MAE,MSE and MAPE aren't comparable?Why does an SVM model store the support vectors, and not just the separating hyperplane?Working back and forth with DataFrame and nparray in Pipeline transformersHow to iterate and modify rows in a dataframe( convert numerical to categorical)Data Mining - Intent matching and classification of textIs this the correct way to apply a recommender system based on KNN and cosine similarity to predict continuous values?Fill missing values AND normaliseUnderstanding the Shuffle and Split Process in a Neural Network Codehow to transformation of row to column and column to row in python pandas?SVM - why does scaling the parameters w and b result in nothing meaningful?
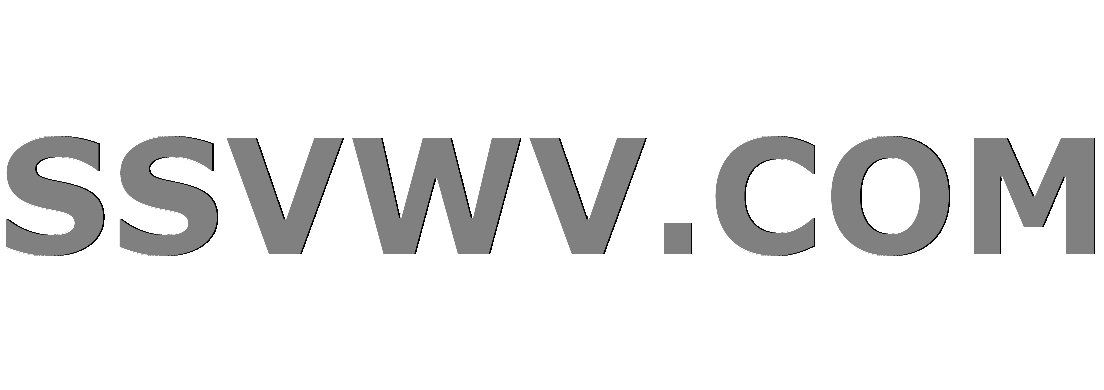
Multi tool use
Problem with TransformedDistribution
Do Legal Documents Require Signing In Standard Pen Colors?
Drawing ramified coverings with tikz
The IT department bottlenecks progress. How should I handle this?
Travelling outside the UK without a passport
Has any country ever had 2 former presidents in jail simultaneously?
Where did Heinlein say "Once you get to Earth orbit, you're halfway to anywhere in the Solar System"?
Did arcade monitors have same pixel aspect ratio as TV sets?
How do I color the graph in datavisualization?
Store Credit Card Information in Password Manager?
Argument list too long when zipping large list of certain files in a folder
Is there a single word describing earning money through any means?
Is it possible to have a strip of cold climate in the middle of a planet?
Calculating Wattage for Resistor in High Frequency Application?
Open a doc from terminal, but not by its name
Why should universal income be universal?
Why does the Sun have different day lengths, but not the gas giants?
Which one is correct as adjective “protruding” or “protruded”?
why `nmap 192.168.1.97` returns less services than `nmap 127.0.0.1`?
What is this called? Old film camera viewer?
What does "landing" mean in this context?
Electoral considerations aside, what are potential benefits, for the US, of policy changes proposed by the tweet recognizing Golan annexation?
I am looking for the correct translation of love for the phrase "in this sign love"
By means of an example, show that P(A) + P(B) = 1 does not mean that B is the complement of A.
MAE,MSE and MAPE aren't comparable?
Why does an SVM model store the support vectors, and not just the separating hyperplane?Working back and forth with DataFrame and nparray in Pipeline transformersHow to iterate and modify rows in a dataframe( convert numerical to categorical)Data Mining - Intent matching and classification of textIs this the correct way to apply a recommender system based on KNN and cosine similarity to predict continuous values?Fill missing values AND normaliseUnderstanding the Shuffle and Split Process in a Neural Network Codehow to transformation of row to column and column to row in python pandas?SVM - why does scaling the parameters w and b result in nothing meaningful?
$begingroup$
I'm a newbie in data science. I'm working on a regression problem. I'm getting 2.5 MAPE. 400 MAE 437000 MSE. As my MAPE is quite low but why I'm getting high MSE and MAE? This is the link to my data
from sklearn.metrics import mean_absolute_error
from sklearn.metrics import mean_squared_error
from sklearn.preprocessing import Normalizer
import matplotlib.pyplot as plt
def mean_absolute_percentage_error(y_true, y_pred):
y_true, y_pred = np.array(y_true), np.array(y_pred)
return np.mean(np.abs((y_true - y_pred) / y_true)) * 100
import pandas as pd
from sklearn import preprocessing
features=pd.read_csv('selectedData.csv')
import numpy as np
from scipy import stats
print(features.shape)
features=features[(np.abs(stats.zscore(features)) < 3).all(axis=1)]
target = features['SYSLoad']
features= features.drop('SYSLoad', axis = 1)
names=list(features)
for i in names:
x=features[[i]].values.astype(float)
min_max_scaler = preprocessing.MinMaxScaler()
x_scaled = min_max_scaler.fit_transform(x)
features[i]=x_scaled
Selecting the target Variable which want to predict and for which we are finding feature imps
import numpy as np
print(features.shape)
print(features.describe())
from sklearn.model_selection import train_test_split
train_input, test_input, train_target, test_target =
train_test_split(features, target, test_size = 0.25, random_state = 42)
trans=Normalizer().fit(train_input);
train_input=Normalizer().fit_transform(train_input);
test_input=trans.fit_transform(test_input);
n=test_target.values;
test_targ=pd.DataFrame(n);
from sklearn.svm import SVR
svr_rbf = SVR(kernel='poly', C=10, epsilon=10,gamma=10)
y_rbf = svr_rbf.fit(train_input, train_target);
predicted=y_rbf.predict(test_input);
plt.figure
plt.xlim(300,500);
print('Total Days For training',len(train_input)); print('Total Days For
Testing',len(test_input))
plt.ylabel('Load(MW) Prediction 3 '); plt.xlabel('Days');
plt.plot(test_targ,'-b',label='Actual'); plt.plot(predicted,'-r',label='POLY
kernel ');
plt.gca().legend(('Actual','RBF'))
plt.title('SVM')
plt.show();
test_target=np.array(test_target)
print(test_target)
MAPE=mean_absolute_percentage_error(test_target,predicted);
print(MAPE);
mae=mean_absolute_error(test_target,predicted)
mse=mean_squared_error(test_target, predicted)
print(mae);
print(mse);
print(test_target);
print(predicted);
data-mining pandas svm numpy
$endgroup$
add a comment |
$begingroup$
I'm a newbie in data science. I'm working on a regression problem. I'm getting 2.5 MAPE. 400 MAE 437000 MSE. As my MAPE is quite low but why I'm getting high MSE and MAE? This is the link to my data
from sklearn.metrics import mean_absolute_error
from sklearn.metrics import mean_squared_error
from sklearn.preprocessing import Normalizer
import matplotlib.pyplot as plt
def mean_absolute_percentage_error(y_true, y_pred):
y_true, y_pred = np.array(y_true), np.array(y_pred)
return np.mean(np.abs((y_true - y_pred) / y_true)) * 100
import pandas as pd
from sklearn import preprocessing
features=pd.read_csv('selectedData.csv')
import numpy as np
from scipy import stats
print(features.shape)
features=features[(np.abs(stats.zscore(features)) < 3).all(axis=1)]
target = features['SYSLoad']
features= features.drop('SYSLoad', axis = 1)
names=list(features)
for i in names:
x=features[[i]].values.astype(float)
min_max_scaler = preprocessing.MinMaxScaler()
x_scaled = min_max_scaler.fit_transform(x)
features[i]=x_scaled
Selecting the target Variable which want to predict and for which we are finding feature imps
import numpy as np
print(features.shape)
print(features.describe())
from sklearn.model_selection import train_test_split
train_input, test_input, train_target, test_target =
train_test_split(features, target, test_size = 0.25, random_state = 42)
trans=Normalizer().fit(train_input);
train_input=Normalizer().fit_transform(train_input);
test_input=trans.fit_transform(test_input);
n=test_target.values;
test_targ=pd.DataFrame(n);
from sklearn.svm import SVR
svr_rbf = SVR(kernel='poly', C=10, epsilon=10,gamma=10)
y_rbf = svr_rbf.fit(train_input, train_target);
predicted=y_rbf.predict(test_input);
plt.figure
plt.xlim(300,500);
print('Total Days For training',len(train_input)); print('Total Days For
Testing',len(test_input))
plt.ylabel('Load(MW) Prediction 3 '); plt.xlabel('Days');
plt.plot(test_targ,'-b',label='Actual'); plt.plot(predicted,'-r',label='POLY
kernel ');
plt.gca().legend(('Actual','RBF'))
plt.title('SVM')
plt.show();
test_target=np.array(test_target)
print(test_target)
MAPE=mean_absolute_percentage_error(test_target,predicted);
print(MAPE);
mae=mean_absolute_error(test_target,predicted)
mse=mean_squared_error(test_target, predicted)
print(mae);
print(mse);
print(test_target);
print(predicted);
data-mining pandas svm numpy
$endgroup$
add a comment |
$begingroup$
I'm a newbie in data science. I'm working on a regression problem. I'm getting 2.5 MAPE. 400 MAE 437000 MSE. As my MAPE is quite low but why I'm getting high MSE and MAE? This is the link to my data
from sklearn.metrics import mean_absolute_error
from sklearn.metrics import mean_squared_error
from sklearn.preprocessing import Normalizer
import matplotlib.pyplot as plt
def mean_absolute_percentage_error(y_true, y_pred):
y_true, y_pred = np.array(y_true), np.array(y_pred)
return np.mean(np.abs((y_true - y_pred) / y_true)) * 100
import pandas as pd
from sklearn import preprocessing
features=pd.read_csv('selectedData.csv')
import numpy as np
from scipy import stats
print(features.shape)
features=features[(np.abs(stats.zscore(features)) < 3).all(axis=1)]
target = features['SYSLoad']
features= features.drop('SYSLoad', axis = 1)
names=list(features)
for i in names:
x=features[[i]].values.astype(float)
min_max_scaler = preprocessing.MinMaxScaler()
x_scaled = min_max_scaler.fit_transform(x)
features[i]=x_scaled
Selecting the target Variable which want to predict and for which we are finding feature imps
import numpy as np
print(features.shape)
print(features.describe())
from sklearn.model_selection import train_test_split
train_input, test_input, train_target, test_target =
train_test_split(features, target, test_size = 0.25, random_state = 42)
trans=Normalizer().fit(train_input);
train_input=Normalizer().fit_transform(train_input);
test_input=trans.fit_transform(test_input);
n=test_target.values;
test_targ=pd.DataFrame(n);
from sklearn.svm import SVR
svr_rbf = SVR(kernel='poly', C=10, epsilon=10,gamma=10)
y_rbf = svr_rbf.fit(train_input, train_target);
predicted=y_rbf.predict(test_input);
plt.figure
plt.xlim(300,500);
print('Total Days For training',len(train_input)); print('Total Days For
Testing',len(test_input))
plt.ylabel('Load(MW) Prediction 3 '); plt.xlabel('Days');
plt.plot(test_targ,'-b',label='Actual'); plt.plot(predicted,'-r',label='POLY
kernel ');
plt.gca().legend(('Actual','RBF'))
plt.title('SVM')
plt.show();
test_target=np.array(test_target)
print(test_target)
MAPE=mean_absolute_percentage_error(test_target,predicted);
print(MAPE);
mae=mean_absolute_error(test_target,predicted)
mse=mean_squared_error(test_target, predicted)
print(mae);
print(mse);
print(test_target);
print(predicted);
data-mining pandas svm numpy
$endgroup$
I'm a newbie in data science. I'm working on a regression problem. I'm getting 2.5 MAPE. 400 MAE 437000 MSE. As my MAPE is quite low but why I'm getting high MSE and MAE? This is the link to my data
from sklearn.metrics import mean_absolute_error
from sklearn.metrics import mean_squared_error
from sklearn.preprocessing import Normalizer
import matplotlib.pyplot as plt
def mean_absolute_percentage_error(y_true, y_pred):
y_true, y_pred = np.array(y_true), np.array(y_pred)
return np.mean(np.abs((y_true - y_pred) / y_true)) * 100
import pandas as pd
from sklearn import preprocessing
features=pd.read_csv('selectedData.csv')
import numpy as np
from scipy import stats
print(features.shape)
features=features[(np.abs(stats.zscore(features)) < 3).all(axis=1)]
target = features['SYSLoad']
features= features.drop('SYSLoad', axis = 1)
names=list(features)
for i in names:
x=features[[i]].values.astype(float)
min_max_scaler = preprocessing.MinMaxScaler()
x_scaled = min_max_scaler.fit_transform(x)
features[i]=x_scaled
Selecting the target Variable which want to predict and for which we are finding feature imps
import numpy as np
print(features.shape)
print(features.describe())
from sklearn.model_selection import train_test_split
train_input, test_input, train_target, test_target =
train_test_split(features, target, test_size = 0.25, random_state = 42)
trans=Normalizer().fit(train_input);
train_input=Normalizer().fit_transform(train_input);
test_input=trans.fit_transform(test_input);
n=test_target.values;
test_targ=pd.DataFrame(n);
from sklearn.svm import SVR
svr_rbf = SVR(kernel='poly', C=10, epsilon=10,gamma=10)
y_rbf = svr_rbf.fit(train_input, train_target);
predicted=y_rbf.predict(test_input);
plt.figure
plt.xlim(300,500);
print('Total Days For training',len(train_input)); print('Total Days For
Testing',len(test_input))
plt.ylabel('Load(MW) Prediction 3 '); plt.xlabel('Days');
plt.plot(test_targ,'-b',label='Actual'); plt.plot(predicted,'-r',label='POLY
kernel ');
plt.gca().legend(('Actual','RBF'))
plt.title('SVM')
plt.show();
test_target=np.array(test_target)
print(test_target)
MAPE=mean_absolute_percentage_error(test_target,predicted);
print(MAPE);
mae=mean_absolute_error(test_target,predicted)
mse=mean_squared_error(test_target, predicted)
print(mae);
print(mse);
print(test_target);
print(predicted);
data-mining pandas svm numpy
data-mining pandas svm numpy
edited Feb 19 at 17:40
ebrahimi
74221021
74221021
asked Feb 19 at 15:26


imtiaz ul Hassanimtiaz ul Hassan
204
204
add a comment |
add a comment |
2 Answers
2
active
oldest
votes
$begingroup$
I'll be honest, I haven't thoroughly checked your code. However, I can see that the range of values of your dataset is approx [0,12000]. As an engineer, I see that:
- sqrt(MSE) = sqrt(437000) = 661 units.
- MAE = 400 units.
- MAPE = 2.5 which means that MAE can be up to 0.025*12000= 250 units.
All three cases show similar magnitude of error, so I wouldn't say that "MAPE is quite low but you're getting high mse and MAE".
Those 3 values explain the results from similar yet different perspectives. Keep in mind, if the values were all the same, there would have been no need for all 3 of those metrics to exist :)
$endgroup$
$begingroup$
Thank you and what does r-square metrics shows?
$endgroup$
– imtiaz ul Hassan
Feb 19 at 16:25
$begingroup$
I believe Wikipedia has a very nice explanation :) en.wikipedia.org/wiki/Coefficient_of_determination
$endgroup$
– pcko1
Feb 19 at 21:49
$begingroup$
@pcko1 thank you for your answer. Is it possible to elaborate? I didn't understand what you've meat for 1 to 3. For instance, what do you mean by MAE = 400 units?
$endgroup$
– Media
2 days ago
$begingroup$
well MAE stands for Mean Absolute Error and OP mentioned that he gets "2.5 MAPE, 400 MAE, 437000 MSE". I just tried to make a back-of-the-envelope evaluation of the magnitute of those values, which seem reasonable given his dataset :) as for "units", this refers to the physical units of the problem, whatever they might be
$endgroup$
– pcko1
2 days ago
1
$begingroup$
Thank you :) nice perspective.
$endgroup$
– Media
2 days ago
add a comment |
$begingroup$
You are stating something that is by definition the case. A Mean Absolute Percentage Error (MAPE) is typically a small number expressed in percentage, hopefully in the single digit. Meanwhile the Mean Squared Error (MSE) and Mean Absolute Error) are expressed in square of units and units respectively. If your units are > 1, the MSE could get easily very large on a relative basis compared to the MAE. And, the MAE could also be a lot larger than the MAPE. This is just like saying a nominal number is a lot larger than its log or natural logs. Your three error measures are measured on pretty different scale.
They just bring some perspective to how well your model fit your data. Depending on the circumstances or context one error measure may be more relevant than the other.
New contributor
Sympa is a new contributor to this site. Take care in asking for clarification, commenting, and answering.
Check out our Code of Conduct.
$endgroup$
add a comment |
Your Answer
StackExchange.ifUsing("editor", function ()
return StackExchange.using("mathjaxEditing", function ()
StackExchange.MarkdownEditor.creationCallbacks.add(function (editor, postfix)
StackExchange.mathjaxEditing.prepareWmdForMathJax(editor, postfix, [["$", "$"], ["\\(","\\)"]]);
);
);
, "mathjax-editing");
StackExchange.ready(function()
var channelOptions =
tags: "".split(" "),
id: "557"
;
initTagRenderer("".split(" "), "".split(" "), channelOptions);
StackExchange.using("externalEditor", function()
// Have to fire editor after snippets, if snippets enabled
if (StackExchange.settings.snippets.snippetsEnabled)
StackExchange.using("snippets", function()
createEditor();
);
else
createEditor();
);
function createEditor()
StackExchange.prepareEditor(
heartbeatType: 'answer',
autoActivateHeartbeat: false,
convertImagesToLinks: false,
noModals: true,
showLowRepImageUploadWarning: true,
reputationToPostImages: null,
bindNavPrevention: true,
postfix: "",
imageUploader:
brandingHtml: "Powered by u003ca class="icon-imgur-white" href="https://imgur.com/"u003eu003c/au003e",
contentPolicyHtml: "User contributions licensed under u003ca href="https://creativecommons.org/licenses/by-sa/3.0/"u003ecc by-sa 3.0 with attribution requiredu003c/au003e u003ca href="https://stackoverflow.com/legal/content-policy"u003e(content policy)u003c/au003e",
allowUrls: true
,
onDemand: true,
discardSelector: ".discard-answer"
,immediatelyShowMarkdownHelp:true
);
);
Sign up or log in
StackExchange.ready(function ()
StackExchange.helpers.onClickDraftSave('#login-link');
);
Sign up using Google
Sign up using Facebook
Sign up using Email and Password
Post as a guest
Required, but never shown
StackExchange.ready(
function ()
StackExchange.openid.initPostLogin('.new-post-login', 'https%3a%2f%2fdatascience.stackexchange.com%2fquestions%2f45821%2fmae-mse-and-mape-arent-comparable%23new-answer', 'question_page');
);
Post as a guest
Required, but never shown
2 Answers
2
active
oldest
votes
2 Answers
2
active
oldest
votes
active
oldest
votes
active
oldest
votes
$begingroup$
I'll be honest, I haven't thoroughly checked your code. However, I can see that the range of values of your dataset is approx [0,12000]. As an engineer, I see that:
- sqrt(MSE) = sqrt(437000) = 661 units.
- MAE = 400 units.
- MAPE = 2.5 which means that MAE can be up to 0.025*12000= 250 units.
All three cases show similar magnitude of error, so I wouldn't say that "MAPE is quite low but you're getting high mse and MAE".
Those 3 values explain the results from similar yet different perspectives. Keep in mind, if the values were all the same, there would have been no need for all 3 of those metrics to exist :)
$endgroup$
$begingroup$
Thank you and what does r-square metrics shows?
$endgroup$
– imtiaz ul Hassan
Feb 19 at 16:25
$begingroup$
I believe Wikipedia has a very nice explanation :) en.wikipedia.org/wiki/Coefficient_of_determination
$endgroup$
– pcko1
Feb 19 at 21:49
$begingroup$
@pcko1 thank you for your answer. Is it possible to elaborate? I didn't understand what you've meat for 1 to 3. For instance, what do you mean by MAE = 400 units?
$endgroup$
– Media
2 days ago
$begingroup$
well MAE stands for Mean Absolute Error and OP mentioned that he gets "2.5 MAPE, 400 MAE, 437000 MSE". I just tried to make a back-of-the-envelope evaluation of the magnitute of those values, which seem reasonable given his dataset :) as for "units", this refers to the physical units of the problem, whatever they might be
$endgroup$
– pcko1
2 days ago
1
$begingroup$
Thank you :) nice perspective.
$endgroup$
– Media
2 days ago
add a comment |
$begingroup$
I'll be honest, I haven't thoroughly checked your code. However, I can see that the range of values of your dataset is approx [0,12000]. As an engineer, I see that:
- sqrt(MSE) = sqrt(437000) = 661 units.
- MAE = 400 units.
- MAPE = 2.5 which means that MAE can be up to 0.025*12000= 250 units.
All three cases show similar magnitude of error, so I wouldn't say that "MAPE is quite low but you're getting high mse and MAE".
Those 3 values explain the results from similar yet different perspectives. Keep in mind, if the values were all the same, there would have been no need for all 3 of those metrics to exist :)
$endgroup$
$begingroup$
Thank you and what does r-square metrics shows?
$endgroup$
– imtiaz ul Hassan
Feb 19 at 16:25
$begingroup$
I believe Wikipedia has a very nice explanation :) en.wikipedia.org/wiki/Coefficient_of_determination
$endgroup$
– pcko1
Feb 19 at 21:49
$begingroup$
@pcko1 thank you for your answer. Is it possible to elaborate? I didn't understand what you've meat for 1 to 3. For instance, what do you mean by MAE = 400 units?
$endgroup$
– Media
2 days ago
$begingroup$
well MAE stands for Mean Absolute Error and OP mentioned that he gets "2.5 MAPE, 400 MAE, 437000 MSE". I just tried to make a back-of-the-envelope evaluation of the magnitute of those values, which seem reasonable given his dataset :) as for "units", this refers to the physical units of the problem, whatever they might be
$endgroup$
– pcko1
2 days ago
1
$begingroup$
Thank you :) nice perspective.
$endgroup$
– Media
2 days ago
add a comment |
$begingroup$
I'll be honest, I haven't thoroughly checked your code. However, I can see that the range of values of your dataset is approx [0,12000]. As an engineer, I see that:
- sqrt(MSE) = sqrt(437000) = 661 units.
- MAE = 400 units.
- MAPE = 2.5 which means that MAE can be up to 0.025*12000= 250 units.
All three cases show similar magnitude of error, so I wouldn't say that "MAPE is quite low but you're getting high mse and MAE".
Those 3 values explain the results from similar yet different perspectives. Keep in mind, if the values were all the same, there would have been no need for all 3 of those metrics to exist :)
$endgroup$
I'll be honest, I haven't thoroughly checked your code. However, I can see that the range of values of your dataset is approx [0,12000]. As an engineer, I see that:
- sqrt(MSE) = sqrt(437000) = 661 units.
- MAE = 400 units.
- MAPE = 2.5 which means that MAE can be up to 0.025*12000= 250 units.
All three cases show similar magnitude of error, so I wouldn't say that "MAPE is quite low but you're getting high mse and MAE".
Those 3 values explain the results from similar yet different perspectives. Keep in mind, if the values were all the same, there would have been no need for all 3 of those metrics to exist :)
edited Feb 19 at 21:57
answered Feb 19 at 15:58


pcko1pcko1
1,581417
1,581417
$begingroup$
Thank you and what does r-square metrics shows?
$endgroup$
– imtiaz ul Hassan
Feb 19 at 16:25
$begingroup$
I believe Wikipedia has a very nice explanation :) en.wikipedia.org/wiki/Coefficient_of_determination
$endgroup$
– pcko1
Feb 19 at 21:49
$begingroup$
@pcko1 thank you for your answer. Is it possible to elaborate? I didn't understand what you've meat for 1 to 3. For instance, what do you mean by MAE = 400 units?
$endgroup$
– Media
2 days ago
$begingroup$
well MAE stands for Mean Absolute Error and OP mentioned that he gets "2.5 MAPE, 400 MAE, 437000 MSE". I just tried to make a back-of-the-envelope evaluation of the magnitute of those values, which seem reasonable given his dataset :) as for "units", this refers to the physical units of the problem, whatever they might be
$endgroup$
– pcko1
2 days ago
1
$begingroup$
Thank you :) nice perspective.
$endgroup$
– Media
2 days ago
add a comment |
$begingroup$
Thank you and what does r-square metrics shows?
$endgroup$
– imtiaz ul Hassan
Feb 19 at 16:25
$begingroup$
I believe Wikipedia has a very nice explanation :) en.wikipedia.org/wiki/Coefficient_of_determination
$endgroup$
– pcko1
Feb 19 at 21:49
$begingroup$
@pcko1 thank you for your answer. Is it possible to elaborate? I didn't understand what you've meat for 1 to 3. For instance, what do you mean by MAE = 400 units?
$endgroup$
– Media
2 days ago
$begingroup$
well MAE stands for Mean Absolute Error and OP mentioned that he gets "2.5 MAPE, 400 MAE, 437000 MSE". I just tried to make a back-of-the-envelope evaluation of the magnitute of those values, which seem reasonable given his dataset :) as for "units", this refers to the physical units of the problem, whatever they might be
$endgroup$
– pcko1
2 days ago
1
$begingroup$
Thank you :) nice perspective.
$endgroup$
– Media
2 days ago
$begingroup$
Thank you and what does r-square metrics shows?
$endgroup$
– imtiaz ul Hassan
Feb 19 at 16:25
$begingroup$
Thank you and what does r-square metrics shows?
$endgroup$
– imtiaz ul Hassan
Feb 19 at 16:25
$begingroup$
I believe Wikipedia has a very nice explanation :) en.wikipedia.org/wiki/Coefficient_of_determination
$endgroup$
– pcko1
Feb 19 at 21:49
$begingroup$
I believe Wikipedia has a very nice explanation :) en.wikipedia.org/wiki/Coefficient_of_determination
$endgroup$
– pcko1
Feb 19 at 21:49
$begingroup$
@pcko1 thank you for your answer. Is it possible to elaborate? I didn't understand what you've meat for 1 to 3. For instance, what do you mean by MAE = 400 units?
$endgroup$
– Media
2 days ago
$begingroup$
@pcko1 thank you for your answer. Is it possible to elaborate? I didn't understand what you've meat for 1 to 3. For instance, what do you mean by MAE = 400 units?
$endgroup$
– Media
2 days ago
$begingroup$
well MAE stands for Mean Absolute Error and OP mentioned that he gets "2.5 MAPE, 400 MAE, 437000 MSE". I just tried to make a back-of-the-envelope evaluation of the magnitute of those values, which seem reasonable given his dataset :) as for "units", this refers to the physical units of the problem, whatever they might be
$endgroup$
– pcko1
2 days ago
$begingroup$
well MAE stands for Mean Absolute Error and OP mentioned that he gets "2.5 MAPE, 400 MAE, 437000 MSE". I just tried to make a back-of-the-envelope evaluation of the magnitute of those values, which seem reasonable given his dataset :) as for "units", this refers to the physical units of the problem, whatever they might be
$endgroup$
– pcko1
2 days ago
1
1
$begingroup$
Thank you :) nice perspective.
$endgroup$
– Media
2 days ago
$begingroup$
Thank you :) nice perspective.
$endgroup$
– Media
2 days ago
add a comment |
$begingroup$
You are stating something that is by definition the case. A Mean Absolute Percentage Error (MAPE) is typically a small number expressed in percentage, hopefully in the single digit. Meanwhile the Mean Squared Error (MSE) and Mean Absolute Error) are expressed in square of units and units respectively. If your units are > 1, the MSE could get easily very large on a relative basis compared to the MAE. And, the MAE could also be a lot larger than the MAPE. This is just like saying a nominal number is a lot larger than its log or natural logs. Your three error measures are measured on pretty different scale.
They just bring some perspective to how well your model fit your data. Depending on the circumstances or context one error measure may be more relevant than the other.
New contributor
Sympa is a new contributor to this site. Take care in asking for clarification, commenting, and answering.
Check out our Code of Conduct.
$endgroup$
add a comment |
$begingroup$
You are stating something that is by definition the case. A Mean Absolute Percentage Error (MAPE) is typically a small number expressed in percentage, hopefully in the single digit. Meanwhile the Mean Squared Error (MSE) and Mean Absolute Error) are expressed in square of units and units respectively. If your units are > 1, the MSE could get easily very large on a relative basis compared to the MAE. And, the MAE could also be a lot larger than the MAPE. This is just like saying a nominal number is a lot larger than its log or natural logs. Your three error measures are measured on pretty different scale.
They just bring some perspective to how well your model fit your data. Depending on the circumstances or context one error measure may be more relevant than the other.
New contributor
Sympa is a new contributor to this site. Take care in asking for clarification, commenting, and answering.
Check out our Code of Conduct.
$endgroup$
add a comment |
$begingroup$
You are stating something that is by definition the case. A Mean Absolute Percentage Error (MAPE) is typically a small number expressed in percentage, hopefully in the single digit. Meanwhile the Mean Squared Error (MSE) and Mean Absolute Error) are expressed in square of units and units respectively. If your units are > 1, the MSE could get easily very large on a relative basis compared to the MAE. And, the MAE could also be a lot larger than the MAPE. This is just like saying a nominal number is a lot larger than its log or natural logs. Your three error measures are measured on pretty different scale.
They just bring some perspective to how well your model fit your data. Depending on the circumstances or context one error measure may be more relevant than the other.
New contributor
Sympa is a new contributor to this site. Take care in asking for clarification, commenting, and answering.
Check out our Code of Conduct.
$endgroup$
You are stating something that is by definition the case. A Mean Absolute Percentage Error (MAPE) is typically a small number expressed in percentage, hopefully in the single digit. Meanwhile the Mean Squared Error (MSE) and Mean Absolute Error) are expressed in square of units and units respectively. If your units are > 1, the MSE could get easily very large on a relative basis compared to the MAE. And, the MAE could also be a lot larger than the MAPE. This is just like saying a nominal number is a lot larger than its log or natural logs. Your three error measures are measured on pretty different scale.
They just bring some perspective to how well your model fit your data. Depending on the circumstances or context one error measure may be more relevant than the other.
New contributor
Sympa is a new contributor to this site. Take care in asking for clarification, commenting, and answering.
Check out our Code of Conduct.
New contributor
Sympa is a new contributor to this site. Take care in asking for clarification, commenting, and answering.
Check out our Code of Conduct.
answered 2 days ago
SympaSympa
1164
1164
New contributor
Sympa is a new contributor to this site. Take care in asking for clarification, commenting, and answering.
Check out our Code of Conduct.
New contributor
Sympa is a new contributor to this site. Take care in asking for clarification, commenting, and answering.
Check out our Code of Conduct.
Sympa is a new contributor to this site. Take care in asking for clarification, commenting, and answering.
Check out our Code of Conduct.
add a comment |
add a comment |
Thanks for contributing an answer to Data Science Stack Exchange!
- Please be sure to answer the question. Provide details and share your research!
But avoid …
- Asking for help, clarification, or responding to other answers.
- Making statements based on opinion; back them up with references or personal experience.
Use MathJax to format equations. MathJax reference.
To learn more, see our tips on writing great answers.
Sign up or log in
StackExchange.ready(function ()
StackExchange.helpers.onClickDraftSave('#login-link');
);
Sign up using Google
Sign up using Facebook
Sign up using Email and Password
Post as a guest
Required, but never shown
StackExchange.ready(
function ()
StackExchange.openid.initPostLogin('.new-post-login', 'https%3a%2f%2fdatascience.stackexchange.com%2fquestions%2f45821%2fmae-mse-and-mape-arent-comparable%23new-answer', 'question_page');
);
Post as a guest
Required, but never shown
Sign up or log in
StackExchange.ready(function ()
StackExchange.helpers.onClickDraftSave('#login-link');
);
Sign up using Google
Sign up using Facebook
Sign up using Email and Password
Post as a guest
Required, but never shown
Sign up or log in
StackExchange.ready(function ()
StackExchange.helpers.onClickDraftSave('#login-link');
);
Sign up using Google
Sign up using Facebook
Sign up using Email and Password
Post as a guest
Required, but never shown
Sign up or log in
StackExchange.ready(function ()
StackExchange.helpers.onClickDraftSave('#login-link');
);
Sign up using Google
Sign up using Facebook
Sign up using Email and Password
Sign up using Google
Sign up using Facebook
Sign up using Email and Password
Post as a guest
Required, but never shown
Required, but never shown
Required, but never shown
Required, but never shown
Required, but never shown
Required, but never shown
Required, but never shown
Required, but never shown
Required, but never shown
iZcU9d PTolHo4Yv8BAMQ SfL,grnDA,QH2uPqrmFDd2f