Using cross-validation technique for a CNN model? The Next CEO of Stack Overflow2019 Community Moderator ElectionValidation vs. test vs. training accuracy. Which one should I compare for claiming overfit?Convolutional Neural Network not learning EEG dataConsistently inconsistent cross-validation results that are wildly different from original model accuracyReporting test result for cross-validation with Neural NetworkDecision tree classifier: possible overfittingTaking average of multiple neural networks?Interpreting confusion matrix and validation results in convolutional networksDifficulty in choosing Hyperparameters for my CNNsklearn cross_validate without test/train splitOversampling before Cross-Validation, is it a problem?Stop CNN model at high accuracy and low loss rate?
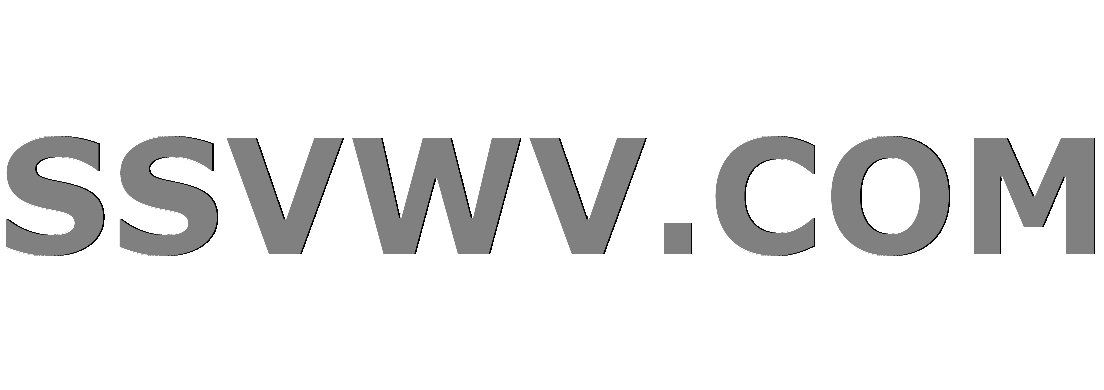
Multi tool use
Inappropriate reference requests from Journal reviewers
Return the Closest Prime Number
How to start emacs in "nothing" mode (`fundamental-mode`)
I believe this to be a fraud - hired, then asked to cash check and send cash as Bitcoin
Would this house-rule that treats advantage as a +1 to the roll instead (and disadvantage as -1) and allows them to stack be balanced?
If/When UK leaves the EU, can a future goverment do a referendum to join EU
Trouble understanding the speech of overseas colleagues
How long to clear the 'suck zone' of a turbofan after start is initiated?
How do I go from 300 unfinished/half written blog posts, to published posts?
How should I support this large drywall patch?
Why is there a PLL in CPU?
Help understanding this unsettling image of Titan, Epimetheus, and Saturn's rings?
Clustering points and summing up attributes per cluster in QGIS
How to get regions to plot as graphics
Can a caster that cast Polymorph on themselves stop concentrating at any point even if their Int is low?
At which OSI layer a user-generated data resides?
Which organization defines CJK Unified Ideographs?
Why doesn't a table tennis ball float on a surface of steel balls? How do we calculate buoyancy here?
Is HostGator storing my password in plaintext?
On model categories where every object is bifibrant
If the heap is initialized for security, then why is the stack uninitialized?
Why do airplanes bank sharply to the right after air-to-air refueling?
How to write papers efficiently when English isn't my first language?
How to count occurrences of text in a file?
Using cross-validation technique for a CNN model?
The Next CEO of Stack Overflow2019 Community Moderator ElectionValidation vs. test vs. training accuracy. Which one should I compare for claiming overfit?Convolutional Neural Network not learning EEG dataConsistently inconsistent cross-validation results that are wildly different from original model accuracyReporting test result for cross-validation with Neural NetworkDecision tree classifier: possible overfittingTaking average of multiple neural networks?Interpreting confusion matrix and validation results in convolutional networksDifficulty in choosing Hyperparameters for my CNNsklearn cross_validate without test/train splitOversampling before Cross-Validation, is it a problem?Stop CNN model at high accuracy and low loss rate?
$begingroup$
I am working on the CNN model, as always I use batches with epochs to train my model, for my model, when it completed training and validation, finally I use a test set to measure the model performance and generate confusion matrix, now I want to use cross-validation to train my model, I can implement it but there are some questions in my mind, my questions are:
1- why most CNN models not using cross-validation technique?
2- if I use cross-validation how can I generate confusion matrix? can I split dataset to train/test then do cross-validation on train set as train/validation (i.e. doing cross-validation as train/validation except for the usual train/test) and at last use test set the same way? or how?
python deep-learning
$endgroup$
add a comment |
$begingroup$
I am working on the CNN model, as always I use batches with epochs to train my model, for my model, when it completed training and validation, finally I use a test set to measure the model performance and generate confusion matrix, now I want to use cross-validation to train my model, I can implement it but there are some questions in my mind, my questions are:
1- why most CNN models not using cross-validation technique?
2- if I use cross-validation how can I generate confusion matrix? can I split dataset to train/test then do cross-validation on train set as train/validation (i.e. doing cross-validation as train/validation except for the usual train/test) and at last use test set the same way? or how?
python deep-learning
$endgroup$
add a comment |
$begingroup$
I am working on the CNN model, as always I use batches with epochs to train my model, for my model, when it completed training and validation, finally I use a test set to measure the model performance and generate confusion matrix, now I want to use cross-validation to train my model, I can implement it but there are some questions in my mind, my questions are:
1- why most CNN models not using cross-validation technique?
2- if I use cross-validation how can I generate confusion matrix? can I split dataset to train/test then do cross-validation on train set as train/validation (i.e. doing cross-validation as train/validation except for the usual train/test) and at last use test set the same way? or how?
python deep-learning
$endgroup$
I am working on the CNN model, as always I use batches with epochs to train my model, for my model, when it completed training and validation, finally I use a test set to measure the model performance and generate confusion matrix, now I want to use cross-validation to train my model, I can implement it but there are some questions in my mind, my questions are:
1- why most CNN models not using cross-validation technique?
2- if I use cross-validation how can I generate confusion matrix? can I split dataset to train/test then do cross-validation on train set as train/validation (i.e. doing cross-validation as train/validation except for the usual train/test) and at last use test set the same way? or how?
python deep-learning
python deep-learning
asked Mar 22 at 15:40


honar.cshonar.cs
14113
14113
add a comment |
add a comment |
2 Answers
2
active
oldest
votes
$begingroup$
Question 1: Why do most CNN models not apply the cross-validation technique?
$k$-fold cross-validation is often used for simple models with few parameters, models with simple hyperparameters and additionally the models are easy to optimize. Typical examples are linear regression, logistic regression, small neural networks and support vector machines.
For a convolutional neural network with many parameters (e.g. more than one million) we just have too many possible changes in the architecture. What you can do is to do some experiments with the learning rate, batch size, dropout (amount and position) and batch normalization (position). Training a convolutional neural network with a huge dataset takes quite a long time. Doing hyperparameter optimization would just be total overkill. Often in papers, they try to improve the results of other research papers. It is not the goal to get better results by improving the chosen hyperparameters but rather to come up with new ideas to solve the given task but with better accuracy or less computational effort.
Question 2: If I use cross-validation how can I generate confusion
matrix? can I split dataset to train/test then do cross-validation on
train set as train/validation (i.e. doing cross-validation as
train/validation except for the usual train/test) and at last use test
set the same way? or how?
In order to do $k$-fold cross validation you will need to split your initial data set into two parts. One dataset for doing the hyperparameter optimization and one for the final validation. Then we take the dataset for the hyperparameter optimization and split it into $k$ (hopefully) equally sized data sets $mathcalD_1,mathcalD_2,ldots,mathcalD_k$. For the sake of clarity let us set $k=3$. Then for each possible hyperparameter combination that we want to test we use $mathcalD_1$ and $mathcalD_2$ to fit our model and we use $mathcalD_3$ to validate our model. Then we do the same with $mathcalD_2$ and $mathcalD_3$ and use $mathcalD_1$ for validation. Then we do the same with $mathcalD_1$ and $mathcalD_3$ and use $mathcalD_2$ for validation. We will get $3$ confusion matrices for every possible hyperparameter configuration. In order to derive a metric from these three results, we take the mean of these confusion matrices. Then we can scan through all averaged confusion matrices so select the hyperparameter configuration that was the best (you have to define what parts of the confusion matrix are important for your problem). Finally, we pick the 'best' hyperparameters and calculate the prediction performance on the final validation set. This performance metrics are the ones that you report.
New contributor
MachineLearner is a new contributor to this site. Take care in asking for clarification, commenting, and answering.
Check out our Code of Conduct.
$endgroup$
add a comment |
$begingroup$
The previous answer already got accepted, but I am answering this question just to make sure that things are clear. I will go one step deeper which can be helpful to advanced people.
First of all, cross validation is a model selection mechanism that is used mainly to select hyperparameters. Changing hyperparameters will affect the number of parameters in the model. For example, increasing the number of layers in a neural network can introduce thousands more parameters ( depending on the width of the layer).
Second, almost any training algorithm can have unlimited number of possible hyperparameters. To make sure this is clear, let me give an example: in CNN, the number of layers is a hyperparameter that can take in theory any value between 1 and infinite, which means by just changing this hyparameter, I can generate infinite number of models. At the same time, the number of levels (depth) in decision tree is a hyperparameter that can take also a value between 1 and infinite, which means I can generate infinite number of models using decision tree, yet we use cross validation with decision tree but not cnn!!!!
Do not confused hyperparameters with parameters, cross validation has nothing to do with parameters it is only about hyperparameters and different training algorithms. Changing the values of the parameters will be taken care of by training algorithm.
Let us go back to the original question, why do not we use cross validation with CNN??
In fact, the answer to this question is based on a very important concept in machine learning. Variance error vs. Biased error. Let us say you have N models that you trained, they all have variance error and zero biased error, in this case using cross validation to select a model is not useful, but averaging the models is useful. If you have N models that all have different biased errors (non zero), then using cross validation is useful to select the best model, but averaging is harmful.
Any time you have models that have different biased errors, use cross validation to determine the best model. Anytime you have models that have variance errors, use averaging to determine the final outcome.
CNN has tendency toward overfitting not underfitting. Today we know that the deeper the network the better, but overfitting is what scares us.
CNN are good targets for averaging rather than selection, that is why some times they train four or five models and then they average their outputs.
The concepts to select network architecture, was studied in literature. They made it clear how to select your hyper parameters. In fact, if you have a lot of data just go for larger models.
I recommend you read the following papers:
1- Alex- Hinton paper in 2012, the paper where Alex proposed his network. You will see that most of the tricks they proposed is to deal with overfitting (variance error) and not biased errors.
2- super learner,
Super Learner In Prediction
This paper explains mathematically what is cross validation. Many people think about cross validation as a set of training/testing experiments that scans a set of parameters and returns the best model, but they ignore if this is enough to guarantee that this is the best model I can get using the training data available. They also ignore all the assumptions that cross validation needs to guarantee that the returned model is the super learner.
$endgroup$
add a comment |
Your Answer
StackExchange.ifUsing("editor", function ()
return StackExchange.using("mathjaxEditing", function ()
StackExchange.MarkdownEditor.creationCallbacks.add(function (editor, postfix)
StackExchange.mathjaxEditing.prepareWmdForMathJax(editor, postfix, [["$", "$"], ["\\(","\\)"]]);
);
);
, "mathjax-editing");
StackExchange.ready(function()
var channelOptions =
tags: "".split(" "),
id: "557"
;
initTagRenderer("".split(" "), "".split(" "), channelOptions);
StackExchange.using("externalEditor", function()
// Have to fire editor after snippets, if snippets enabled
if (StackExchange.settings.snippets.snippetsEnabled)
StackExchange.using("snippets", function()
createEditor();
);
else
createEditor();
);
function createEditor()
StackExchange.prepareEditor(
heartbeatType: 'answer',
autoActivateHeartbeat: false,
convertImagesToLinks: false,
noModals: true,
showLowRepImageUploadWarning: true,
reputationToPostImages: null,
bindNavPrevention: true,
postfix: "",
imageUploader:
brandingHtml: "Powered by u003ca class="icon-imgur-white" href="https://imgur.com/"u003eu003c/au003e",
contentPolicyHtml: "User contributions licensed under u003ca href="https://creativecommons.org/licenses/by-sa/3.0/"u003ecc by-sa 3.0 with attribution requiredu003c/au003e u003ca href="https://stackoverflow.com/legal/content-policy"u003e(content policy)u003c/au003e",
allowUrls: true
,
onDemand: true,
discardSelector: ".discard-answer"
,immediatelyShowMarkdownHelp:true
);
);
Sign up or log in
StackExchange.ready(function ()
StackExchange.helpers.onClickDraftSave('#login-link');
);
Sign up using Google
Sign up using Facebook
Sign up using Email and Password
Post as a guest
Required, but never shown
StackExchange.ready(
function ()
StackExchange.openid.initPostLogin('.new-post-login', 'https%3a%2f%2fdatascience.stackexchange.com%2fquestions%2f47797%2fusing-cross-validation-technique-for-a-cnn-model%23new-answer', 'question_page');
);
Post as a guest
Required, but never shown
2 Answers
2
active
oldest
votes
2 Answers
2
active
oldest
votes
active
oldest
votes
active
oldest
votes
$begingroup$
Question 1: Why do most CNN models not apply the cross-validation technique?
$k$-fold cross-validation is often used for simple models with few parameters, models with simple hyperparameters and additionally the models are easy to optimize. Typical examples are linear regression, logistic regression, small neural networks and support vector machines.
For a convolutional neural network with many parameters (e.g. more than one million) we just have too many possible changes in the architecture. What you can do is to do some experiments with the learning rate, batch size, dropout (amount and position) and batch normalization (position). Training a convolutional neural network with a huge dataset takes quite a long time. Doing hyperparameter optimization would just be total overkill. Often in papers, they try to improve the results of other research papers. It is not the goal to get better results by improving the chosen hyperparameters but rather to come up with new ideas to solve the given task but with better accuracy or less computational effort.
Question 2: If I use cross-validation how can I generate confusion
matrix? can I split dataset to train/test then do cross-validation on
train set as train/validation (i.e. doing cross-validation as
train/validation except for the usual train/test) and at last use test
set the same way? or how?
In order to do $k$-fold cross validation you will need to split your initial data set into two parts. One dataset for doing the hyperparameter optimization and one for the final validation. Then we take the dataset for the hyperparameter optimization and split it into $k$ (hopefully) equally sized data sets $mathcalD_1,mathcalD_2,ldots,mathcalD_k$. For the sake of clarity let us set $k=3$. Then for each possible hyperparameter combination that we want to test we use $mathcalD_1$ and $mathcalD_2$ to fit our model and we use $mathcalD_3$ to validate our model. Then we do the same with $mathcalD_2$ and $mathcalD_3$ and use $mathcalD_1$ for validation. Then we do the same with $mathcalD_1$ and $mathcalD_3$ and use $mathcalD_2$ for validation. We will get $3$ confusion matrices for every possible hyperparameter configuration. In order to derive a metric from these three results, we take the mean of these confusion matrices. Then we can scan through all averaged confusion matrices so select the hyperparameter configuration that was the best (you have to define what parts of the confusion matrix are important for your problem). Finally, we pick the 'best' hyperparameters and calculate the prediction performance on the final validation set. This performance metrics are the ones that you report.
New contributor
MachineLearner is a new contributor to this site. Take care in asking for clarification, commenting, and answering.
Check out our Code of Conduct.
$endgroup$
add a comment |
$begingroup$
Question 1: Why do most CNN models not apply the cross-validation technique?
$k$-fold cross-validation is often used for simple models with few parameters, models with simple hyperparameters and additionally the models are easy to optimize. Typical examples are linear regression, logistic regression, small neural networks and support vector machines.
For a convolutional neural network with many parameters (e.g. more than one million) we just have too many possible changes in the architecture. What you can do is to do some experiments with the learning rate, batch size, dropout (amount and position) and batch normalization (position). Training a convolutional neural network with a huge dataset takes quite a long time. Doing hyperparameter optimization would just be total overkill. Often in papers, they try to improve the results of other research papers. It is not the goal to get better results by improving the chosen hyperparameters but rather to come up with new ideas to solve the given task but with better accuracy or less computational effort.
Question 2: If I use cross-validation how can I generate confusion
matrix? can I split dataset to train/test then do cross-validation on
train set as train/validation (i.e. doing cross-validation as
train/validation except for the usual train/test) and at last use test
set the same way? or how?
In order to do $k$-fold cross validation you will need to split your initial data set into two parts. One dataset for doing the hyperparameter optimization and one for the final validation. Then we take the dataset for the hyperparameter optimization and split it into $k$ (hopefully) equally sized data sets $mathcalD_1,mathcalD_2,ldots,mathcalD_k$. For the sake of clarity let us set $k=3$. Then for each possible hyperparameter combination that we want to test we use $mathcalD_1$ and $mathcalD_2$ to fit our model and we use $mathcalD_3$ to validate our model. Then we do the same with $mathcalD_2$ and $mathcalD_3$ and use $mathcalD_1$ for validation. Then we do the same with $mathcalD_1$ and $mathcalD_3$ and use $mathcalD_2$ for validation. We will get $3$ confusion matrices for every possible hyperparameter configuration. In order to derive a metric from these three results, we take the mean of these confusion matrices. Then we can scan through all averaged confusion matrices so select the hyperparameter configuration that was the best (you have to define what parts of the confusion matrix are important for your problem). Finally, we pick the 'best' hyperparameters and calculate the prediction performance on the final validation set. This performance metrics are the ones that you report.
New contributor
MachineLearner is a new contributor to this site. Take care in asking for clarification, commenting, and answering.
Check out our Code of Conduct.
$endgroup$
add a comment |
$begingroup$
Question 1: Why do most CNN models not apply the cross-validation technique?
$k$-fold cross-validation is often used for simple models with few parameters, models with simple hyperparameters and additionally the models are easy to optimize. Typical examples are linear regression, logistic regression, small neural networks and support vector machines.
For a convolutional neural network with many parameters (e.g. more than one million) we just have too many possible changes in the architecture. What you can do is to do some experiments with the learning rate, batch size, dropout (amount and position) and batch normalization (position). Training a convolutional neural network with a huge dataset takes quite a long time. Doing hyperparameter optimization would just be total overkill. Often in papers, they try to improve the results of other research papers. It is not the goal to get better results by improving the chosen hyperparameters but rather to come up with new ideas to solve the given task but with better accuracy or less computational effort.
Question 2: If I use cross-validation how can I generate confusion
matrix? can I split dataset to train/test then do cross-validation on
train set as train/validation (i.e. doing cross-validation as
train/validation except for the usual train/test) and at last use test
set the same way? or how?
In order to do $k$-fold cross validation you will need to split your initial data set into two parts. One dataset for doing the hyperparameter optimization and one for the final validation. Then we take the dataset for the hyperparameter optimization and split it into $k$ (hopefully) equally sized data sets $mathcalD_1,mathcalD_2,ldots,mathcalD_k$. For the sake of clarity let us set $k=3$. Then for each possible hyperparameter combination that we want to test we use $mathcalD_1$ and $mathcalD_2$ to fit our model and we use $mathcalD_3$ to validate our model. Then we do the same with $mathcalD_2$ and $mathcalD_3$ and use $mathcalD_1$ for validation. Then we do the same with $mathcalD_1$ and $mathcalD_3$ and use $mathcalD_2$ for validation. We will get $3$ confusion matrices for every possible hyperparameter configuration. In order to derive a metric from these three results, we take the mean of these confusion matrices. Then we can scan through all averaged confusion matrices so select the hyperparameter configuration that was the best (you have to define what parts of the confusion matrix are important for your problem). Finally, we pick the 'best' hyperparameters and calculate the prediction performance on the final validation set. This performance metrics are the ones that you report.
New contributor
MachineLearner is a new contributor to this site. Take care in asking for clarification, commenting, and answering.
Check out our Code of Conduct.
$endgroup$
Question 1: Why do most CNN models not apply the cross-validation technique?
$k$-fold cross-validation is often used for simple models with few parameters, models with simple hyperparameters and additionally the models are easy to optimize. Typical examples are linear regression, logistic regression, small neural networks and support vector machines.
For a convolutional neural network with many parameters (e.g. more than one million) we just have too many possible changes in the architecture. What you can do is to do some experiments with the learning rate, batch size, dropout (amount and position) and batch normalization (position). Training a convolutional neural network with a huge dataset takes quite a long time. Doing hyperparameter optimization would just be total overkill. Often in papers, they try to improve the results of other research papers. It is not the goal to get better results by improving the chosen hyperparameters but rather to come up with new ideas to solve the given task but with better accuracy or less computational effort.
Question 2: If I use cross-validation how can I generate confusion
matrix? can I split dataset to train/test then do cross-validation on
train set as train/validation (i.e. doing cross-validation as
train/validation except for the usual train/test) and at last use test
set the same way? or how?
In order to do $k$-fold cross validation you will need to split your initial data set into two parts. One dataset for doing the hyperparameter optimization and one for the final validation. Then we take the dataset for the hyperparameter optimization and split it into $k$ (hopefully) equally sized data sets $mathcalD_1,mathcalD_2,ldots,mathcalD_k$. For the sake of clarity let us set $k=3$. Then for each possible hyperparameter combination that we want to test we use $mathcalD_1$ and $mathcalD_2$ to fit our model and we use $mathcalD_3$ to validate our model. Then we do the same with $mathcalD_2$ and $mathcalD_3$ and use $mathcalD_1$ for validation. Then we do the same with $mathcalD_1$ and $mathcalD_3$ and use $mathcalD_2$ for validation. We will get $3$ confusion matrices for every possible hyperparameter configuration. In order to derive a metric from these three results, we take the mean of these confusion matrices. Then we can scan through all averaged confusion matrices so select the hyperparameter configuration that was the best (you have to define what parts of the confusion matrix are important for your problem). Finally, we pick the 'best' hyperparameters and calculate the prediction performance on the final validation set. This performance metrics are the ones that you report.
New contributor
MachineLearner is a new contributor to this site. Take care in asking for clarification, commenting, and answering.
Check out our Code of Conduct.
New contributor
MachineLearner is a new contributor to this site. Take care in asking for clarification, commenting, and answering.
Check out our Code of Conduct.
answered Mar 22 at 16:10
MachineLearnerMachineLearner
36910
36910
New contributor
MachineLearner is a new contributor to this site. Take care in asking for clarification, commenting, and answering.
Check out our Code of Conduct.
New contributor
MachineLearner is a new contributor to this site. Take care in asking for clarification, commenting, and answering.
Check out our Code of Conduct.
MachineLearner is a new contributor to this site. Take care in asking for clarification, commenting, and answering.
Check out our Code of Conduct.
add a comment |
add a comment |
$begingroup$
The previous answer already got accepted, but I am answering this question just to make sure that things are clear. I will go one step deeper which can be helpful to advanced people.
First of all, cross validation is a model selection mechanism that is used mainly to select hyperparameters. Changing hyperparameters will affect the number of parameters in the model. For example, increasing the number of layers in a neural network can introduce thousands more parameters ( depending on the width of the layer).
Second, almost any training algorithm can have unlimited number of possible hyperparameters. To make sure this is clear, let me give an example: in CNN, the number of layers is a hyperparameter that can take in theory any value between 1 and infinite, which means by just changing this hyparameter, I can generate infinite number of models. At the same time, the number of levels (depth) in decision tree is a hyperparameter that can take also a value between 1 and infinite, which means I can generate infinite number of models using decision tree, yet we use cross validation with decision tree but not cnn!!!!
Do not confused hyperparameters with parameters, cross validation has nothing to do with parameters it is only about hyperparameters and different training algorithms. Changing the values of the parameters will be taken care of by training algorithm.
Let us go back to the original question, why do not we use cross validation with CNN??
In fact, the answer to this question is based on a very important concept in machine learning. Variance error vs. Biased error. Let us say you have N models that you trained, they all have variance error and zero biased error, in this case using cross validation to select a model is not useful, but averaging the models is useful. If you have N models that all have different biased errors (non zero), then using cross validation is useful to select the best model, but averaging is harmful.
Any time you have models that have different biased errors, use cross validation to determine the best model. Anytime you have models that have variance errors, use averaging to determine the final outcome.
CNN has tendency toward overfitting not underfitting. Today we know that the deeper the network the better, but overfitting is what scares us.
CNN are good targets for averaging rather than selection, that is why some times they train four or five models and then they average their outputs.
The concepts to select network architecture, was studied in literature. They made it clear how to select your hyper parameters. In fact, if you have a lot of data just go for larger models.
I recommend you read the following papers:
1- Alex- Hinton paper in 2012, the paper where Alex proposed his network. You will see that most of the tricks they proposed is to deal with overfitting (variance error) and not biased errors.
2- super learner,
Super Learner In Prediction
This paper explains mathematically what is cross validation. Many people think about cross validation as a set of training/testing experiments that scans a set of parameters and returns the best model, but they ignore if this is enough to guarantee that this is the best model I can get using the training data available. They also ignore all the assumptions that cross validation needs to guarantee that the returned model is the super learner.
$endgroup$
add a comment |
$begingroup$
The previous answer already got accepted, but I am answering this question just to make sure that things are clear. I will go one step deeper which can be helpful to advanced people.
First of all, cross validation is a model selection mechanism that is used mainly to select hyperparameters. Changing hyperparameters will affect the number of parameters in the model. For example, increasing the number of layers in a neural network can introduce thousands more parameters ( depending on the width of the layer).
Second, almost any training algorithm can have unlimited number of possible hyperparameters. To make sure this is clear, let me give an example: in CNN, the number of layers is a hyperparameter that can take in theory any value between 1 and infinite, which means by just changing this hyparameter, I can generate infinite number of models. At the same time, the number of levels (depth) in decision tree is a hyperparameter that can take also a value between 1 and infinite, which means I can generate infinite number of models using decision tree, yet we use cross validation with decision tree but not cnn!!!!
Do not confused hyperparameters with parameters, cross validation has nothing to do with parameters it is only about hyperparameters and different training algorithms. Changing the values of the parameters will be taken care of by training algorithm.
Let us go back to the original question, why do not we use cross validation with CNN??
In fact, the answer to this question is based on a very important concept in machine learning. Variance error vs. Biased error. Let us say you have N models that you trained, they all have variance error and zero biased error, in this case using cross validation to select a model is not useful, but averaging the models is useful. If you have N models that all have different biased errors (non zero), then using cross validation is useful to select the best model, but averaging is harmful.
Any time you have models that have different biased errors, use cross validation to determine the best model. Anytime you have models that have variance errors, use averaging to determine the final outcome.
CNN has tendency toward overfitting not underfitting. Today we know that the deeper the network the better, but overfitting is what scares us.
CNN are good targets for averaging rather than selection, that is why some times they train four or five models and then they average their outputs.
The concepts to select network architecture, was studied in literature. They made it clear how to select your hyper parameters. In fact, if you have a lot of data just go for larger models.
I recommend you read the following papers:
1- Alex- Hinton paper in 2012, the paper where Alex proposed his network. You will see that most of the tricks they proposed is to deal with overfitting (variance error) and not biased errors.
2- super learner,
Super Learner In Prediction
This paper explains mathematically what is cross validation. Many people think about cross validation as a set of training/testing experiments that scans a set of parameters and returns the best model, but they ignore if this is enough to guarantee that this is the best model I can get using the training data available. They also ignore all the assumptions that cross validation needs to guarantee that the returned model is the super learner.
$endgroup$
add a comment |
$begingroup$
The previous answer already got accepted, but I am answering this question just to make sure that things are clear. I will go one step deeper which can be helpful to advanced people.
First of all, cross validation is a model selection mechanism that is used mainly to select hyperparameters. Changing hyperparameters will affect the number of parameters in the model. For example, increasing the number of layers in a neural network can introduce thousands more parameters ( depending on the width of the layer).
Second, almost any training algorithm can have unlimited number of possible hyperparameters. To make sure this is clear, let me give an example: in CNN, the number of layers is a hyperparameter that can take in theory any value between 1 and infinite, which means by just changing this hyparameter, I can generate infinite number of models. At the same time, the number of levels (depth) in decision tree is a hyperparameter that can take also a value between 1 and infinite, which means I can generate infinite number of models using decision tree, yet we use cross validation with decision tree but not cnn!!!!
Do not confused hyperparameters with parameters, cross validation has nothing to do with parameters it is only about hyperparameters and different training algorithms. Changing the values of the parameters will be taken care of by training algorithm.
Let us go back to the original question, why do not we use cross validation with CNN??
In fact, the answer to this question is based on a very important concept in machine learning. Variance error vs. Biased error. Let us say you have N models that you trained, they all have variance error and zero biased error, in this case using cross validation to select a model is not useful, but averaging the models is useful. If you have N models that all have different biased errors (non zero), then using cross validation is useful to select the best model, but averaging is harmful.
Any time you have models that have different biased errors, use cross validation to determine the best model. Anytime you have models that have variance errors, use averaging to determine the final outcome.
CNN has tendency toward overfitting not underfitting. Today we know that the deeper the network the better, but overfitting is what scares us.
CNN are good targets for averaging rather than selection, that is why some times they train four or five models and then they average their outputs.
The concepts to select network architecture, was studied in literature. They made it clear how to select your hyper parameters. In fact, if you have a lot of data just go for larger models.
I recommend you read the following papers:
1- Alex- Hinton paper in 2012, the paper where Alex proposed his network. You will see that most of the tricks they proposed is to deal with overfitting (variance error) and not biased errors.
2- super learner,
Super Learner In Prediction
This paper explains mathematically what is cross validation. Many people think about cross validation as a set of training/testing experiments that scans a set of parameters and returns the best model, but they ignore if this is enough to guarantee that this is the best model I can get using the training data available. They also ignore all the assumptions that cross validation needs to guarantee that the returned model is the super learner.
$endgroup$
The previous answer already got accepted, but I am answering this question just to make sure that things are clear. I will go one step deeper which can be helpful to advanced people.
First of all, cross validation is a model selection mechanism that is used mainly to select hyperparameters. Changing hyperparameters will affect the number of parameters in the model. For example, increasing the number of layers in a neural network can introduce thousands more parameters ( depending on the width of the layer).
Second, almost any training algorithm can have unlimited number of possible hyperparameters. To make sure this is clear, let me give an example: in CNN, the number of layers is a hyperparameter that can take in theory any value between 1 and infinite, which means by just changing this hyparameter, I can generate infinite number of models. At the same time, the number of levels (depth) in decision tree is a hyperparameter that can take also a value between 1 and infinite, which means I can generate infinite number of models using decision tree, yet we use cross validation with decision tree but not cnn!!!!
Do not confused hyperparameters with parameters, cross validation has nothing to do with parameters it is only about hyperparameters and different training algorithms. Changing the values of the parameters will be taken care of by training algorithm.
Let us go back to the original question, why do not we use cross validation with CNN??
In fact, the answer to this question is based on a very important concept in machine learning. Variance error vs. Biased error. Let us say you have N models that you trained, they all have variance error and zero biased error, in this case using cross validation to select a model is not useful, but averaging the models is useful. If you have N models that all have different biased errors (non zero), then using cross validation is useful to select the best model, but averaging is harmful.
Any time you have models that have different biased errors, use cross validation to determine the best model. Anytime you have models that have variance errors, use averaging to determine the final outcome.
CNN has tendency toward overfitting not underfitting. Today we know that the deeper the network the better, but overfitting is what scares us.
CNN are good targets for averaging rather than selection, that is why some times they train four or five models and then they average their outputs.
The concepts to select network architecture, was studied in literature. They made it clear how to select your hyper parameters. In fact, if you have a lot of data just go for larger models.
I recommend you read the following papers:
1- Alex- Hinton paper in 2012, the paper where Alex proposed his network. You will see that most of the tricks they proposed is to deal with overfitting (variance error) and not biased errors.
2- super learner,
Super Learner In Prediction
This paper explains mathematically what is cross validation. Many people think about cross validation as a set of training/testing experiments that scans a set of parameters and returns the best model, but they ignore if this is enough to guarantee that this is the best model I can get using the training data available. They also ignore all the assumptions that cross validation needs to guarantee that the returned model is the super learner.
answered Mar 23 at 2:59


Bashar HaddadBashar Haddad
1,2521313
1,2521313
add a comment |
add a comment |
Thanks for contributing an answer to Data Science Stack Exchange!
- Please be sure to answer the question. Provide details and share your research!
But avoid …
- Asking for help, clarification, or responding to other answers.
- Making statements based on opinion; back them up with references or personal experience.
Use MathJax to format equations. MathJax reference.
To learn more, see our tips on writing great answers.
Sign up or log in
StackExchange.ready(function ()
StackExchange.helpers.onClickDraftSave('#login-link');
);
Sign up using Google
Sign up using Facebook
Sign up using Email and Password
Post as a guest
Required, but never shown
StackExchange.ready(
function ()
StackExchange.openid.initPostLogin('.new-post-login', 'https%3a%2f%2fdatascience.stackexchange.com%2fquestions%2f47797%2fusing-cross-validation-technique-for-a-cnn-model%23new-answer', 'question_page');
);
Post as a guest
Required, but never shown
Sign up or log in
StackExchange.ready(function ()
StackExchange.helpers.onClickDraftSave('#login-link');
);
Sign up using Google
Sign up using Facebook
Sign up using Email and Password
Post as a guest
Required, but never shown
Sign up or log in
StackExchange.ready(function ()
StackExchange.helpers.onClickDraftSave('#login-link');
);
Sign up using Google
Sign up using Facebook
Sign up using Email and Password
Post as a guest
Required, but never shown
Sign up or log in
StackExchange.ready(function ()
StackExchange.helpers.onClickDraftSave('#login-link');
);
Sign up using Google
Sign up using Facebook
Sign up using Email and Password
Sign up using Google
Sign up using Facebook
Sign up using Email and Password
Post as a guest
Required, but never shown
Required, but never shown
Required, but never shown
Required, but never shown
Required, but never shown
Required, but never shown
Required, but never shown
Required, but never shown
Required, but never shown
HRL5BI,B1zj1kV3K8Ld