Multilabel classifcation in sklearn with soft (fuzzy) labels2019 Community Moderator ElectionBalanced Linear SVM wins every class except One vs AllWhat is the difference between multilabel dataset and special dataset with respect to imbalance problem in datasets?Classifying multilabel images with TensorFlowdata type (int vs float) with sklearn modelsMultilabel image classification: is it necessary to have traning data for each combination of labels?matching results with sklearn average_precision_scoreWhat does it mean that classes are mutually exlcusive but soft-labels are accepeted?Unbalanced multi-class : distribution might change as more data come inHow to visualize results/errors of multilabel classifiers?Multilabel Classification With Ranking
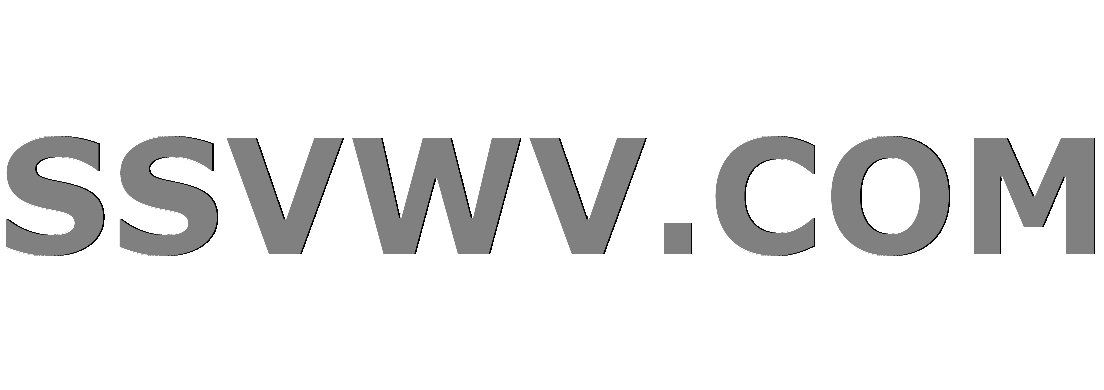
Multi tool use
What typically incentivizes a professor to change jobs to a lower ranking university?
Are the number of citations and number of published articles the most important criteria for a tenure promotion?
DC-DC converter from low voltage at high current, to high voltage at low current
Do infinite dimensional systems make sense?
Today is the Center
A case of the sniffles
How is it possible to have an ability score that is less than 3?
Question on branch cuts and branch points
Rock identification in KY
Do I have a twin with permutated remainders?
Client team has low performances and low technical skills: we always fix their work and now they stop collaborate with us. How to solve?
Is it possible to do 50 km distance without any previous training?
High voltage LED indicator 40-1000 VDC without additional power supply
Is it possible to run Internet Explorer on OS X El Capitan?
Why are electrically insulating heatsinks so rare? Is it just cost?
NMaximize is not converging to a solution
Linear Path Optimization with Two Dependent Variables
Accidentally leaked the solution to an assignment, what to do now? (I'm the prof)
Revoked SSL certificate
Why doesn't H₄O²⁺ exist?
Are astronomers waiting to see something in an image from a gravitational lens that they've already seen in an adjacent image?
What's that red-plus icon near a text?
Alternative to sending password over mail?
How to format long polynomial?
Multilabel classifcation in sklearn with soft (fuzzy) labels
2019 Community Moderator ElectionBalanced Linear SVM wins every class except One vs AllWhat is the difference between multilabel dataset and special dataset with respect to imbalance problem in datasets?Classifying multilabel images with TensorFlowdata type (int vs float) with sklearn modelsMultilabel image classification: is it necessary to have traning data for each combination of labels?matching results with sklearn average_precision_scoreWhat does it mean that classes are mutually exlcusive but soft-labels are accepeted?Unbalanced multi-class : distribution might change as more data come inHow to visualize results/errors of multilabel classifiers?Multilabel Classification With Ranking
$begingroup$
I have a model which is trained in sklearn on a 5-way classification problem, which performs relatively well (there are kNN and SVM versions, and both reproduce a test set with high accuracy).
When the model is applied in "real life", it is highly likely that many samples will contain linear combinations of multiple classes. So a sample may be 70% class A and 30% class B.
Much of what I have read about multilabel classification in sklearn relates to problems which don't fit this paradigm well, most of them are "tagging" type problems such as movie genre classification. Is there a way to apply my SVM/kNN models to this type of problem? I would prefer to only train on single-class examples but can modify the training set to create some multi-class samples too.
It seems I could work this by simply doing an indivdiual binary classifier for each class. However, this wouldn't give me the relative strength of each label, i.e. the linear coefficient. Is that possible?
classification scikit-learn multilabel-classification
$endgroup$
add a comment |
$begingroup$
I have a model which is trained in sklearn on a 5-way classification problem, which performs relatively well (there are kNN and SVM versions, and both reproduce a test set with high accuracy).
When the model is applied in "real life", it is highly likely that many samples will contain linear combinations of multiple classes. So a sample may be 70% class A and 30% class B.
Much of what I have read about multilabel classification in sklearn relates to problems which don't fit this paradigm well, most of them are "tagging" type problems such as movie genre classification. Is there a way to apply my SVM/kNN models to this type of problem? I would prefer to only train on single-class examples but can modify the training set to create some multi-class samples too.
It seems I could work this by simply doing an indivdiual binary classifier for each class. However, this wouldn't give me the relative strength of each label, i.e. the linear coefficient. Is that possible?
classification scikit-learn multilabel-classification
$endgroup$
add a comment |
$begingroup$
I have a model which is trained in sklearn on a 5-way classification problem, which performs relatively well (there are kNN and SVM versions, and both reproduce a test set with high accuracy).
When the model is applied in "real life", it is highly likely that many samples will contain linear combinations of multiple classes. So a sample may be 70% class A and 30% class B.
Much of what I have read about multilabel classification in sklearn relates to problems which don't fit this paradigm well, most of them are "tagging" type problems such as movie genre classification. Is there a way to apply my SVM/kNN models to this type of problem? I would prefer to only train on single-class examples but can modify the training set to create some multi-class samples too.
It seems I could work this by simply doing an indivdiual binary classifier for each class. However, this wouldn't give me the relative strength of each label, i.e. the linear coefficient. Is that possible?
classification scikit-learn multilabel-classification
$endgroup$
I have a model which is trained in sklearn on a 5-way classification problem, which performs relatively well (there are kNN and SVM versions, and both reproduce a test set with high accuracy).
When the model is applied in "real life", it is highly likely that many samples will contain linear combinations of multiple classes. So a sample may be 70% class A and 30% class B.
Much of what I have read about multilabel classification in sklearn relates to problems which don't fit this paradigm well, most of them are "tagging" type problems such as movie genre classification. Is there a way to apply my SVM/kNN models to this type of problem? I would prefer to only train on single-class examples but can modify the training set to create some multi-class samples too.
It seems I could work this by simply doing an indivdiual binary classifier for each class. However, this wouldn't give me the relative strength of each label, i.e. the linear coefficient. Is that possible?
classification scikit-learn multilabel-classification
classification scikit-learn multilabel-classification
edited Apr 2 at 12:32
Esmailian
2,621318
2,621318
asked Mar 27 at 21:53
asher1213asher1213
261
261
add a comment |
add a comment |
1 Answer
1
active
oldest
votes
$begingroup$
For example, for a 3-class classification, we want to train with a label like $A$, which is one-hot encoded as $(1, 0, 0)$, and also with a fuzzy label like $(0.8, 0.2, 0)$. In that case, kNN and SVM of sklearn does not support fuzzy labels.
However, we can use sklearn's MultiOutputRegressor
that extends a one-output Regressor such as Support Vector Regression (SVR) to multiple outputs. It is worth noting that neural networks are a natural fit for this type of label since they readily work with numerical vectors as labels.
Here is a code that goes through different types of labels for kNN, SVC (multi-class SVM), and MultiRegression SVR:
import sklearn
import pandas as pd
from sklearn.svm import SVC, SVR
from sklearn.model_selection import KFold, cross_val_score
from sklearn.neighbors import KNeighborsClassifier
from sklearn.multioutput import MultiOutputRegressor
import numpy as np
N = 1000
split = int(0.8 * N)
folds = 5
seed = 1234
# Data
np.random.seed(seed)
feature_1 = np.random.normal(0, 2, N)
feature_2 = np.random.normal(5, 6, N)
X = np.vstack([feature_1, feature_2]).T
Y_label = np.random.choice(['A', 'B', 'C'], N)
Y_one_hot = pd.get_dummies(Y_label).values
smooth_filter = np.array([0.01, 0.98, 0.01])
Y_fuzzy = np.apply_along_axis(
lambda m: np.convolve(m, smooth_filter, mode='same'), axis=1, arr=Y_one_hot
)
kfold = KFold(n_splits=folds, random_state=seed)
kNN = KNeighborsClassifier(n_neighbors=3)
svc = SVC()
svr = SVR()
multi_svr = MultiOutputRegressor(estimator=SVR())
knn_label = np.average(cross_val_score(kNN, X, Y_label, cv=kfold))
knn_one_hot = np.average(cross_val_score(kNN, X, Y_one_hot, cv=kfold))
try:
knn_fuzzy = np.average(cross_val_score(kNN, X, Y_fuzzy, cv=kfold))
except ValueError:
print('kNN: fuzzy classes are not supported')
svc_label = np.average(cross_val_score(svc, X, Y_label, cv=kfold))
try:
svc_one_hot = np.average(cross_val_score(svc, X, Y_one_hot, cv=kfold))
except ValueError:
print('SVC: vector is not supported')
try:
svr_one_hot = np.average(cross_val_score(svr, X, Y_one_hot, cv=kfold))
except ValueError:
print('SVR: vector is not supported')
multi_svr_one_hot = np.average(cross_val_score(multi_svr, X, Y_one_hot, cv=kfold, scoring='neg_mean_absolute_error'))
multi_svr_fuzzy = np.average(cross_val_score(multi_svr, X, Y_fuzzy, cv=kfold, scoring='neg_mean_absolute_error'))
print('sklearn version', sklearn.__version__)
print('Y example: ',
"label: ", Y_label[0],
", one hot: ", Y_one_hot[0, :],
", fuzzy: ", Y_fuzzy[0, :])
print('kNN label: ', knn_label)
print('kNN one hot: ', knn_one_hot)
print('SVC label: ', svc_label)
print('MultiSVR one hot: ', multi_svr_one_hot)
print('MultiSVR fuzzy: ', multi_svr_fuzzy)
Output:
kNN: fuzzy classes are not supported
SVC: vector is not supported
SVR: vector is not supported
sklearn version 0.19.1
Y example: label: B , one hot: [0 1 0] , fuzzy: [0.01 0.98 0.01]
kNN label: 0.321
kNN one hot: 0.254
SVC label: 0.332
MultiSVR one hot: -0.4066160996805417
MultiSVR fuzzy: -0.3970780923514713
Although kNN does not throw an exception for one-hot encoded labels, accuracy 0.254
shows that it does not work correctly with the vector.
Also, Negative Mean Absolute Error is reported for MultiSVR since the task is understood as regression. Score accuracy
can only be used after changing the fuzzy labels and predictions back to a label.
$endgroup$
add a comment |
Your Answer
StackExchange.ifUsing("editor", function ()
return StackExchange.using("mathjaxEditing", function ()
StackExchange.MarkdownEditor.creationCallbacks.add(function (editor, postfix)
StackExchange.mathjaxEditing.prepareWmdForMathJax(editor, postfix, [["$", "$"], ["\\(","\\)"]]);
);
);
, "mathjax-editing");
StackExchange.ready(function()
var channelOptions =
tags: "".split(" "),
id: "557"
;
initTagRenderer("".split(" "), "".split(" "), channelOptions);
StackExchange.using("externalEditor", function()
// Have to fire editor after snippets, if snippets enabled
if (StackExchange.settings.snippets.snippetsEnabled)
StackExchange.using("snippets", function()
createEditor();
);
else
createEditor();
);
function createEditor()
StackExchange.prepareEditor(
heartbeatType: 'answer',
autoActivateHeartbeat: false,
convertImagesToLinks: false,
noModals: true,
showLowRepImageUploadWarning: true,
reputationToPostImages: null,
bindNavPrevention: true,
postfix: "",
imageUploader:
brandingHtml: "Powered by u003ca class="icon-imgur-white" href="https://imgur.com/"u003eu003c/au003e",
contentPolicyHtml: "User contributions licensed under u003ca href="https://creativecommons.org/licenses/by-sa/3.0/"u003ecc by-sa 3.0 with attribution requiredu003c/au003e u003ca href="https://stackoverflow.com/legal/content-policy"u003e(content policy)u003c/au003e",
allowUrls: true
,
onDemand: true,
discardSelector: ".discard-answer"
,immediatelyShowMarkdownHelp:true
);
);
Sign up or log in
StackExchange.ready(function ()
StackExchange.helpers.onClickDraftSave('#login-link');
);
Sign up using Google
Sign up using Facebook
Sign up using Email and Password
Post as a guest
Required, but never shown
StackExchange.ready(
function ()
StackExchange.openid.initPostLogin('.new-post-login', 'https%3a%2f%2fdatascience.stackexchange.com%2fquestions%2f48111%2fmultilabel-classifcation-in-sklearn-with-soft-fuzzy-labels%23new-answer', 'question_page');
);
Post as a guest
Required, but never shown
1 Answer
1
active
oldest
votes
1 Answer
1
active
oldest
votes
active
oldest
votes
active
oldest
votes
$begingroup$
For example, for a 3-class classification, we want to train with a label like $A$, which is one-hot encoded as $(1, 0, 0)$, and also with a fuzzy label like $(0.8, 0.2, 0)$. In that case, kNN and SVM of sklearn does not support fuzzy labels.
However, we can use sklearn's MultiOutputRegressor
that extends a one-output Regressor such as Support Vector Regression (SVR) to multiple outputs. It is worth noting that neural networks are a natural fit for this type of label since they readily work with numerical vectors as labels.
Here is a code that goes through different types of labels for kNN, SVC (multi-class SVM), and MultiRegression SVR:
import sklearn
import pandas as pd
from sklearn.svm import SVC, SVR
from sklearn.model_selection import KFold, cross_val_score
from sklearn.neighbors import KNeighborsClassifier
from sklearn.multioutput import MultiOutputRegressor
import numpy as np
N = 1000
split = int(0.8 * N)
folds = 5
seed = 1234
# Data
np.random.seed(seed)
feature_1 = np.random.normal(0, 2, N)
feature_2 = np.random.normal(5, 6, N)
X = np.vstack([feature_1, feature_2]).T
Y_label = np.random.choice(['A', 'B', 'C'], N)
Y_one_hot = pd.get_dummies(Y_label).values
smooth_filter = np.array([0.01, 0.98, 0.01])
Y_fuzzy = np.apply_along_axis(
lambda m: np.convolve(m, smooth_filter, mode='same'), axis=1, arr=Y_one_hot
)
kfold = KFold(n_splits=folds, random_state=seed)
kNN = KNeighborsClassifier(n_neighbors=3)
svc = SVC()
svr = SVR()
multi_svr = MultiOutputRegressor(estimator=SVR())
knn_label = np.average(cross_val_score(kNN, X, Y_label, cv=kfold))
knn_one_hot = np.average(cross_val_score(kNN, X, Y_one_hot, cv=kfold))
try:
knn_fuzzy = np.average(cross_val_score(kNN, X, Y_fuzzy, cv=kfold))
except ValueError:
print('kNN: fuzzy classes are not supported')
svc_label = np.average(cross_val_score(svc, X, Y_label, cv=kfold))
try:
svc_one_hot = np.average(cross_val_score(svc, X, Y_one_hot, cv=kfold))
except ValueError:
print('SVC: vector is not supported')
try:
svr_one_hot = np.average(cross_val_score(svr, X, Y_one_hot, cv=kfold))
except ValueError:
print('SVR: vector is not supported')
multi_svr_one_hot = np.average(cross_val_score(multi_svr, X, Y_one_hot, cv=kfold, scoring='neg_mean_absolute_error'))
multi_svr_fuzzy = np.average(cross_val_score(multi_svr, X, Y_fuzzy, cv=kfold, scoring='neg_mean_absolute_error'))
print('sklearn version', sklearn.__version__)
print('Y example: ',
"label: ", Y_label[0],
", one hot: ", Y_one_hot[0, :],
", fuzzy: ", Y_fuzzy[0, :])
print('kNN label: ', knn_label)
print('kNN one hot: ', knn_one_hot)
print('SVC label: ', svc_label)
print('MultiSVR one hot: ', multi_svr_one_hot)
print('MultiSVR fuzzy: ', multi_svr_fuzzy)
Output:
kNN: fuzzy classes are not supported
SVC: vector is not supported
SVR: vector is not supported
sklearn version 0.19.1
Y example: label: B , one hot: [0 1 0] , fuzzy: [0.01 0.98 0.01]
kNN label: 0.321
kNN one hot: 0.254
SVC label: 0.332
MultiSVR one hot: -0.4066160996805417
MultiSVR fuzzy: -0.3970780923514713
Although kNN does not throw an exception for one-hot encoded labels, accuracy 0.254
shows that it does not work correctly with the vector.
Also, Negative Mean Absolute Error is reported for MultiSVR since the task is understood as regression. Score accuracy
can only be used after changing the fuzzy labels and predictions back to a label.
$endgroup$
add a comment |
$begingroup$
For example, for a 3-class classification, we want to train with a label like $A$, which is one-hot encoded as $(1, 0, 0)$, and also with a fuzzy label like $(0.8, 0.2, 0)$. In that case, kNN and SVM of sklearn does not support fuzzy labels.
However, we can use sklearn's MultiOutputRegressor
that extends a one-output Regressor such as Support Vector Regression (SVR) to multiple outputs. It is worth noting that neural networks are a natural fit for this type of label since they readily work with numerical vectors as labels.
Here is a code that goes through different types of labels for kNN, SVC (multi-class SVM), and MultiRegression SVR:
import sklearn
import pandas as pd
from sklearn.svm import SVC, SVR
from sklearn.model_selection import KFold, cross_val_score
from sklearn.neighbors import KNeighborsClassifier
from sklearn.multioutput import MultiOutputRegressor
import numpy as np
N = 1000
split = int(0.8 * N)
folds = 5
seed = 1234
# Data
np.random.seed(seed)
feature_1 = np.random.normal(0, 2, N)
feature_2 = np.random.normal(5, 6, N)
X = np.vstack([feature_1, feature_2]).T
Y_label = np.random.choice(['A', 'B', 'C'], N)
Y_one_hot = pd.get_dummies(Y_label).values
smooth_filter = np.array([0.01, 0.98, 0.01])
Y_fuzzy = np.apply_along_axis(
lambda m: np.convolve(m, smooth_filter, mode='same'), axis=1, arr=Y_one_hot
)
kfold = KFold(n_splits=folds, random_state=seed)
kNN = KNeighborsClassifier(n_neighbors=3)
svc = SVC()
svr = SVR()
multi_svr = MultiOutputRegressor(estimator=SVR())
knn_label = np.average(cross_val_score(kNN, X, Y_label, cv=kfold))
knn_one_hot = np.average(cross_val_score(kNN, X, Y_one_hot, cv=kfold))
try:
knn_fuzzy = np.average(cross_val_score(kNN, X, Y_fuzzy, cv=kfold))
except ValueError:
print('kNN: fuzzy classes are not supported')
svc_label = np.average(cross_val_score(svc, X, Y_label, cv=kfold))
try:
svc_one_hot = np.average(cross_val_score(svc, X, Y_one_hot, cv=kfold))
except ValueError:
print('SVC: vector is not supported')
try:
svr_one_hot = np.average(cross_val_score(svr, X, Y_one_hot, cv=kfold))
except ValueError:
print('SVR: vector is not supported')
multi_svr_one_hot = np.average(cross_val_score(multi_svr, X, Y_one_hot, cv=kfold, scoring='neg_mean_absolute_error'))
multi_svr_fuzzy = np.average(cross_val_score(multi_svr, X, Y_fuzzy, cv=kfold, scoring='neg_mean_absolute_error'))
print('sklearn version', sklearn.__version__)
print('Y example: ',
"label: ", Y_label[0],
", one hot: ", Y_one_hot[0, :],
", fuzzy: ", Y_fuzzy[0, :])
print('kNN label: ', knn_label)
print('kNN one hot: ', knn_one_hot)
print('SVC label: ', svc_label)
print('MultiSVR one hot: ', multi_svr_one_hot)
print('MultiSVR fuzzy: ', multi_svr_fuzzy)
Output:
kNN: fuzzy classes are not supported
SVC: vector is not supported
SVR: vector is not supported
sklearn version 0.19.1
Y example: label: B , one hot: [0 1 0] , fuzzy: [0.01 0.98 0.01]
kNN label: 0.321
kNN one hot: 0.254
SVC label: 0.332
MultiSVR one hot: -0.4066160996805417
MultiSVR fuzzy: -0.3970780923514713
Although kNN does not throw an exception for one-hot encoded labels, accuracy 0.254
shows that it does not work correctly with the vector.
Also, Negative Mean Absolute Error is reported for MultiSVR since the task is understood as regression. Score accuracy
can only be used after changing the fuzzy labels and predictions back to a label.
$endgroup$
add a comment |
$begingroup$
For example, for a 3-class classification, we want to train with a label like $A$, which is one-hot encoded as $(1, 0, 0)$, and also with a fuzzy label like $(0.8, 0.2, 0)$. In that case, kNN and SVM of sklearn does not support fuzzy labels.
However, we can use sklearn's MultiOutputRegressor
that extends a one-output Regressor such as Support Vector Regression (SVR) to multiple outputs. It is worth noting that neural networks are a natural fit for this type of label since they readily work with numerical vectors as labels.
Here is a code that goes through different types of labels for kNN, SVC (multi-class SVM), and MultiRegression SVR:
import sklearn
import pandas as pd
from sklearn.svm import SVC, SVR
from sklearn.model_selection import KFold, cross_val_score
from sklearn.neighbors import KNeighborsClassifier
from sklearn.multioutput import MultiOutputRegressor
import numpy as np
N = 1000
split = int(0.8 * N)
folds = 5
seed = 1234
# Data
np.random.seed(seed)
feature_1 = np.random.normal(0, 2, N)
feature_2 = np.random.normal(5, 6, N)
X = np.vstack([feature_1, feature_2]).T
Y_label = np.random.choice(['A', 'B', 'C'], N)
Y_one_hot = pd.get_dummies(Y_label).values
smooth_filter = np.array([0.01, 0.98, 0.01])
Y_fuzzy = np.apply_along_axis(
lambda m: np.convolve(m, smooth_filter, mode='same'), axis=1, arr=Y_one_hot
)
kfold = KFold(n_splits=folds, random_state=seed)
kNN = KNeighborsClassifier(n_neighbors=3)
svc = SVC()
svr = SVR()
multi_svr = MultiOutputRegressor(estimator=SVR())
knn_label = np.average(cross_val_score(kNN, X, Y_label, cv=kfold))
knn_one_hot = np.average(cross_val_score(kNN, X, Y_one_hot, cv=kfold))
try:
knn_fuzzy = np.average(cross_val_score(kNN, X, Y_fuzzy, cv=kfold))
except ValueError:
print('kNN: fuzzy classes are not supported')
svc_label = np.average(cross_val_score(svc, X, Y_label, cv=kfold))
try:
svc_one_hot = np.average(cross_val_score(svc, X, Y_one_hot, cv=kfold))
except ValueError:
print('SVC: vector is not supported')
try:
svr_one_hot = np.average(cross_val_score(svr, X, Y_one_hot, cv=kfold))
except ValueError:
print('SVR: vector is not supported')
multi_svr_one_hot = np.average(cross_val_score(multi_svr, X, Y_one_hot, cv=kfold, scoring='neg_mean_absolute_error'))
multi_svr_fuzzy = np.average(cross_val_score(multi_svr, X, Y_fuzzy, cv=kfold, scoring='neg_mean_absolute_error'))
print('sklearn version', sklearn.__version__)
print('Y example: ',
"label: ", Y_label[0],
", one hot: ", Y_one_hot[0, :],
", fuzzy: ", Y_fuzzy[0, :])
print('kNN label: ', knn_label)
print('kNN one hot: ', knn_one_hot)
print('SVC label: ', svc_label)
print('MultiSVR one hot: ', multi_svr_one_hot)
print('MultiSVR fuzzy: ', multi_svr_fuzzy)
Output:
kNN: fuzzy classes are not supported
SVC: vector is not supported
SVR: vector is not supported
sklearn version 0.19.1
Y example: label: B , one hot: [0 1 0] , fuzzy: [0.01 0.98 0.01]
kNN label: 0.321
kNN one hot: 0.254
SVC label: 0.332
MultiSVR one hot: -0.4066160996805417
MultiSVR fuzzy: -0.3970780923514713
Although kNN does not throw an exception for one-hot encoded labels, accuracy 0.254
shows that it does not work correctly with the vector.
Also, Negative Mean Absolute Error is reported for MultiSVR since the task is understood as regression. Score accuracy
can only be used after changing the fuzzy labels and predictions back to a label.
$endgroup$
For example, for a 3-class classification, we want to train with a label like $A$, which is one-hot encoded as $(1, 0, 0)$, and also with a fuzzy label like $(0.8, 0.2, 0)$. In that case, kNN and SVM of sklearn does not support fuzzy labels.
However, we can use sklearn's MultiOutputRegressor
that extends a one-output Regressor such as Support Vector Regression (SVR) to multiple outputs. It is worth noting that neural networks are a natural fit for this type of label since they readily work with numerical vectors as labels.
Here is a code that goes through different types of labels for kNN, SVC (multi-class SVM), and MultiRegression SVR:
import sklearn
import pandas as pd
from sklearn.svm import SVC, SVR
from sklearn.model_selection import KFold, cross_val_score
from sklearn.neighbors import KNeighborsClassifier
from sklearn.multioutput import MultiOutputRegressor
import numpy as np
N = 1000
split = int(0.8 * N)
folds = 5
seed = 1234
# Data
np.random.seed(seed)
feature_1 = np.random.normal(0, 2, N)
feature_2 = np.random.normal(5, 6, N)
X = np.vstack([feature_1, feature_2]).T
Y_label = np.random.choice(['A', 'B', 'C'], N)
Y_one_hot = pd.get_dummies(Y_label).values
smooth_filter = np.array([0.01, 0.98, 0.01])
Y_fuzzy = np.apply_along_axis(
lambda m: np.convolve(m, smooth_filter, mode='same'), axis=1, arr=Y_one_hot
)
kfold = KFold(n_splits=folds, random_state=seed)
kNN = KNeighborsClassifier(n_neighbors=3)
svc = SVC()
svr = SVR()
multi_svr = MultiOutputRegressor(estimator=SVR())
knn_label = np.average(cross_val_score(kNN, X, Y_label, cv=kfold))
knn_one_hot = np.average(cross_val_score(kNN, X, Y_one_hot, cv=kfold))
try:
knn_fuzzy = np.average(cross_val_score(kNN, X, Y_fuzzy, cv=kfold))
except ValueError:
print('kNN: fuzzy classes are not supported')
svc_label = np.average(cross_val_score(svc, X, Y_label, cv=kfold))
try:
svc_one_hot = np.average(cross_val_score(svc, X, Y_one_hot, cv=kfold))
except ValueError:
print('SVC: vector is not supported')
try:
svr_one_hot = np.average(cross_val_score(svr, X, Y_one_hot, cv=kfold))
except ValueError:
print('SVR: vector is not supported')
multi_svr_one_hot = np.average(cross_val_score(multi_svr, X, Y_one_hot, cv=kfold, scoring='neg_mean_absolute_error'))
multi_svr_fuzzy = np.average(cross_val_score(multi_svr, X, Y_fuzzy, cv=kfold, scoring='neg_mean_absolute_error'))
print('sklearn version', sklearn.__version__)
print('Y example: ',
"label: ", Y_label[0],
", one hot: ", Y_one_hot[0, :],
", fuzzy: ", Y_fuzzy[0, :])
print('kNN label: ', knn_label)
print('kNN one hot: ', knn_one_hot)
print('SVC label: ', svc_label)
print('MultiSVR one hot: ', multi_svr_one_hot)
print('MultiSVR fuzzy: ', multi_svr_fuzzy)
Output:
kNN: fuzzy classes are not supported
SVC: vector is not supported
SVR: vector is not supported
sklearn version 0.19.1
Y example: label: B , one hot: [0 1 0] , fuzzy: [0.01 0.98 0.01]
kNN label: 0.321
kNN one hot: 0.254
SVC label: 0.332
MultiSVR one hot: -0.4066160996805417
MultiSVR fuzzy: -0.3970780923514713
Although kNN does not throw an exception for one-hot encoded labels, accuracy 0.254
shows that it does not work correctly with the vector.
Also, Negative Mean Absolute Error is reported for MultiSVR since the task is understood as regression. Score accuracy
can only be used after changing the fuzzy labels and predictions back to a label.
edited Mar 28 at 0:00
answered Mar 27 at 23:55
EsmailianEsmailian
2,621318
2,621318
add a comment |
add a comment |
Thanks for contributing an answer to Data Science Stack Exchange!
- Please be sure to answer the question. Provide details and share your research!
But avoid …
- Asking for help, clarification, or responding to other answers.
- Making statements based on opinion; back them up with references or personal experience.
Use MathJax to format equations. MathJax reference.
To learn more, see our tips on writing great answers.
Sign up or log in
StackExchange.ready(function ()
StackExchange.helpers.onClickDraftSave('#login-link');
);
Sign up using Google
Sign up using Facebook
Sign up using Email and Password
Post as a guest
Required, but never shown
StackExchange.ready(
function ()
StackExchange.openid.initPostLogin('.new-post-login', 'https%3a%2f%2fdatascience.stackexchange.com%2fquestions%2f48111%2fmultilabel-classifcation-in-sklearn-with-soft-fuzzy-labels%23new-answer', 'question_page');
);
Post as a guest
Required, but never shown
Sign up or log in
StackExchange.ready(function ()
StackExchange.helpers.onClickDraftSave('#login-link');
);
Sign up using Google
Sign up using Facebook
Sign up using Email and Password
Post as a guest
Required, but never shown
Sign up or log in
StackExchange.ready(function ()
StackExchange.helpers.onClickDraftSave('#login-link');
);
Sign up using Google
Sign up using Facebook
Sign up using Email and Password
Post as a guest
Required, but never shown
Sign up or log in
StackExchange.ready(function ()
StackExchange.helpers.onClickDraftSave('#login-link');
);
Sign up using Google
Sign up using Facebook
Sign up using Email and Password
Sign up using Google
Sign up using Facebook
Sign up using Email and Password
Post as a guest
Required, but never shown
Required, but never shown
Required, but never shown
Required, but never shown
Required, but never shown
Required, but never shown
Required, but never shown
Required, but never shown
Required, but never shown
XzSKXyz2j