Classifying ultrasound videos with a small datasetBinary Classification on small dataset < 200 samplesBinary text classification problem with small label-dataset using kerasPredicting contract churn/cancellation: Great model results does not work in the real worldClassifying with certaintyRNN unable to classify time seriesLong term time series forecasts with small datasetExploratory analysis and feature engineering for time till failure prediction using sensor data of enginesUnsupervised binary classification on small datasetTime series analysis on small datasetSmall dataset in Time series
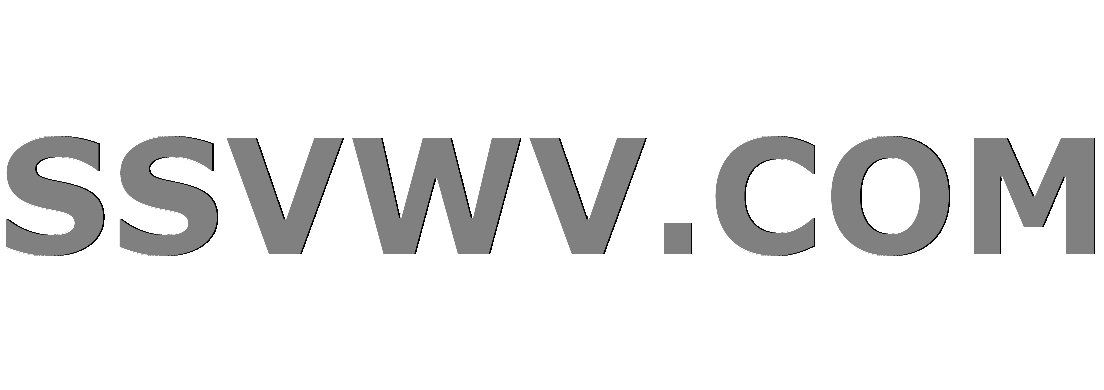
Multi tool use
Is it cheaper to drop cargo than to land it?
Would a 1/1 token with persist dying trigger on death effects a second time?
Would glacier 'trees' be plausible?
Airbnb - host wants to reduce rooms, can we get refund?
When and why did journal article titles become descriptive, rather than creatively allusive?
Short story with physics professor who "brings back the dead" (Asimov or Bradbury?)
Was Unix ever a single-user OS?
Roll Dice to get a random number between 1 and 150
How could a planet have most of its water in the atmosphere?
Moving the subject of the sentence into a dangling participle
Coefficients of linear dependency
I need a disease
Enumerate Derangements
Automatically use long arrows in display mode
Why do money exchangers give different rates to different bills?
Is Cola "probably the best-known" Latin word in the world? If not, which might it be?
Can the 歳 counter be used for architecture, furniture etc to tell its age?
Why is random forest an improvement of decision tree?
How to reply this mail from potential PhD professor?
Identifying my late father's D&D stuff found in the attic
Is there a legal ground for stripping the UK of its UN Veto if Scotland and/or N.Ireland split from the UK?
How did Arya get her dagger back from Sansa?
Upside-Down Pyramid Addition...REVERSED!
Catholic vs Protestant Support for Nazism in Germany
Classifying ultrasound videos with a small dataset
Binary Classification on small dataset < 200 samplesBinary text classification problem with small label-dataset using kerasPredicting contract churn/cancellation: Great model results does not work in the real worldClassifying with certaintyRNN unable to classify time seriesLong term time series forecasts with small datasetExploratory analysis and feature engineering for time till failure prediction using sensor data of enginesUnsupervised binary classification on small datasetTime series analysis on small datasetSmall dataset in Time series
$begingroup$
I have a small dataset of ~300 ultrasound clips, about evenly divided between 3 classes. Due to the nature of the data (medical) it's difficult to get more.
I need to train a model (or an ensemble) to classify these clips.
Feature reduction is a necessary first step. I've considered using HOGS (for example) on a cropped part of the video to create relatively small set of time-series features.
However, even a "dumbed down" version of HOGS would give at least 100 time series features.
That being said, lets assume for a minute I'm able to reduce the feature space even more while maintaining variability between classes.
Which model(s) would be best suited for this problem?
classification time-series
$endgroup$
add a comment |
$begingroup$
I have a small dataset of ~300 ultrasound clips, about evenly divided between 3 classes. Due to the nature of the data (medical) it's difficult to get more.
I need to train a model (or an ensemble) to classify these clips.
Feature reduction is a necessary first step. I've considered using HOGS (for example) on a cropped part of the video to create relatively small set of time-series features.
However, even a "dumbed down" version of HOGS would give at least 100 time series features.
That being said, lets assume for a minute I'm able to reduce the feature space even more while maintaining variability between classes.
Which model(s) would be best suited for this problem?
classification time-series
$endgroup$
add a comment |
$begingroup$
I have a small dataset of ~300 ultrasound clips, about evenly divided between 3 classes. Due to the nature of the data (medical) it's difficult to get more.
I need to train a model (or an ensemble) to classify these clips.
Feature reduction is a necessary first step. I've considered using HOGS (for example) on a cropped part of the video to create relatively small set of time-series features.
However, even a "dumbed down" version of HOGS would give at least 100 time series features.
That being said, lets assume for a minute I'm able to reduce the feature space even more while maintaining variability between classes.
Which model(s) would be best suited for this problem?
classification time-series
$endgroup$
I have a small dataset of ~300 ultrasound clips, about evenly divided between 3 classes. Due to the nature of the data (medical) it's difficult to get more.
I need to train a model (or an ensemble) to classify these clips.
Feature reduction is a necessary first step. I've considered using HOGS (for example) on a cropped part of the video to create relatively small set of time-series features.
However, even a "dumbed down" version of HOGS would give at least 100 time series features.
That being said, lets assume for a minute I'm able to reduce the feature space even more while maintaining variability between classes.
Which model(s) would be best suited for this problem?
classification time-series
classification time-series
asked Apr 10 at 9:04


IdodoIdodo
101
101
add a comment |
add a comment |
1 Answer
1
active
oldest
votes
$begingroup$
One option in similar problems is to use transfer learning on raw images. For similar problems (few hundred images for training), solution that worked for me is transfer learning + image pre-processing to generate augmented images (E.g.: Stretched / Rotated versions of images).
High level design is :
- Use a model that has been trained on large number of images (millions). This model has already learnt image processing as a domain (such as edge detection, phase / color changes and so on) and might remove need to feature engineering (like HOGS)
- Add few more layers to be trained for Ultrasound images
- Train these new layers with images (Existing layers are frozen since they are already trained)
Examples of this approach are :
https://www.researchgate.net/publication/311094437_Transfer_Learning_with_Convolutional_Neural_Networks_for_Classification_of_Abdominal_Ultrasound_Images
https://www.ncbi.nlm.nih.gov/pmc/articles/PMC5359213/
https://www.sciencedirect.com/science/article/pii/S2095809918301887
$endgroup$
$begingroup$
Thank you for your answer. We had actually already tried this method and the results were poor. The issue is that even a human doctor is only able to classify these clips based on the whole clip, and not just an image from it. There is significance to the "flow" of the main features in the image. This is the reason I'm intent on doing time-series classification, rather than per image.
$endgroup$
– Idodo
Apr 10 at 12:41
$begingroup$
That might be similar to "action classification in video" problem. You can feed each individual frame to this network and feed output of network to an LSTM. With this, you still get benefit of transfer learning and combine it with time-series / sequence via LSTM.
$endgroup$
– Shamit Verma
Apr 10 at 13:06
add a comment |
Your Answer
StackExchange.ready(function()
var channelOptions =
tags: "".split(" "),
id: "557"
;
initTagRenderer("".split(" "), "".split(" "), channelOptions);
StackExchange.using("externalEditor", function()
// Have to fire editor after snippets, if snippets enabled
if (StackExchange.settings.snippets.snippetsEnabled)
StackExchange.using("snippets", function()
createEditor();
);
else
createEditor();
);
function createEditor()
StackExchange.prepareEditor(
heartbeatType: 'answer',
autoActivateHeartbeat: false,
convertImagesToLinks: false,
noModals: true,
showLowRepImageUploadWarning: true,
reputationToPostImages: null,
bindNavPrevention: true,
postfix: "",
imageUploader:
brandingHtml: "Powered by u003ca class="icon-imgur-white" href="https://imgur.com/"u003eu003c/au003e",
contentPolicyHtml: "User contributions licensed under u003ca href="https://creativecommons.org/licenses/by-sa/3.0/"u003ecc by-sa 3.0 with attribution requiredu003c/au003e u003ca href="https://stackoverflow.com/legal/content-policy"u003e(content policy)u003c/au003e",
allowUrls: true
,
onDemand: true,
discardSelector: ".discard-answer"
,immediatelyShowMarkdownHelp:true
);
);
Sign up or log in
StackExchange.ready(function ()
StackExchange.helpers.onClickDraftSave('#login-link');
);
Sign up using Google
Sign up using Facebook
Sign up using Email and Password
Post as a guest
Required, but never shown
StackExchange.ready(
function ()
StackExchange.openid.initPostLogin('.new-post-login', 'https%3a%2f%2fdatascience.stackexchange.com%2fquestions%2f49030%2fclassifying-ultrasound-videos-with-a-small-dataset%23new-answer', 'question_page');
);
Post as a guest
Required, but never shown
1 Answer
1
active
oldest
votes
1 Answer
1
active
oldest
votes
active
oldest
votes
active
oldest
votes
$begingroup$
One option in similar problems is to use transfer learning on raw images. For similar problems (few hundred images for training), solution that worked for me is transfer learning + image pre-processing to generate augmented images (E.g.: Stretched / Rotated versions of images).
High level design is :
- Use a model that has been trained on large number of images (millions). This model has already learnt image processing as a domain (such as edge detection, phase / color changes and so on) and might remove need to feature engineering (like HOGS)
- Add few more layers to be trained for Ultrasound images
- Train these new layers with images (Existing layers are frozen since they are already trained)
Examples of this approach are :
https://www.researchgate.net/publication/311094437_Transfer_Learning_with_Convolutional_Neural_Networks_for_Classification_of_Abdominal_Ultrasound_Images
https://www.ncbi.nlm.nih.gov/pmc/articles/PMC5359213/
https://www.sciencedirect.com/science/article/pii/S2095809918301887
$endgroup$
$begingroup$
Thank you for your answer. We had actually already tried this method and the results were poor. The issue is that even a human doctor is only able to classify these clips based on the whole clip, and not just an image from it. There is significance to the "flow" of the main features in the image. This is the reason I'm intent on doing time-series classification, rather than per image.
$endgroup$
– Idodo
Apr 10 at 12:41
$begingroup$
That might be similar to "action classification in video" problem. You can feed each individual frame to this network and feed output of network to an LSTM. With this, you still get benefit of transfer learning and combine it with time-series / sequence via LSTM.
$endgroup$
– Shamit Verma
Apr 10 at 13:06
add a comment |
$begingroup$
One option in similar problems is to use transfer learning on raw images. For similar problems (few hundred images for training), solution that worked for me is transfer learning + image pre-processing to generate augmented images (E.g.: Stretched / Rotated versions of images).
High level design is :
- Use a model that has been trained on large number of images (millions). This model has already learnt image processing as a domain (such as edge detection, phase / color changes and so on) and might remove need to feature engineering (like HOGS)
- Add few more layers to be trained for Ultrasound images
- Train these new layers with images (Existing layers are frozen since they are already trained)
Examples of this approach are :
https://www.researchgate.net/publication/311094437_Transfer_Learning_with_Convolutional_Neural_Networks_for_Classification_of_Abdominal_Ultrasound_Images
https://www.ncbi.nlm.nih.gov/pmc/articles/PMC5359213/
https://www.sciencedirect.com/science/article/pii/S2095809918301887
$endgroup$
$begingroup$
Thank you for your answer. We had actually already tried this method and the results were poor. The issue is that even a human doctor is only able to classify these clips based on the whole clip, and not just an image from it. There is significance to the "flow" of the main features in the image. This is the reason I'm intent on doing time-series classification, rather than per image.
$endgroup$
– Idodo
Apr 10 at 12:41
$begingroup$
That might be similar to "action classification in video" problem. You can feed each individual frame to this network and feed output of network to an LSTM. With this, you still get benefit of transfer learning and combine it with time-series / sequence via LSTM.
$endgroup$
– Shamit Verma
Apr 10 at 13:06
add a comment |
$begingroup$
One option in similar problems is to use transfer learning on raw images. For similar problems (few hundred images for training), solution that worked for me is transfer learning + image pre-processing to generate augmented images (E.g.: Stretched / Rotated versions of images).
High level design is :
- Use a model that has been trained on large number of images (millions). This model has already learnt image processing as a domain (such as edge detection, phase / color changes and so on) and might remove need to feature engineering (like HOGS)
- Add few more layers to be trained for Ultrasound images
- Train these new layers with images (Existing layers are frozen since they are already trained)
Examples of this approach are :
https://www.researchgate.net/publication/311094437_Transfer_Learning_with_Convolutional_Neural_Networks_for_Classification_of_Abdominal_Ultrasound_Images
https://www.ncbi.nlm.nih.gov/pmc/articles/PMC5359213/
https://www.sciencedirect.com/science/article/pii/S2095809918301887
$endgroup$
One option in similar problems is to use transfer learning on raw images. For similar problems (few hundred images for training), solution that worked for me is transfer learning + image pre-processing to generate augmented images (E.g.: Stretched / Rotated versions of images).
High level design is :
- Use a model that has been trained on large number of images (millions). This model has already learnt image processing as a domain (such as edge detection, phase / color changes and so on) and might remove need to feature engineering (like HOGS)
- Add few more layers to be trained for Ultrasound images
- Train these new layers with images (Existing layers are frozen since they are already trained)
Examples of this approach are :
https://www.researchgate.net/publication/311094437_Transfer_Learning_with_Convolutional_Neural_Networks_for_Classification_of_Abdominal_Ultrasound_Images
https://www.ncbi.nlm.nih.gov/pmc/articles/PMC5359213/
https://www.sciencedirect.com/science/article/pii/S2095809918301887
answered Apr 10 at 12:22
Shamit VermaShamit Verma
1,6891414
1,6891414
$begingroup$
Thank you for your answer. We had actually already tried this method and the results were poor. The issue is that even a human doctor is only able to classify these clips based on the whole clip, and not just an image from it. There is significance to the "flow" of the main features in the image. This is the reason I'm intent on doing time-series classification, rather than per image.
$endgroup$
– Idodo
Apr 10 at 12:41
$begingroup$
That might be similar to "action classification in video" problem. You can feed each individual frame to this network and feed output of network to an LSTM. With this, you still get benefit of transfer learning and combine it with time-series / sequence via LSTM.
$endgroup$
– Shamit Verma
Apr 10 at 13:06
add a comment |
$begingroup$
Thank you for your answer. We had actually already tried this method and the results were poor. The issue is that even a human doctor is only able to classify these clips based on the whole clip, and not just an image from it. There is significance to the "flow" of the main features in the image. This is the reason I'm intent on doing time-series classification, rather than per image.
$endgroup$
– Idodo
Apr 10 at 12:41
$begingroup$
That might be similar to "action classification in video" problem. You can feed each individual frame to this network and feed output of network to an LSTM. With this, you still get benefit of transfer learning and combine it with time-series / sequence via LSTM.
$endgroup$
– Shamit Verma
Apr 10 at 13:06
$begingroup$
Thank you for your answer. We had actually already tried this method and the results were poor. The issue is that even a human doctor is only able to classify these clips based on the whole clip, and not just an image from it. There is significance to the "flow" of the main features in the image. This is the reason I'm intent on doing time-series classification, rather than per image.
$endgroup$
– Idodo
Apr 10 at 12:41
$begingroup$
Thank you for your answer. We had actually already tried this method and the results were poor. The issue is that even a human doctor is only able to classify these clips based on the whole clip, and not just an image from it. There is significance to the "flow" of the main features in the image. This is the reason I'm intent on doing time-series classification, rather than per image.
$endgroup$
– Idodo
Apr 10 at 12:41
$begingroup$
That might be similar to "action classification in video" problem. You can feed each individual frame to this network and feed output of network to an LSTM. With this, you still get benefit of transfer learning and combine it with time-series / sequence via LSTM.
$endgroup$
– Shamit Verma
Apr 10 at 13:06
$begingroup$
That might be similar to "action classification in video" problem. You can feed each individual frame to this network and feed output of network to an LSTM. With this, you still get benefit of transfer learning and combine it with time-series / sequence via LSTM.
$endgroup$
– Shamit Verma
Apr 10 at 13:06
add a comment |
Thanks for contributing an answer to Data Science Stack Exchange!
- Please be sure to answer the question. Provide details and share your research!
But avoid …
- Asking for help, clarification, or responding to other answers.
- Making statements based on opinion; back them up with references or personal experience.
Use MathJax to format equations. MathJax reference.
To learn more, see our tips on writing great answers.
Sign up or log in
StackExchange.ready(function ()
StackExchange.helpers.onClickDraftSave('#login-link');
);
Sign up using Google
Sign up using Facebook
Sign up using Email and Password
Post as a guest
Required, but never shown
StackExchange.ready(
function ()
StackExchange.openid.initPostLogin('.new-post-login', 'https%3a%2f%2fdatascience.stackexchange.com%2fquestions%2f49030%2fclassifying-ultrasound-videos-with-a-small-dataset%23new-answer', 'question_page');
);
Post as a guest
Required, but never shown
Sign up or log in
StackExchange.ready(function ()
StackExchange.helpers.onClickDraftSave('#login-link');
);
Sign up using Google
Sign up using Facebook
Sign up using Email and Password
Post as a guest
Required, but never shown
Sign up or log in
StackExchange.ready(function ()
StackExchange.helpers.onClickDraftSave('#login-link');
);
Sign up using Google
Sign up using Facebook
Sign up using Email and Password
Post as a guest
Required, but never shown
Sign up or log in
StackExchange.ready(function ()
StackExchange.helpers.onClickDraftSave('#login-link');
);
Sign up using Google
Sign up using Facebook
Sign up using Email and Password
Sign up using Google
Sign up using Facebook
Sign up using Email and Password
Post as a guest
Required, but never shown
Required, but never shown
Required, but never shown
Required, but never shown
Required, but never shown
Required, but never shown
Required, but never shown
Required, but never shown
Required, but never shown
9qAHKMNjAnazzrcNPzylm6RcP,IbJMatpQ71dPHKgZgs Hy36X,CNtLOwm2,ojt NWGzd9rOfpM7XyCyepp,F6t