Q-Learning experience replay: how to feed the neural network? The 2019 Stack Overflow Developer Survey Results Are InWhat is “experience replay” and what are its benefits?Deep Reinforcent Learning Model, experience replayQ learning neural network experience replay problemIs this a Q-learning algorithm or just brute force?How does a Q algorithm consider future rewards?Experience Replay ExplainPrioritized Experience Replay - why to approximate the Density Function?Difference between advantages of Experience Replay in DQN2013 paperWhy is my loss function for DQN converging too quickly?Potential-based reward shaping in DQN reinforcement learning
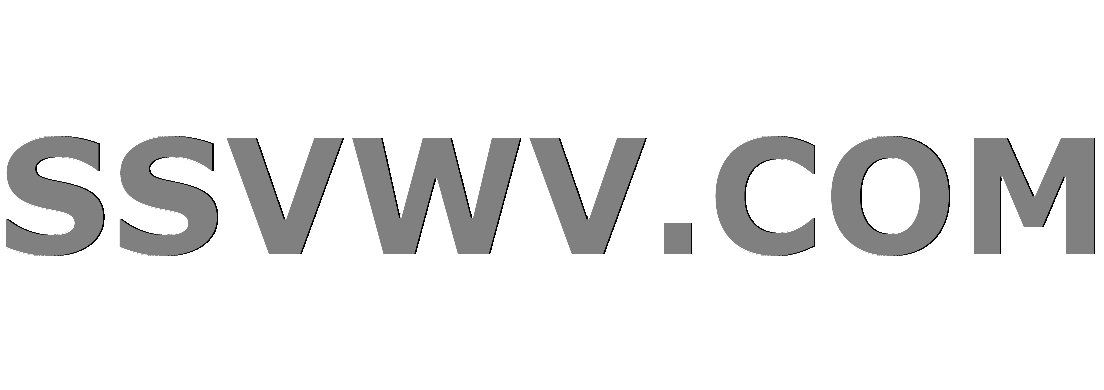
Multi tool use
How to type this arrow in math mode?
Why is the maximum length of OpenWrt’s root password 8 characters?
Can we generate random numbers using irrational numbers like π and e?
Is Sun brighter than what we actually see?
Why don't hard Brexiteers insist on a hard border to prevent illegal immigration after Brexit?
Button changing its text & action. Good or terrible?
"consumers choosing to rely" vs. "consumers to choose to rely"
Did Scotland spend $250,000 for the slogan "Welcome to Scotland"?
Is an up-to-date browser secure on an out-of-date OS?
The difference between dialogue marks
How to manage monthly salary
When should I buy a clipper card after flying to Oakland?
Why doesn't shell automatically fix "useless use of cat"?
How to notate time signature switching consistently every measure
If I can cast sorceries at instant speed, can I use sorcery-speed activated abilities at instant speed?
Worn-tile Scrabble
Falsification in Math vs Science
Deal with toxic manager when you can't quit
What could be the right powersource for 15 seconds lifespan disposable giant chainsaw?
How to type a long/em dash `—`
Using xargs with pdftk
What do hard-Brexiteers want with respect to the Irish border?
slides for 30min~1hr skype tenure track application interview
How to deal with speedster characters?
Q-Learning experience replay: how to feed the neural network?
The 2019 Stack Overflow Developer Survey Results Are InWhat is “experience replay” and what are its benefits?Deep Reinforcent Learning Model, experience replayQ learning neural network experience replay problemIs this a Q-learning algorithm or just brute force?How does a Q algorithm consider future rewards?Experience Replay ExplainPrioritized Experience Replay - why to approximate the Density Function?Difference between advantages of Experience Replay in DQN2013 paperWhy is my loss function for DQN converging too quickly?Potential-based reward shaping in DQN reinforcement learning
$begingroup$
I'm trying to replicate the DQN Atari experiment. Actually my DQN isn't performing well; checking another one's codes, I saw something about experience replay which I don't understand. First, when you define your CNN, in the first layer you have to specify the size (I'm using Keras + Tensorflow so in my case it's something like (105, 80, 4), which corresponds to height, width and number of images I feed my CNN.). In the codes I revisited, when they get the minibatch from the memory, I see they usually fed the CNN without "packing" it on 4 batches. How it is possible? I mean for example if you get 32 random sampled experiences, don't you need to make batches of 4 before feeding it?
Here are an example of what I'm saying: https://github.com/yilundu/DQN-DDQN-on-Space-Invaders/blob/master/replay_buffer.py
https://github.com/yilundu/DQN-DDQN-on-Space-Invaders/blob/master/deep_Q.py
In this code, that's how he/she stores the experiences:
def add(self, s, a, r, d, s2):
"""Add an experience to the buffer"""
# S represents current state, a is action,
# r is reward, d is whether it is the end,
# and s2 is next state
experience = (s, a, r, d, s2)
if self.count < self.buffer_size:
self.buffer.append(experience)
self.count += 1
else:
self.buffer.popleft()
self.buffer.append(experience)
Then when you need to use them:
def sample(self, batch_size):
"""Samples a total of elements equal to batch_size from buffer
if buffer contains enough elements. Otherwise return all elements"""
batch = []
if self.count < batch_size:
batch = random.sample(self.buffer, self.count)
else:
batch = random.sample(self.buffer, batch_size)
# Maps each experience in batch in batches of states, actions, rewards
# and new states
s_batch, a_batch, r_batch, d_batch, s2_batch = list(map(np.array, list(zip(*batch))))
return s_batch, a_batch, r_batch, d_batch, s2_batch
Ok, so now you have a batch of 32 states, actions, rewards, done and next states.
This is how you feed the state batch (s_batch) and next state batch (s2_batch) to the CNN:
def train(self, s_batch, a_batch, r_batch, d_batch, s2_batch, observation_num):
"""Trains network to fit given parameters"""
batch_size = s_batch.shape[0]
targets = np.zeros((batch_size, NUM_ACTIONS))
for i in range(batch_size):
targets[i] = self.model.predict(s_batch[i].reshape(1, 84, 84, NUM_FRAMES), batch_size = 1)
fut_action = self.target_model.predict(s2_batch[i].reshape(1, 84, 84, NUM_FRAMES), batch_size = 1)
targets[i, a_batch[i]] = r_batch[i]
if d_batch[i] == False:
targets[i, a_batch[i]] += DECAY_RATE * np.max(fut_action)
loss = self.model.train_on_batch(s_batch, targets)
# Print the loss every 10 iterations.
if observation_num % 10 == 0:
print("We had a loss equal to ", loss)
In my code (https://bitbucket.org/jocapal/dqn_public/src/master/Deimos_v2_13.py) I get a batch of 32 experiences; then make small batches of 4 experiences and feed the CNN. My question is: am I doing it wrong? And if so, how can I feed 32 experiences when my CNN is waiting for 4 experiences?
Another example of what I'm saying: https://yanpanlau.github.io/2016/07/10/FlappyBird-Keras.html
python reinforcement-learning q-learning dqn keras-rl
New contributor
Joaquin is a new contributor to this site. Take care in asking for clarification, commenting, and answering.
Check out our Code of Conduct.
$endgroup$
add a comment |
$begingroup$
I'm trying to replicate the DQN Atari experiment. Actually my DQN isn't performing well; checking another one's codes, I saw something about experience replay which I don't understand. First, when you define your CNN, in the first layer you have to specify the size (I'm using Keras + Tensorflow so in my case it's something like (105, 80, 4), which corresponds to height, width and number of images I feed my CNN.). In the codes I revisited, when they get the minibatch from the memory, I see they usually fed the CNN without "packing" it on 4 batches. How it is possible? I mean for example if you get 32 random sampled experiences, don't you need to make batches of 4 before feeding it?
Here are an example of what I'm saying: https://github.com/yilundu/DQN-DDQN-on-Space-Invaders/blob/master/replay_buffer.py
https://github.com/yilundu/DQN-DDQN-on-Space-Invaders/blob/master/deep_Q.py
In this code, that's how he/she stores the experiences:
def add(self, s, a, r, d, s2):
"""Add an experience to the buffer"""
# S represents current state, a is action,
# r is reward, d is whether it is the end,
# and s2 is next state
experience = (s, a, r, d, s2)
if self.count < self.buffer_size:
self.buffer.append(experience)
self.count += 1
else:
self.buffer.popleft()
self.buffer.append(experience)
Then when you need to use them:
def sample(self, batch_size):
"""Samples a total of elements equal to batch_size from buffer
if buffer contains enough elements. Otherwise return all elements"""
batch = []
if self.count < batch_size:
batch = random.sample(self.buffer, self.count)
else:
batch = random.sample(self.buffer, batch_size)
# Maps each experience in batch in batches of states, actions, rewards
# and new states
s_batch, a_batch, r_batch, d_batch, s2_batch = list(map(np.array, list(zip(*batch))))
return s_batch, a_batch, r_batch, d_batch, s2_batch
Ok, so now you have a batch of 32 states, actions, rewards, done and next states.
This is how you feed the state batch (s_batch) and next state batch (s2_batch) to the CNN:
def train(self, s_batch, a_batch, r_batch, d_batch, s2_batch, observation_num):
"""Trains network to fit given parameters"""
batch_size = s_batch.shape[0]
targets = np.zeros((batch_size, NUM_ACTIONS))
for i in range(batch_size):
targets[i] = self.model.predict(s_batch[i].reshape(1, 84, 84, NUM_FRAMES), batch_size = 1)
fut_action = self.target_model.predict(s2_batch[i].reshape(1, 84, 84, NUM_FRAMES), batch_size = 1)
targets[i, a_batch[i]] = r_batch[i]
if d_batch[i] == False:
targets[i, a_batch[i]] += DECAY_RATE * np.max(fut_action)
loss = self.model.train_on_batch(s_batch, targets)
# Print the loss every 10 iterations.
if observation_num % 10 == 0:
print("We had a loss equal to ", loss)
In my code (https://bitbucket.org/jocapal/dqn_public/src/master/Deimos_v2_13.py) I get a batch of 32 experiences; then make small batches of 4 experiences and feed the CNN. My question is: am I doing it wrong? And if so, how can I feed 32 experiences when my CNN is waiting for 4 experiences?
Another example of what I'm saying: https://yanpanlau.github.io/2016/07/10/FlappyBird-Keras.html
python reinforcement-learning q-learning dqn keras-rl
New contributor
Joaquin is a new contributor to this site. Take care in asking for clarification, commenting, and answering.
Check out our Code of Conduct.
$endgroup$
add a comment |
$begingroup$
I'm trying to replicate the DQN Atari experiment. Actually my DQN isn't performing well; checking another one's codes, I saw something about experience replay which I don't understand. First, when you define your CNN, in the first layer you have to specify the size (I'm using Keras + Tensorflow so in my case it's something like (105, 80, 4), which corresponds to height, width and number of images I feed my CNN.). In the codes I revisited, when they get the minibatch from the memory, I see they usually fed the CNN without "packing" it on 4 batches. How it is possible? I mean for example if you get 32 random sampled experiences, don't you need to make batches of 4 before feeding it?
Here are an example of what I'm saying: https://github.com/yilundu/DQN-DDQN-on-Space-Invaders/blob/master/replay_buffer.py
https://github.com/yilundu/DQN-DDQN-on-Space-Invaders/blob/master/deep_Q.py
In this code, that's how he/she stores the experiences:
def add(self, s, a, r, d, s2):
"""Add an experience to the buffer"""
# S represents current state, a is action,
# r is reward, d is whether it is the end,
# and s2 is next state
experience = (s, a, r, d, s2)
if self.count < self.buffer_size:
self.buffer.append(experience)
self.count += 1
else:
self.buffer.popleft()
self.buffer.append(experience)
Then when you need to use them:
def sample(self, batch_size):
"""Samples a total of elements equal to batch_size from buffer
if buffer contains enough elements. Otherwise return all elements"""
batch = []
if self.count < batch_size:
batch = random.sample(self.buffer, self.count)
else:
batch = random.sample(self.buffer, batch_size)
# Maps each experience in batch in batches of states, actions, rewards
# and new states
s_batch, a_batch, r_batch, d_batch, s2_batch = list(map(np.array, list(zip(*batch))))
return s_batch, a_batch, r_batch, d_batch, s2_batch
Ok, so now you have a batch of 32 states, actions, rewards, done and next states.
This is how you feed the state batch (s_batch) and next state batch (s2_batch) to the CNN:
def train(self, s_batch, a_batch, r_batch, d_batch, s2_batch, observation_num):
"""Trains network to fit given parameters"""
batch_size = s_batch.shape[0]
targets = np.zeros((batch_size, NUM_ACTIONS))
for i in range(batch_size):
targets[i] = self.model.predict(s_batch[i].reshape(1, 84, 84, NUM_FRAMES), batch_size = 1)
fut_action = self.target_model.predict(s2_batch[i].reshape(1, 84, 84, NUM_FRAMES), batch_size = 1)
targets[i, a_batch[i]] = r_batch[i]
if d_batch[i] == False:
targets[i, a_batch[i]] += DECAY_RATE * np.max(fut_action)
loss = self.model.train_on_batch(s_batch, targets)
# Print the loss every 10 iterations.
if observation_num % 10 == 0:
print("We had a loss equal to ", loss)
In my code (https://bitbucket.org/jocapal/dqn_public/src/master/Deimos_v2_13.py) I get a batch of 32 experiences; then make small batches of 4 experiences and feed the CNN. My question is: am I doing it wrong? And if so, how can I feed 32 experiences when my CNN is waiting for 4 experiences?
Another example of what I'm saying: https://yanpanlau.github.io/2016/07/10/FlappyBird-Keras.html
python reinforcement-learning q-learning dqn keras-rl
New contributor
Joaquin is a new contributor to this site. Take care in asking for clarification, commenting, and answering.
Check out our Code of Conduct.
$endgroup$
I'm trying to replicate the DQN Atari experiment. Actually my DQN isn't performing well; checking another one's codes, I saw something about experience replay which I don't understand. First, when you define your CNN, in the first layer you have to specify the size (I'm using Keras + Tensorflow so in my case it's something like (105, 80, 4), which corresponds to height, width and number of images I feed my CNN.). In the codes I revisited, when they get the minibatch from the memory, I see they usually fed the CNN without "packing" it on 4 batches. How it is possible? I mean for example if you get 32 random sampled experiences, don't you need to make batches of 4 before feeding it?
Here are an example of what I'm saying: https://github.com/yilundu/DQN-DDQN-on-Space-Invaders/blob/master/replay_buffer.py
https://github.com/yilundu/DQN-DDQN-on-Space-Invaders/blob/master/deep_Q.py
In this code, that's how he/she stores the experiences:
def add(self, s, a, r, d, s2):
"""Add an experience to the buffer"""
# S represents current state, a is action,
# r is reward, d is whether it is the end,
# and s2 is next state
experience = (s, a, r, d, s2)
if self.count < self.buffer_size:
self.buffer.append(experience)
self.count += 1
else:
self.buffer.popleft()
self.buffer.append(experience)
Then when you need to use them:
def sample(self, batch_size):
"""Samples a total of elements equal to batch_size from buffer
if buffer contains enough elements. Otherwise return all elements"""
batch = []
if self.count < batch_size:
batch = random.sample(self.buffer, self.count)
else:
batch = random.sample(self.buffer, batch_size)
# Maps each experience in batch in batches of states, actions, rewards
# and new states
s_batch, a_batch, r_batch, d_batch, s2_batch = list(map(np.array, list(zip(*batch))))
return s_batch, a_batch, r_batch, d_batch, s2_batch
Ok, so now you have a batch of 32 states, actions, rewards, done and next states.
This is how you feed the state batch (s_batch) and next state batch (s2_batch) to the CNN:
def train(self, s_batch, a_batch, r_batch, d_batch, s2_batch, observation_num):
"""Trains network to fit given parameters"""
batch_size = s_batch.shape[0]
targets = np.zeros((batch_size, NUM_ACTIONS))
for i in range(batch_size):
targets[i] = self.model.predict(s_batch[i].reshape(1, 84, 84, NUM_FRAMES), batch_size = 1)
fut_action = self.target_model.predict(s2_batch[i].reshape(1, 84, 84, NUM_FRAMES), batch_size = 1)
targets[i, a_batch[i]] = r_batch[i]
if d_batch[i] == False:
targets[i, a_batch[i]] += DECAY_RATE * np.max(fut_action)
loss = self.model.train_on_batch(s_batch, targets)
# Print the loss every 10 iterations.
if observation_num % 10 == 0:
print("We had a loss equal to ", loss)
In my code (https://bitbucket.org/jocapal/dqn_public/src/master/Deimos_v2_13.py) I get a batch of 32 experiences; then make small batches of 4 experiences and feed the CNN. My question is: am I doing it wrong? And if so, how can I feed 32 experiences when my CNN is waiting for 4 experiences?
Another example of what I'm saying: https://yanpanlau.github.io/2016/07/10/FlappyBird-Keras.html
python reinforcement-learning q-learning dqn keras-rl
python reinforcement-learning q-learning dqn keras-rl
New contributor
Joaquin is a new contributor to this site. Take care in asking for clarification, commenting, and answering.
Check out our Code of Conduct.
New contributor
Joaquin is a new contributor to this site. Take care in asking for clarification, commenting, and answering.
Check out our Code of Conduct.
edited 5 mins ago
Joaquin
New contributor
Joaquin is a new contributor to this site. Take care in asking for clarification, commenting, and answering.
Check out our Code of Conduct.
asked 16 mins ago
JoaquinJoaquin
12
12
New contributor
Joaquin is a new contributor to this site. Take care in asking for clarification, commenting, and answering.
Check out our Code of Conduct.
New contributor
Joaquin is a new contributor to this site. Take care in asking for clarification, commenting, and answering.
Check out our Code of Conduct.
Joaquin is a new contributor to this site. Take care in asking for clarification, commenting, and answering.
Check out our Code of Conduct.
add a comment |
add a comment |
0
active
oldest
votes
Your Answer
StackExchange.ifUsing("editor", function ()
return StackExchange.using("mathjaxEditing", function ()
StackExchange.MarkdownEditor.creationCallbacks.add(function (editor, postfix)
StackExchange.mathjaxEditing.prepareWmdForMathJax(editor, postfix, [["$", "$"], ["\\(","\\)"]]);
);
);
, "mathjax-editing");
StackExchange.ready(function()
var channelOptions =
tags: "".split(" "),
id: "557"
;
initTagRenderer("".split(" "), "".split(" "), channelOptions);
StackExchange.using("externalEditor", function()
// Have to fire editor after snippets, if snippets enabled
if (StackExchange.settings.snippets.snippetsEnabled)
StackExchange.using("snippets", function()
createEditor();
);
else
createEditor();
);
function createEditor()
StackExchange.prepareEditor(
heartbeatType: 'answer',
autoActivateHeartbeat: false,
convertImagesToLinks: false,
noModals: true,
showLowRepImageUploadWarning: true,
reputationToPostImages: null,
bindNavPrevention: true,
postfix: "",
imageUploader:
brandingHtml: "Powered by u003ca class="icon-imgur-white" href="https://imgur.com/"u003eu003c/au003e",
contentPolicyHtml: "User contributions licensed under u003ca href="https://creativecommons.org/licenses/by-sa/3.0/"u003ecc by-sa 3.0 with attribution requiredu003c/au003e u003ca href="https://stackoverflow.com/legal/content-policy"u003e(content policy)u003c/au003e",
allowUrls: true
,
onDemand: true,
discardSelector: ".discard-answer"
,immediatelyShowMarkdownHelp:true
);
);
Joaquin is a new contributor. Be nice, and check out our Code of Conduct.
Sign up or log in
StackExchange.ready(function ()
StackExchange.helpers.onClickDraftSave('#login-link');
);
Sign up using Google
Sign up using Facebook
Sign up using Email and Password
Post as a guest
Required, but never shown
StackExchange.ready(
function ()
StackExchange.openid.initPostLogin('.new-post-login', 'https%3a%2f%2fdatascience.stackexchange.com%2fquestions%2f49128%2fq-learning-experience-replay-how-to-feed-the-neural-network%23new-answer', 'question_page');
);
Post as a guest
Required, but never shown
0
active
oldest
votes
0
active
oldest
votes
active
oldest
votes
active
oldest
votes
Joaquin is a new contributor. Be nice, and check out our Code of Conduct.
Joaquin is a new contributor. Be nice, and check out our Code of Conduct.
Joaquin is a new contributor. Be nice, and check out our Code of Conduct.
Joaquin is a new contributor. Be nice, and check out our Code of Conduct.
Thanks for contributing an answer to Data Science Stack Exchange!
- Please be sure to answer the question. Provide details and share your research!
But avoid …
- Asking for help, clarification, or responding to other answers.
- Making statements based on opinion; back them up with references or personal experience.
Use MathJax to format equations. MathJax reference.
To learn more, see our tips on writing great answers.
Sign up or log in
StackExchange.ready(function ()
StackExchange.helpers.onClickDraftSave('#login-link');
);
Sign up using Google
Sign up using Facebook
Sign up using Email and Password
Post as a guest
Required, but never shown
StackExchange.ready(
function ()
StackExchange.openid.initPostLogin('.new-post-login', 'https%3a%2f%2fdatascience.stackexchange.com%2fquestions%2f49128%2fq-learning-experience-replay-how-to-feed-the-neural-network%23new-answer', 'question_page');
);
Post as a guest
Required, but never shown
Sign up or log in
StackExchange.ready(function ()
StackExchange.helpers.onClickDraftSave('#login-link');
);
Sign up using Google
Sign up using Facebook
Sign up using Email and Password
Post as a guest
Required, but never shown
Sign up or log in
StackExchange.ready(function ()
StackExchange.helpers.onClickDraftSave('#login-link');
);
Sign up using Google
Sign up using Facebook
Sign up using Email and Password
Post as a guest
Required, but never shown
Sign up or log in
StackExchange.ready(function ()
StackExchange.helpers.onClickDraftSave('#login-link');
);
Sign up using Google
Sign up using Facebook
Sign up using Email and Password
Sign up using Google
Sign up using Facebook
Sign up using Email and Password
Post as a guest
Required, but never shown
Required, but never shown
Required, but never shown
Required, but never shown
Required, but never shown
Required, but never shown
Required, but never shown
Required, but never shown
Required, but never shown
vN1IhtiTVN6Fjl9qxdyL5 D slab27gyazxOVuu