Sequence to sequence RNN model, maximum number of training sizeImage clustering by similarity measurement (CW-SSIM)Time series prediction without sliding windowPreparing, Scaling and Selecting from a combination of numerical and categorical featuresRight Way to Input Text Data in Keras Auto EncoderHow to download dynamic files created during work on Google Colab?Keras val_acc unchanging when training (same label assigned to all images)Can you have too uniform test data in a feedforward neural network?Memory problems with smaller CNNUsing categorial_crossentropy to train a model in kerasApply Labeled LDA on large data
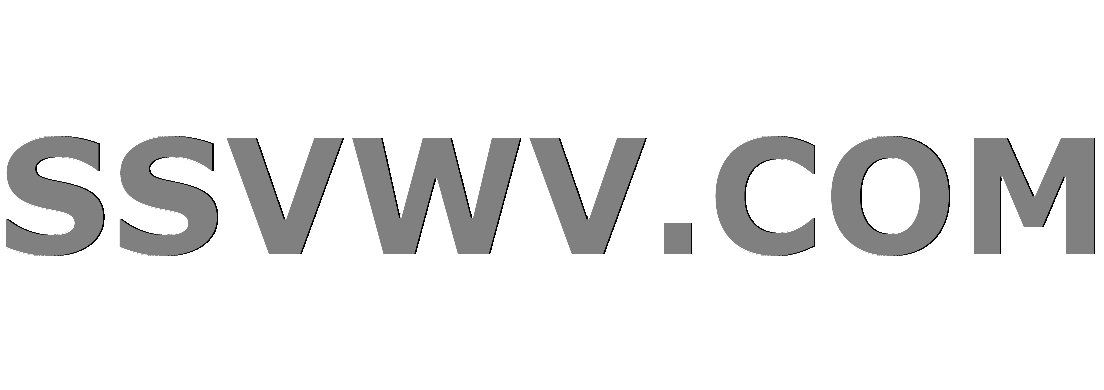
Multi tool use
Consistent Linux device enumeration
What is it called to attack a person then say something uplifting?
The garden where everything is possible
How do I tell my boss that I'm quitting in 15 days (a colleague left this week)
Naive definition of treewidth
What is this high flying aircraft over Pennsylvania?
Do I have to know the General Relativity theory to understand the concept of inertial frame?
Why didn’t Eve recognize the little cockroach as a living organism?
What properties make a magic weapon befit a Rogue more than a DEX-based Fighter?
Do you waste sorcery points if you try to apply metamagic to a spell from a scroll but fail to cast it?
Distinction between 地平線 【ちへいせん】 and 水平線 【すいへいせん】
Why do Radio Buttons not fill the entire outer circle?
Why is the sun approximated as a black body at ~ 5800 K?
Pre-Employment Background Check With Consent For Future Checks
If Captain Marvel (MCU) marries a human male, will they have human or Kree children?
Why didn't Voldemort know what Grindelwald looked like?
Writing in a Christian voice
How much do grades matter for a future academia position?
How to predict the next number in a series while having additional series of data that might affect it?
Typing CO_2 easily
Reason why a kingside attack is not justified
Limit max CPU usage SQL SERVER with WSRM
Showing mass murder in a kid's book
Efficiently query two properties QGIS spatialite
Sequence to sequence RNN model, maximum number of training size
Image clustering by similarity measurement (CW-SSIM)Time series prediction without sliding windowPreparing, Scaling and Selecting from a combination of numerical and categorical featuresRight Way to Input Text Data in Keras Auto EncoderHow to download dynamic files created during work on Google Colab?Keras val_acc unchanging when training (same label assigned to all images)Can you have too uniform test data in a feedforward neural network?Memory problems with smaller CNNUsing categorial_crossentropy to train a model in kerasApply Labeled LDA on large data
$begingroup$
So when running this example script from Keras repo (https://github.com/keras-team/keras/blob/master/examples/lstm_seq2seq.py), I found that we can easily run into out of memory for the input or output one-hot encoding in this code:
encoder_input_data = np.zeros(
(len(input_texts), max_encoder_seq_length, num_encoder_tokens),
dtype='float32')
decoder_input_data = np.zeros(
(len(input_texts), max_decoder_seq_length, num_decoder_tokens),
dtype='float32')
decoder_target_data = np.zeros(
(len(input_texts), max_decoder_seq_length, num_decoder_tokens),
dtype='float32')
When I have a huge size of training samples, this one-hot does not fit into memory. Is there way to handle this issue?
UPDATE:
I changed float32 to uint, but thats about the smallest one hot array can get.
python deep-learning numpy
$endgroup$
bumped to the homepage by Community♦ 2 days ago
This question has answers that may be good or bad; the system has marked it active so that they can be reviewed.
add a comment |
$begingroup$
So when running this example script from Keras repo (https://github.com/keras-team/keras/blob/master/examples/lstm_seq2seq.py), I found that we can easily run into out of memory for the input or output one-hot encoding in this code:
encoder_input_data = np.zeros(
(len(input_texts), max_encoder_seq_length, num_encoder_tokens),
dtype='float32')
decoder_input_data = np.zeros(
(len(input_texts), max_decoder_seq_length, num_decoder_tokens),
dtype='float32')
decoder_target_data = np.zeros(
(len(input_texts), max_decoder_seq_length, num_decoder_tokens),
dtype='float32')
When I have a huge size of training samples, this one-hot does not fit into memory. Is there way to handle this issue?
UPDATE:
I changed float32 to uint, but thats about the smallest one hot array can get.
python deep-learning numpy
$endgroup$
bumped to the homepage by Community♦ 2 days ago
This question has answers that may be good or bad; the system has marked it active so that they can be reviewed.
add a comment |
$begingroup$
So when running this example script from Keras repo (https://github.com/keras-team/keras/blob/master/examples/lstm_seq2seq.py), I found that we can easily run into out of memory for the input or output one-hot encoding in this code:
encoder_input_data = np.zeros(
(len(input_texts), max_encoder_seq_length, num_encoder_tokens),
dtype='float32')
decoder_input_data = np.zeros(
(len(input_texts), max_decoder_seq_length, num_decoder_tokens),
dtype='float32')
decoder_target_data = np.zeros(
(len(input_texts), max_decoder_seq_length, num_decoder_tokens),
dtype='float32')
When I have a huge size of training samples, this one-hot does not fit into memory. Is there way to handle this issue?
UPDATE:
I changed float32 to uint, but thats about the smallest one hot array can get.
python deep-learning numpy
$endgroup$
So when running this example script from Keras repo (https://github.com/keras-team/keras/blob/master/examples/lstm_seq2seq.py), I found that we can easily run into out of memory for the input or output one-hot encoding in this code:
encoder_input_data = np.zeros(
(len(input_texts), max_encoder_seq_length, num_encoder_tokens),
dtype='float32')
decoder_input_data = np.zeros(
(len(input_texts), max_decoder_seq_length, num_decoder_tokens),
dtype='float32')
decoder_target_data = np.zeros(
(len(input_texts), max_decoder_seq_length, num_decoder_tokens),
dtype='float32')
When I have a huge size of training samples, this one-hot does not fit into memory. Is there way to handle this issue?
UPDATE:
I changed float32 to uint, but thats about the smallest one hot array can get.
python deep-learning numpy
python deep-learning numpy
edited Feb 15 at 15:06
eugen
asked Feb 15 at 14:30


eugeneugen
11
11
bumped to the homepage by Community♦ 2 days ago
This question has answers that may be good or bad; the system has marked it active so that they can be reviewed.
bumped to the homepage by Community♦ 2 days ago
This question has answers that may be good or bad; the system has marked it active so that they can be reviewed.
add a comment |
add a comment |
1 Answer
1
active
oldest
votes
$begingroup$
so I found a solution by dividing input training data into small batches and it did the trick. Here is the code:
divideby=1000
for j in range(divideby):
start=j*len(input_texts)/divideby
end=(j+1)*len(input_texts)/divideby if j<divideby+1 else -1
encoder_input_data = np.zeros(
(end-start, max_encoder_seq_length, num_encoder_tokens),
dtype='uint8') # size_of_training_samples, max_length_word_measured_in_characters,number_of_unique_chars,
decoder_input_data = np.zeros(
(end-start, max_decoder_seq_length, num_decoder_tokens),
dtype='uint8')
decoder_target_data = np.zeros(
(end-start, max_decoder_seq_length, num_decoder_tokens),
dtype='uint8')
for i, (input_text, target_text) in enumerate(zip(input_texts[start:end], target_texts[start:end])):
for t, char in enumerate(input_text.split(splitby)):
encoder_input_data[i, t, input_token_index[char]] = 1.
for t, char in enumerate(target_text.split(splitby)):
# decoder_target_data is ahead of decoder_input_data by one timestep
decoder_input_data[i, t, target_token_index[char]] = 1.
if t > 0:
# decoder_target_data will be ahead by one timestep
# and will not include the start character.
decoder_target_data[i, t - 1, target_token_index[char]] = 1.
model.fit([encoder_input_data, decoder_input_data], decoder_target_data,
batch_size=batch_size,
epochs=epochs,
validation_split=0.2)
```
$endgroup$
add a comment |
Your Answer
StackExchange.ifUsing("editor", function ()
return StackExchange.using("mathjaxEditing", function ()
StackExchange.MarkdownEditor.creationCallbacks.add(function (editor, postfix)
StackExchange.mathjaxEditing.prepareWmdForMathJax(editor, postfix, [["$", "$"], ["\\(","\\)"]]);
);
);
, "mathjax-editing");
StackExchange.ready(function()
var channelOptions =
tags: "".split(" "),
id: "557"
;
initTagRenderer("".split(" "), "".split(" "), channelOptions);
StackExchange.using("externalEditor", function()
// Have to fire editor after snippets, if snippets enabled
if (StackExchange.settings.snippets.snippetsEnabled)
StackExchange.using("snippets", function()
createEditor();
);
else
createEditor();
);
function createEditor()
StackExchange.prepareEditor(
heartbeatType: 'answer',
autoActivateHeartbeat: false,
convertImagesToLinks: false,
noModals: true,
showLowRepImageUploadWarning: true,
reputationToPostImages: null,
bindNavPrevention: true,
postfix: "",
imageUploader:
brandingHtml: "Powered by u003ca class="icon-imgur-white" href="https://imgur.com/"u003eu003c/au003e",
contentPolicyHtml: "User contributions licensed under u003ca href="https://creativecommons.org/licenses/by-sa/3.0/"u003ecc by-sa 3.0 with attribution requiredu003c/au003e u003ca href="https://stackoverflow.com/legal/content-policy"u003e(content policy)u003c/au003e",
allowUrls: true
,
onDemand: true,
discardSelector: ".discard-answer"
,immediatelyShowMarkdownHelp:true
);
);
Sign up or log in
StackExchange.ready(function ()
StackExchange.helpers.onClickDraftSave('#login-link');
);
Sign up using Google
Sign up using Facebook
Sign up using Email and Password
Post as a guest
Required, but never shown
StackExchange.ready(
function ()
StackExchange.openid.initPostLogin('.new-post-login', 'https%3a%2f%2fdatascience.stackexchange.com%2fquestions%2f45639%2fsequence-to-sequence-rnn-model-maximum-number-of-training-size%23new-answer', 'question_page');
);
Post as a guest
Required, but never shown
1 Answer
1
active
oldest
votes
1 Answer
1
active
oldest
votes
active
oldest
votes
active
oldest
votes
$begingroup$
so I found a solution by dividing input training data into small batches and it did the trick. Here is the code:
divideby=1000
for j in range(divideby):
start=j*len(input_texts)/divideby
end=(j+1)*len(input_texts)/divideby if j<divideby+1 else -1
encoder_input_data = np.zeros(
(end-start, max_encoder_seq_length, num_encoder_tokens),
dtype='uint8') # size_of_training_samples, max_length_word_measured_in_characters,number_of_unique_chars,
decoder_input_data = np.zeros(
(end-start, max_decoder_seq_length, num_decoder_tokens),
dtype='uint8')
decoder_target_data = np.zeros(
(end-start, max_decoder_seq_length, num_decoder_tokens),
dtype='uint8')
for i, (input_text, target_text) in enumerate(zip(input_texts[start:end], target_texts[start:end])):
for t, char in enumerate(input_text.split(splitby)):
encoder_input_data[i, t, input_token_index[char]] = 1.
for t, char in enumerate(target_text.split(splitby)):
# decoder_target_data is ahead of decoder_input_data by one timestep
decoder_input_data[i, t, target_token_index[char]] = 1.
if t > 0:
# decoder_target_data will be ahead by one timestep
# and will not include the start character.
decoder_target_data[i, t - 1, target_token_index[char]] = 1.
model.fit([encoder_input_data, decoder_input_data], decoder_target_data,
batch_size=batch_size,
epochs=epochs,
validation_split=0.2)
```
$endgroup$
add a comment |
$begingroup$
so I found a solution by dividing input training data into small batches and it did the trick. Here is the code:
divideby=1000
for j in range(divideby):
start=j*len(input_texts)/divideby
end=(j+1)*len(input_texts)/divideby if j<divideby+1 else -1
encoder_input_data = np.zeros(
(end-start, max_encoder_seq_length, num_encoder_tokens),
dtype='uint8') # size_of_training_samples, max_length_word_measured_in_characters,number_of_unique_chars,
decoder_input_data = np.zeros(
(end-start, max_decoder_seq_length, num_decoder_tokens),
dtype='uint8')
decoder_target_data = np.zeros(
(end-start, max_decoder_seq_length, num_decoder_tokens),
dtype='uint8')
for i, (input_text, target_text) in enumerate(zip(input_texts[start:end], target_texts[start:end])):
for t, char in enumerate(input_text.split(splitby)):
encoder_input_data[i, t, input_token_index[char]] = 1.
for t, char in enumerate(target_text.split(splitby)):
# decoder_target_data is ahead of decoder_input_data by one timestep
decoder_input_data[i, t, target_token_index[char]] = 1.
if t > 0:
# decoder_target_data will be ahead by one timestep
# and will not include the start character.
decoder_target_data[i, t - 1, target_token_index[char]] = 1.
model.fit([encoder_input_data, decoder_input_data], decoder_target_data,
batch_size=batch_size,
epochs=epochs,
validation_split=0.2)
```
$endgroup$
add a comment |
$begingroup$
so I found a solution by dividing input training data into small batches and it did the trick. Here is the code:
divideby=1000
for j in range(divideby):
start=j*len(input_texts)/divideby
end=(j+1)*len(input_texts)/divideby if j<divideby+1 else -1
encoder_input_data = np.zeros(
(end-start, max_encoder_seq_length, num_encoder_tokens),
dtype='uint8') # size_of_training_samples, max_length_word_measured_in_characters,number_of_unique_chars,
decoder_input_data = np.zeros(
(end-start, max_decoder_seq_length, num_decoder_tokens),
dtype='uint8')
decoder_target_data = np.zeros(
(end-start, max_decoder_seq_length, num_decoder_tokens),
dtype='uint8')
for i, (input_text, target_text) in enumerate(zip(input_texts[start:end], target_texts[start:end])):
for t, char in enumerate(input_text.split(splitby)):
encoder_input_data[i, t, input_token_index[char]] = 1.
for t, char in enumerate(target_text.split(splitby)):
# decoder_target_data is ahead of decoder_input_data by one timestep
decoder_input_data[i, t, target_token_index[char]] = 1.
if t > 0:
# decoder_target_data will be ahead by one timestep
# and will not include the start character.
decoder_target_data[i, t - 1, target_token_index[char]] = 1.
model.fit([encoder_input_data, decoder_input_data], decoder_target_data,
batch_size=batch_size,
epochs=epochs,
validation_split=0.2)
```
$endgroup$
so I found a solution by dividing input training data into small batches and it did the trick. Here is the code:
divideby=1000
for j in range(divideby):
start=j*len(input_texts)/divideby
end=(j+1)*len(input_texts)/divideby if j<divideby+1 else -1
encoder_input_data = np.zeros(
(end-start, max_encoder_seq_length, num_encoder_tokens),
dtype='uint8') # size_of_training_samples, max_length_word_measured_in_characters,number_of_unique_chars,
decoder_input_data = np.zeros(
(end-start, max_decoder_seq_length, num_decoder_tokens),
dtype='uint8')
decoder_target_data = np.zeros(
(end-start, max_decoder_seq_length, num_decoder_tokens),
dtype='uint8')
for i, (input_text, target_text) in enumerate(zip(input_texts[start:end], target_texts[start:end])):
for t, char in enumerate(input_text.split(splitby)):
encoder_input_data[i, t, input_token_index[char]] = 1.
for t, char in enumerate(target_text.split(splitby)):
# decoder_target_data is ahead of decoder_input_data by one timestep
decoder_input_data[i, t, target_token_index[char]] = 1.
if t > 0:
# decoder_target_data will be ahead by one timestep
# and will not include the start character.
decoder_target_data[i, t - 1, target_token_index[char]] = 1.
model.fit([encoder_input_data, decoder_input_data], decoder_target_data,
batch_size=batch_size,
epochs=epochs,
validation_split=0.2)
```
answered Feb 15 at 16:13


eugeneugen
11
11
add a comment |
add a comment |
Thanks for contributing an answer to Data Science Stack Exchange!
- Please be sure to answer the question. Provide details and share your research!
But avoid …
- Asking for help, clarification, or responding to other answers.
- Making statements based on opinion; back them up with references or personal experience.
Use MathJax to format equations. MathJax reference.
To learn more, see our tips on writing great answers.
Sign up or log in
StackExchange.ready(function ()
StackExchange.helpers.onClickDraftSave('#login-link');
);
Sign up using Google
Sign up using Facebook
Sign up using Email and Password
Post as a guest
Required, but never shown
StackExchange.ready(
function ()
StackExchange.openid.initPostLogin('.new-post-login', 'https%3a%2f%2fdatascience.stackexchange.com%2fquestions%2f45639%2fsequence-to-sequence-rnn-model-maximum-number-of-training-size%23new-answer', 'question_page');
);
Post as a guest
Required, but never shown
Sign up or log in
StackExchange.ready(function ()
StackExchange.helpers.onClickDraftSave('#login-link');
);
Sign up using Google
Sign up using Facebook
Sign up using Email and Password
Post as a guest
Required, but never shown
Sign up or log in
StackExchange.ready(function ()
StackExchange.helpers.onClickDraftSave('#login-link');
);
Sign up using Google
Sign up using Facebook
Sign up using Email and Password
Post as a guest
Required, but never shown
Sign up or log in
StackExchange.ready(function ()
StackExchange.helpers.onClickDraftSave('#login-link');
);
Sign up using Google
Sign up using Facebook
Sign up using Email and Password
Sign up using Google
Sign up using Facebook
Sign up using Email and Password
Post as a guest
Required, but never shown
Required, but never shown
Required, but never shown
Required, but never shown
Required, but never shown
Required, but never shown
Required, but never shown
Required, but never shown
Required, but never shown
6i 9HDjhFJ bNxY6C8qG5CopV5oOHepxGlQPgv9roCagb,2NFlSbDLLRTl9fuX15 wfXbVe