Understanding Youtube recommender (candidate generation step) The Next CEO of Stack Overflow2019 Community Moderator ElectionHow to create a multi-dimensional softmax output in Tensorflow?Skip gram Word2Vec model, neural network implementationHow to create a multi-dimensional softmax output in Tensorflow?How can you decide the window size on a pooling layer?Tensorflow regression model giving same prediction every timeAlternatives to linear activation function in regression tasks to limit the outputRecommender system for next career stepHow to dual encode two sentences to show similarity scoreMultiple-input multiple-output CNN with custom loss functionArchitecture help for multivariate input and output LSTM modelsWhat kinds of math do I need to know to construct graph that preserve its directed simplicies at each time step?
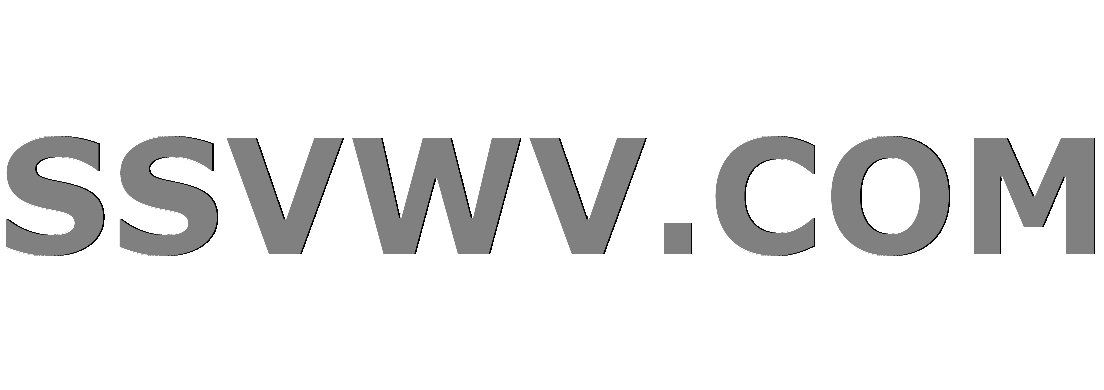
Multi tool use
Can MTA send mail via a relay without being told so?
Is it my responsibility to learn a new technology in my own time my employer wants to implement?
What can we do to stop prior company from asking us questions?
Why does standard notation not preserve intervals (visually)
How to invert MapIndexed on a ragged structure? How to construct a tree from rules?
Is wanting to ask what to write an indication that you need to change your story?
How did people program for Consoles with multiple CPUs?
Won the lottery - how do I keep the money?
What is the value of α and β in a triangle?
How does Madhvacharya interpret Bhagavad Gita sloka 18.66?
Would a completely good Muggle be able to use a wand?
Is it possible to replace duplicates of a character with one character using tr
What benefits would be gained by using human laborers instead of drones in deep sea mining?
Why do airplanes bank sharply to the right after air-to-air refueling?
How to prevent changing the value of variable
WOW air has ceased operation, can I get my tickets refunded?
Why, when going from special to general relativity, do we just replace partial derivatives with covariant derivatives?
Is 'diverse range' a pleonastic phrase?
Is it professional to write unrelated content in an almost-empty email?
How should I support this large drywall patch?
What is the purpose of the Evocation wizard's Potent Cantrip feature?
Does increasing your ability score affect your main stat?
Which one is the true statement?
Beveled cylinder cutout
Understanding Youtube recommender (candidate generation step)
The Next CEO of Stack Overflow2019 Community Moderator ElectionHow to create a multi-dimensional softmax output in Tensorflow?Skip gram Word2Vec model, neural network implementationHow to create a multi-dimensional softmax output in Tensorflow?How can you decide the window size on a pooling layer?Tensorflow regression model giving same prediction every timeAlternatives to linear activation function in regression tasks to limit the outputRecommender system for next career stepHow to dual encode two sentences to show similarity scoreMultiple-input multiple-output CNN with custom loss functionArchitecture help for multivariate input and output LSTM modelsWhat kinds of math do I need to know to construct graph that preserve its directed simplicies at each time step?
$begingroup$
I'm trying to understand https://storage.googleapis.com/pub-tools-public-publication-data/pdf/45530.pdf
Their candidate generation step outputs topn items
- via softmax (with negative sampling) at training time .
- via nearestneighbor at serving time.
I guess Vj represents, (from softmax layer to nearest neighbor index)
topn videos you get via softmax, and represent them in the original encoding (same encoding you used for the input (used for embedded video watches))apparently, Vj are in the different encoding from the input encodings.
The softmax layer outputs a multinomial distribution over the same 1M
video classes with a dimension of 256 (which can be thought
of as a separate output video embedding)I'm trying to understand what they mean by interpreting softmax output as a separate output video embedding. I thought softmax layer that outputs 1M classes has dimension of 1M, where does 256 came from? (It's the same question as How to create a multi-dimensional softmax output in Tensorflow? and I don't think it has been answered there..)
user vector u is the output of the final ReLU unit, although I'm not sure what this user vector is used for.
I guess in serving time, to pick the topn for a given user, user vector u is used by nearest-neighbor. But my understanding of nearest-neighbor is for a given vector, it finds nearest vectors in the same dimension. (such as given an movie, find nearest movies). However here, you are given a user and need to find topn videos. How does that work?
My best guess is that, for a given user, u get a user vector as the ReLU output, then find user-user nearest neighbor, and combine their topn items obtained in the training time. But it's just a guess..
deep-learning recommender-system
$endgroup$
add a comment |
$begingroup$
I'm trying to understand https://storage.googleapis.com/pub-tools-public-publication-data/pdf/45530.pdf
Their candidate generation step outputs topn items
- via softmax (with negative sampling) at training time .
- via nearestneighbor at serving time.
I guess Vj represents, (from softmax layer to nearest neighbor index)
topn videos you get via softmax, and represent them in the original encoding (same encoding you used for the input (used for embedded video watches))apparently, Vj are in the different encoding from the input encodings.
The softmax layer outputs a multinomial distribution over the same 1M
video classes with a dimension of 256 (which can be thought
of as a separate output video embedding)I'm trying to understand what they mean by interpreting softmax output as a separate output video embedding. I thought softmax layer that outputs 1M classes has dimension of 1M, where does 256 came from? (It's the same question as How to create a multi-dimensional softmax output in Tensorflow? and I don't think it has been answered there..)
user vector u is the output of the final ReLU unit, although I'm not sure what this user vector is used for.
I guess in serving time, to pick the topn for a given user, user vector u is used by nearest-neighbor. But my understanding of nearest-neighbor is for a given vector, it finds nearest vectors in the same dimension. (such as given an movie, find nearest movies). However here, you are given a user and need to find topn videos. How does that work?
My best guess is that, for a given user, u get a user vector as the ReLU output, then find user-user nearest neighbor, and combine their topn items obtained in the training time. But it's just a guess..
deep-learning recommender-system
$endgroup$
add a comment |
$begingroup$
I'm trying to understand https://storage.googleapis.com/pub-tools-public-publication-data/pdf/45530.pdf
Their candidate generation step outputs topn items
- via softmax (with negative sampling) at training time .
- via nearestneighbor at serving time.
I guess Vj represents, (from softmax layer to nearest neighbor index)
topn videos you get via softmax, and represent them in the original encoding (same encoding you used for the input (used for embedded video watches))apparently, Vj are in the different encoding from the input encodings.
The softmax layer outputs a multinomial distribution over the same 1M
video classes with a dimension of 256 (which can be thought
of as a separate output video embedding)I'm trying to understand what they mean by interpreting softmax output as a separate output video embedding. I thought softmax layer that outputs 1M classes has dimension of 1M, where does 256 came from? (It's the same question as How to create a multi-dimensional softmax output in Tensorflow? and I don't think it has been answered there..)
user vector u is the output of the final ReLU unit, although I'm not sure what this user vector is used for.
I guess in serving time, to pick the topn for a given user, user vector u is used by nearest-neighbor. But my understanding of nearest-neighbor is for a given vector, it finds nearest vectors in the same dimension. (such as given an movie, find nearest movies). However here, you are given a user and need to find topn videos. How does that work?
My best guess is that, for a given user, u get a user vector as the ReLU output, then find user-user nearest neighbor, and combine their topn items obtained in the training time. But it's just a guess..
deep-learning recommender-system
$endgroup$
I'm trying to understand https://storage.googleapis.com/pub-tools-public-publication-data/pdf/45530.pdf
Their candidate generation step outputs topn items
- via softmax (with negative sampling) at training time .
- via nearestneighbor at serving time.
I guess Vj represents, (from softmax layer to nearest neighbor index)
topn videos you get via softmax, and represent them in the original encoding (same encoding you used for the input (used for embedded video watches))apparently, Vj are in the different encoding from the input encodings.
The softmax layer outputs a multinomial distribution over the same 1M
video classes with a dimension of 256 (which can be thought
of as a separate output video embedding)I'm trying to understand what they mean by interpreting softmax output as a separate output video embedding. I thought softmax layer that outputs 1M classes has dimension of 1M, where does 256 came from? (It's the same question as How to create a multi-dimensional softmax output in Tensorflow? and I don't think it has been answered there..)
user vector u is the output of the final ReLU unit, although I'm not sure what this user vector is used for.
I guess in serving time, to pick the topn for a given user, user vector u is used by nearest-neighbor. But my understanding of nearest-neighbor is for a given vector, it finds nearest vectors in the same dimension. (such as given an movie, find nearest movies). However here, you are given a user and need to find topn videos. How does that work?
My best guess is that, for a given user, u get a user vector as the ReLU output, then find user-user nearest neighbor, and combine their topn items obtained in the training time. But it's just a guess..
deep-learning recommender-system
deep-learning recommender-system
asked Mar 23 at 6:26


eugeneeugene
1064
1064
add a comment |
add a comment |
0
active
oldest
votes
Your Answer
StackExchange.ifUsing("editor", function ()
return StackExchange.using("mathjaxEditing", function ()
StackExchange.MarkdownEditor.creationCallbacks.add(function (editor, postfix)
StackExchange.mathjaxEditing.prepareWmdForMathJax(editor, postfix, [["$", "$"], ["\\(","\\)"]]);
);
);
, "mathjax-editing");
StackExchange.ready(function()
var channelOptions =
tags: "".split(" "),
id: "557"
;
initTagRenderer("".split(" "), "".split(" "), channelOptions);
StackExchange.using("externalEditor", function()
// Have to fire editor after snippets, if snippets enabled
if (StackExchange.settings.snippets.snippetsEnabled)
StackExchange.using("snippets", function()
createEditor();
);
else
createEditor();
);
function createEditor()
StackExchange.prepareEditor(
heartbeatType: 'answer',
autoActivateHeartbeat: false,
convertImagesToLinks: false,
noModals: true,
showLowRepImageUploadWarning: true,
reputationToPostImages: null,
bindNavPrevention: true,
postfix: "",
imageUploader:
brandingHtml: "Powered by u003ca class="icon-imgur-white" href="https://imgur.com/"u003eu003c/au003e",
contentPolicyHtml: "User contributions licensed under u003ca href="https://creativecommons.org/licenses/by-sa/3.0/"u003ecc by-sa 3.0 with attribution requiredu003c/au003e u003ca href="https://stackoverflow.com/legal/content-policy"u003e(content policy)u003c/au003e",
allowUrls: true
,
onDemand: true,
discardSelector: ".discard-answer"
,immediatelyShowMarkdownHelp:true
);
);
Sign up or log in
StackExchange.ready(function ()
StackExchange.helpers.onClickDraftSave('#login-link');
);
Sign up using Google
Sign up using Facebook
Sign up using Email and Password
Post as a guest
Required, but never shown
StackExchange.ready(
function ()
StackExchange.openid.initPostLogin('.new-post-login', 'https%3a%2f%2fdatascience.stackexchange.com%2fquestions%2f47824%2funderstanding-youtube-recommender-candidate-generation-step%23new-answer', 'question_page');
);
Post as a guest
Required, but never shown
0
active
oldest
votes
0
active
oldest
votes
active
oldest
votes
active
oldest
votes
Thanks for contributing an answer to Data Science Stack Exchange!
- Please be sure to answer the question. Provide details and share your research!
But avoid …
- Asking for help, clarification, or responding to other answers.
- Making statements based on opinion; back them up with references or personal experience.
Use MathJax to format equations. MathJax reference.
To learn more, see our tips on writing great answers.
Sign up or log in
StackExchange.ready(function ()
StackExchange.helpers.onClickDraftSave('#login-link');
);
Sign up using Google
Sign up using Facebook
Sign up using Email and Password
Post as a guest
Required, but never shown
StackExchange.ready(
function ()
StackExchange.openid.initPostLogin('.new-post-login', 'https%3a%2f%2fdatascience.stackexchange.com%2fquestions%2f47824%2funderstanding-youtube-recommender-candidate-generation-step%23new-answer', 'question_page');
);
Post as a guest
Required, but never shown
Sign up or log in
StackExchange.ready(function ()
StackExchange.helpers.onClickDraftSave('#login-link');
);
Sign up using Google
Sign up using Facebook
Sign up using Email and Password
Post as a guest
Required, but never shown
Sign up or log in
StackExchange.ready(function ()
StackExchange.helpers.onClickDraftSave('#login-link');
);
Sign up using Google
Sign up using Facebook
Sign up using Email and Password
Post as a guest
Required, but never shown
Sign up or log in
StackExchange.ready(function ()
StackExchange.helpers.onClickDraftSave('#login-link');
);
Sign up using Google
Sign up using Facebook
Sign up using Email and Password
Sign up using Google
Sign up using Facebook
Sign up using Email and Password
Post as a guest
Required, but never shown
Required, but never shown
Required, but never shown
Required, but never shown
Required, but never shown
Required, but never shown
Required, but never shown
Required, but never shown
Required, but never shown
2T3eOBO YLsudl