image or the filter, recovery might be possible, since convolution can be inverted using deconvolution but note that:
Convolution is defined as
$$ f(x) circledast g(x) = h(x) = int_-infty^inftyf(x-t)g(t)dt $$The some integral transforms (such as Fourier and Laplace) have the property that:
$$ Tf(x) circledast g(x)(s) = Th(x)(s) = Tf(x)(s) times Tg(x)(s) $$
- This is true for the Discrete Fourier Transform and Discrete Convolution as well, so to find your image $i$ from the filtered image $j$ by a filter $h$ given $I$,$J$ and $H$ as the Discrete Fourier transform of $i$,$j$ and $h$, respectively. Let $F.$ denote the discrete fourier transform and $F^-1.$ denote its inverse transformation:
$$ i = F^-1I = F^-1fracJH $$
- The discrete fourier transform is implemented efficiently by SciPy(signal module), FFTW, NumPy(fft module) and probably Theano. Having a lot of wrappers arround.
Note 1: Is important to notice that you will need at least an estimation of your filter, there are a lot of algorithms to do so.
Note 2: Deconvolution is very sensitive to noise, you can check on this class on Digital Image Processing to understand image filtering, mainly the part on Wiener filters.
Note 3: Image Deconvolution is implemented on scikit-image (e.g. Unsupervised Wiener) and on OpenCV using many algorithms (also on matlab in Image Processing Toolbox).
answered Apr 7 at 3:40
Pedro Henrique MonfortePedro Henrique Monforte
559118
559118
add a comment |
add a comment |
Thanks for contributing an answer to Data Science Stack Exchange!
- Please be sure to answer the question. Provide details and share your research!
But avoid …
- Asking for help, clarification, or responding to other answers.
- Making statements based on opinion; back them up with references or personal experience.
Use MathJax to format equations. MathJax reference.
To learn more, see our tips on writing great answers.
Sign up or log in
StackExchange.ready(function ()
StackExchange.helpers.onClickDraftSave('#login-link');
);
Sign up using Google
Sign up using Facebook
Sign up using Email and Password
Post as a guest
Required, but never shown
StackExchange.ready(
function ()
StackExchange.openid.initPostLogin('.new-post-login', 'https%3a%2f%2fdatascience.stackexchange.com%2fquestions%2f48776%2fdecovolution-function%23new-answer', 'question_page');
);
Post as a guest
Required, but never shown
Sign up or log in
StackExchange.ready(function ()
StackExchange.helpers.onClickDraftSave('#login-link');
);
Sign up using Google
Sign up using Facebook
Sign up using Email and Password
Post as a guest
Required, but never shown
Sign up or log in
StackExchange.ready(function ()
StackExchange.helpers.onClickDraftSave('#login-link');
);
Sign up using Google
Sign up using Facebook
Sign up using Email and Password
Post as a guest
Required, but never shown
Sign up or log in
StackExchange.ready(function ()
StackExchange.helpers.onClickDraftSave('#login-link');
);
Sign up using Google
Sign up using Facebook
Sign up using Email and Password
Sign up using Google
Sign up using Facebook
Sign up using Email and Password
Post as a guest
Required, but never shown
Required, but never shown
Required, but never shown
Required, but never shown
Required, but never shown
Required, but never shown
Required, but never shown
Required, but never shown
Required, but never shown
b5hBn,E DxU 6vHSHJXv gQKPIQc6Kb,p4 8nJARi