Including identifier in machine learning model as feature vs separate model for every identifierGuidelines for Machine Learning modelDoes variable (feature) selection help machine learning performance?Find optimal P(X|Y) given I have a model that has good performance when trained on P(Y|X)LSTM Feature selection processImbalanced data causing mis-classification on multiclass datasetDetermine useful features for machine learning modelConvert nominal to numeric variables?How to prepare photo data for training model to recognize bowling ball name, brand and manufacturer from photo of bowling ball?How to deal with possible data leakage in time series data?Service Request classification, questionnaire filling and call logging
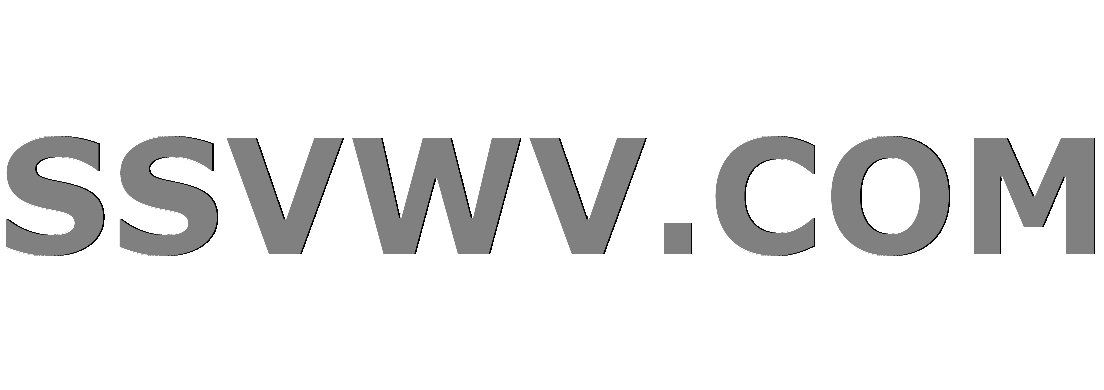
Multi tool use
How are passwords stolen from companies if they only store hashes?
What does "Four-F." mean?
How can an organ that provides biological immortality be unable to regenerate?
Is there a hypothetical scenario that would make Earth uninhabitable for humans, but not for (the majority of) other animals?
How is the partial sum of a geometric sequence calculated?
Suggestions on how to spend Shaabath (constructively) alone
What (if any) is the reason to buy in small local stores?
Is there a term for accumulated dirt on the outside of your hands and feet?
Have the tides ever turned twice on any open problem?
Deletion of copy-ctor & copy-assignment - public, private or protected?
Do I need to be arrogant to get ahead?
Probably overheated black color SMD pads
Maths symbols and unicode-math input inside siunitx commands
Is it possible to stack the damage done by the Absorb Elements spell?
Could Sinn Fein swing any Brexit vote in Parliament?
Knife as defense against stray dogs
Can other pieces capture a threatening piece and prevent a checkmate?
A Ri-diddley-iley Riddle
I seem to dance, I am not a dancer. Who am I?
Word for flower that blooms and wilts in one day
Help rendering a complicated sum/product formula
Turning a hard to access nut?
What is the significance behind "40 days" that often appears in the Bible?
In what cases must I use 了 and in what cases not?
Including identifier in machine learning model as feature vs separate model for every identifier
Guidelines for Machine Learning modelDoes variable (feature) selection help machine learning performance?Find optimal P(X|Y) given I have a model that has good performance when trained on P(Y|X)LSTM Feature selection processImbalanced data causing mis-classification on multiclass datasetDetermine useful features for machine learning modelConvert nominal to numeric variables?How to prepare photo data for training model to recognize bowling ball name, brand and manufacturer from photo of bowling ball?How to deal with possible data leakage in time series data?Service Request classification, questionnaire filling and call logging
$begingroup$
I am new to machine learning and i am building a model to predict number of customers for the model branch at specific hour/season/other feature.
I know it will be bad idea to pit id(branch_id
in my case) into model but customer count in this case hugely depend on which branch it is so i cannot exclude it.
I can think of two solutions, i am not sure which one is right and what is the best practice.
- Create dummy variable(one hot encoding to avoid wieghing one id more than other) for all branch ids,but since i have 600 unique branch ids my features will go up-to
600+rest_of_features
. - Learn a separate model for each of the branch(600 models), i am not sure if it is right approach and also i am not very familiar with this approach and it will be very time consuming.
Looking for the suggestion
Example of the data is below
+-----------+------+-----------+-----------+-------------------+
| branch_id | hour | feature_2 | feature_3 | Count of customer |
+-----------+------+-----------+-----------+-------------------+
| 1 | 12 | .. | .. | 19 |
| 1 | 01 | .. | .. | 25 |
| 2 | 23 | .. | .. | 14 |
| 2 | 01 | .. | .. | 5 |
+-----------+------+-----------+-----------+-------------------+
machine-learning feature-selection
New contributor
mashraf is a new contributor to this site. Take care in asking for clarification, commenting, and answering.
Check out our Code of Conduct.
$endgroup$
add a comment |
$begingroup$
I am new to machine learning and i am building a model to predict number of customers for the model branch at specific hour/season/other feature.
I know it will be bad idea to pit id(branch_id
in my case) into model but customer count in this case hugely depend on which branch it is so i cannot exclude it.
I can think of two solutions, i am not sure which one is right and what is the best practice.
- Create dummy variable(one hot encoding to avoid wieghing one id more than other) for all branch ids,but since i have 600 unique branch ids my features will go up-to
600+rest_of_features
. - Learn a separate model for each of the branch(600 models), i am not sure if it is right approach and also i am not very familiar with this approach and it will be very time consuming.
Looking for the suggestion
Example of the data is below
+-----------+------+-----------+-----------+-------------------+
| branch_id | hour | feature_2 | feature_3 | Count of customer |
+-----------+------+-----------+-----------+-------------------+
| 1 | 12 | .. | .. | 19 |
| 1 | 01 | .. | .. | 25 |
| 2 | 23 | .. | .. | 14 |
| 2 | 01 | .. | .. | 5 |
+-----------+------+-----------+-----------+-------------------+
machine-learning feature-selection
New contributor
mashraf is a new contributor to this site. Take care in asking for clarification, commenting, and answering.
Check out our Code of Conduct.
$endgroup$
add a comment |
$begingroup$
I am new to machine learning and i am building a model to predict number of customers for the model branch at specific hour/season/other feature.
I know it will be bad idea to pit id(branch_id
in my case) into model but customer count in this case hugely depend on which branch it is so i cannot exclude it.
I can think of two solutions, i am not sure which one is right and what is the best practice.
- Create dummy variable(one hot encoding to avoid wieghing one id more than other) for all branch ids,but since i have 600 unique branch ids my features will go up-to
600+rest_of_features
. - Learn a separate model for each of the branch(600 models), i am not sure if it is right approach and also i am not very familiar with this approach and it will be very time consuming.
Looking for the suggestion
Example of the data is below
+-----------+------+-----------+-----------+-------------------+
| branch_id | hour | feature_2 | feature_3 | Count of customer |
+-----------+------+-----------+-----------+-------------------+
| 1 | 12 | .. | .. | 19 |
| 1 | 01 | .. | .. | 25 |
| 2 | 23 | .. | .. | 14 |
| 2 | 01 | .. | .. | 5 |
+-----------+------+-----------+-----------+-------------------+
machine-learning feature-selection
New contributor
mashraf is a new contributor to this site. Take care in asking for clarification, commenting, and answering.
Check out our Code of Conduct.
$endgroup$
I am new to machine learning and i am building a model to predict number of customers for the model branch at specific hour/season/other feature.
I know it will be bad idea to pit id(branch_id
in my case) into model but customer count in this case hugely depend on which branch it is so i cannot exclude it.
I can think of two solutions, i am not sure which one is right and what is the best practice.
- Create dummy variable(one hot encoding to avoid wieghing one id more than other) for all branch ids,but since i have 600 unique branch ids my features will go up-to
600+rest_of_features
. - Learn a separate model for each of the branch(600 models), i am not sure if it is right approach and also i am not very familiar with this approach and it will be very time consuming.
Looking for the suggestion
Example of the data is below
+-----------+------+-----------+-----------+-------------------+
| branch_id | hour | feature_2 | feature_3 | Count of customer |
+-----------+------+-----------+-----------+-------------------+
| 1 | 12 | .. | .. | 19 |
| 1 | 01 | .. | .. | 25 |
| 2 | 23 | .. | .. | 14 |
| 2 | 01 | .. | .. | 5 |
+-----------+------+-----------+-----------+-------------------+
machine-learning feature-selection
machine-learning feature-selection
New contributor
mashraf is a new contributor to this site. Take care in asking for clarification, commenting, and answering.
Check out our Code of Conduct.
New contributor
mashraf is a new contributor to this site. Take care in asking for clarification, commenting, and answering.
Check out our Code of Conduct.
New contributor
mashraf is a new contributor to this site. Take care in asking for clarification, commenting, and answering.
Check out our Code of Conduct.
asked 2 days ago
mashrafmashraf
1
1
New contributor
mashraf is a new contributor to this site. Take care in asking for clarification, commenting, and answering.
Check out our Code of Conduct.
New contributor
mashraf is a new contributor to this site. Take care in asking for clarification, commenting, and answering.
Check out our Code of Conduct.
mashraf is a new contributor to this site. Take care in asking for clarification, commenting, and answering.
Check out our Code of Conduct.
add a comment |
add a comment |
2 Answers
2
active
oldest
votes
$begingroup$
In my opinion including id as a feature will not make sense at all, because the model will treat the id as a numeric value which will decrease the model performance, because there should be no connection how big the id is and how many customers there are for that id.
Option 2 can make sense if you have enough data for every branch.
My suggestion will be to look deep into your features and try to find a feature which will replace the branch id. Let's say the number of supporting desks in a branch or the location of a branch as a categorical value. If you find enough features that can describe the specifics of branches, then no need to include ids or to do it separately.
New contributor
Karen Danielyan is a new contributor to this site. Take care in asking for clarification, commenting, and answering.
Check out our Code of Conduct.
$endgroup$
$begingroup$
thanks for answering, what about "Create dummy variable(one hot encoding) for all branch ids,but since i have 600 unique branch ids my features will go up-to 600+rest_of_features." ?
$endgroup$
– mashraf
yesterday
$begingroup$
Regarding 600 dummy variables: it can practically work with some regressors if you have large enough number of observations. Probably except from decision tree regressors. The typical rule of thumb for the multiple linear regression is usually that the number of observations should be at least 5 times more than the number of variables, otherwise you will have completely insignificant estimates. For your case, I think if you have quite large dataset, then you can try it out, also try different ML algorithms to see which one goes well.
$endgroup$
– Karen Danielyan
11 hours ago
add a comment |
$begingroup$
branch_id
in this case is a categorical variable, and you can treat is just like you would other categoricals (like city: "Seattle", "San Diego", "Austin"). You just need to be sure you use an algorithm that can treat it as categorical. LightGBM uses a method that sorts and optimally splits the histogram of the categorical integers, which is faster than OHE. CatBoost can leverage a few different methods.
In addition to regression, you can similarly convert the customer counts into ranges or histogram bins and use a classification algorithm to predict the bin.
New contributor
wwwslinger is a new contributor to this site. Take care in asking for clarification, commenting, and answering.
Check out our Code of Conduct.
$endgroup$
add a comment |
Your Answer
StackExchange.ifUsing("editor", function ()
return StackExchange.using("mathjaxEditing", function ()
StackExchange.MarkdownEditor.creationCallbacks.add(function (editor, postfix)
StackExchange.mathjaxEditing.prepareWmdForMathJax(editor, postfix, [["$", "$"], ["\\(","\\)"]]);
);
);
, "mathjax-editing");
StackExchange.ready(function()
var channelOptions =
tags: "".split(" "),
id: "557"
;
initTagRenderer("".split(" "), "".split(" "), channelOptions);
StackExchange.using("externalEditor", function()
// Have to fire editor after snippets, if snippets enabled
if (StackExchange.settings.snippets.snippetsEnabled)
StackExchange.using("snippets", function()
createEditor();
);
else
createEditor();
);
function createEditor()
StackExchange.prepareEditor(
heartbeatType: 'answer',
autoActivateHeartbeat: false,
convertImagesToLinks: false,
noModals: true,
showLowRepImageUploadWarning: true,
reputationToPostImages: null,
bindNavPrevention: true,
postfix: "",
imageUploader:
brandingHtml: "Powered by u003ca class="icon-imgur-white" href="https://imgur.com/"u003eu003c/au003e",
contentPolicyHtml: "User contributions licensed under u003ca href="https://creativecommons.org/licenses/by-sa/3.0/"u003ecc by-sa 3.0 with attribution requiredu003c/au003e u003ca href="https://stackoverflow.com/legal/content-policy"u003e(content policy)u003c/au003e",
allowUrls: true
,
onDemand: true,
discardSelector: ".discard-answer"
,immediatelyShowMarkdownHelp:true
);
);
mashraf is a new contributor. Be nice, and check out our Code of Conduct.
Sign up or log in
StackExchange.ready(function ()
StackExchange.helpers.onClickDraftSave('#login-link');
);
Sign up using Google
Sign up using Facebook
Sign up using Email and Password
Post as a guest
Required, but never shown
StackExchange.ready(
function ()
StackExchange.openid.initPostLogin('.new-post-login', 'https%3a%2f%2fdatascience.stackexchange.com%2fquestions%2f47388%2fincluding-identifier-in-machine-learning-model-as-feature-vs-separate-model-for%23new-answer', 'question_page');
);
Post as a guest
Required, but never shown
2 Answers
2
active
oldest
votes
2 Answers
2
active
oldest
votes
active
oldest
votes
active
oldest
votes
$begingroup$
In my opinion including id as a feature will not make sense at all, because the model will treat the id as a numeric value which will decrease the model performance, because there should be no connection how big the id is and how many customers there are for that id.
Option 2 can make sense if you have enough data for every branch.
My suggestion will be to look deep into your features and try to find a feature which will replace the branch id. Let's say the number of supporting desks in a branch or the location of a branch as a categorical value. If you find enough features that can describe the specifics of branches, then no need to include ids or to do it separately.
New contributor
Karen Danielyan is a new contributor to this site. Take care in asking for clarification, commenting, and answering.
Check out our Code of Conduct.
$endgroup$
$begingroup$
thanks for answering, what about "Create dummy variable(one hot encoding) for all branch ids,but since i have 600 unique branch ids my features will go up-to 600+rest_of_features." ?
$endgroup$
– mashraf
yesterday
$begingroup$
Regarding 600 dummy variables: it can practically work with some regressors if you have large enough number of observations. Probably except from decision tree regressors. The typical rule of thumb for the multiple linear regression is usually that the number of observations should be at least 5 times more than the number of variables, otherwise you will have completely insignificant estimates. For your case, I think if you have quite large dataset, then you can try it out, also try different ML algorithms to see which one goes well.
$endgroup$
– Karen Danielyan
11 hours ago
add a comment |
$begingroup$
In my opinion including id as a feature will not make sense at all, because the model will treat the id as a numeric value which will decrease the model performance, because there should be no connection how big the id is and how many customers there are for that id.
Option 2 can make sense if you have enough data for every branch.
My suggestion will be to look deep into your features and try to find a feature which will replace the branch id. Let's say the number of supporting desks in a branch or the location of a branch as a categorical value. If you find enough features that can describe the specifics of branches, then no need to include ids or to do it separately.
New contributor
Karen Danielyan is a new contributor to this site. Take care in asking for clarification, commenting, and answering.
Check out our Code of Conduct.
$endgroup$
$begingroup$
thanks for answering, what about "Create dummy variable(one hot encoding) for all branch ids,but since i have 600 unique branch ids my features will go up-to 600+rest_of_features." ?
$endgroup$
– mashraf
yesterday
$begingroup$
Regarding 600 dummy variables: it can practically work with some regressors if you have large enough number of observations. Probably except from decision tree regressors. The typical rule of thumb for the multiple linear regression is usually that the number of observations should be at least 5 times more than the number of variables, otherwise you will have completely insignificant estimates. For your case, I think if you have quite large dataset, then you can try it out, also try different ML algorithms to see which one goes well.
$endgroup$
– Karen Danielyan
11 hours ago
add a comment |
$begingroup$
In my opinion including id as a feature will not make sense at all, because the model will treat the id as a numeric value which will decrease the model performance, because there should be no connection how big the id is and how many customers there are for that id.
Option 2 can make sense if you have enough data for every branch.
My suggestion will be to look deep into your features and try to find a feature which will replace the branch id. Let's say the number of supporting desks in a branch or the location of a branch as a categorical value. If you find enough features that can describe the specifics of branches, then no need to include ids or to do it separately.
New contributor
Karen Danielyan is a new contributor to this site. Take care in asking for clarification, commenting, and answering.
Check out our Code of Conduct.
$endgroup$
In my opinion including id as a feature will not make sense at all, because the model will treat the id as a numeric value which will decrease the model performance, because there should be no connection how big the id is and how many customers there are for that id.
Option 2 can make sense if you have enough data for every branch.
My suggestion will be to look deep into your features and try to find a feature which will replace the branch id. Let's say the number of supporting desks in a branch or the location of a branch as a categorical value. If you find enough features that can describe the specifics of branches, then no need to include ids or to do it separately.
New contributor
Karen Danielyan is a new contributor to this site. Take care in asking for clarification, commenting, and answering.
Check out our Code of Conduct.
New contributor
Karen Danielyan is a new contributor to this site. Take care in asking for clarification, commenting, and answering.
Check out our Code of Conduct.
answered 2 days ago


Karen DanielyanKaren Danielyan
16
16
New contributor
Karen Danielyan is a new contributor to this site. Take care in asking for clarification, commenting, and answering.
Check out our Code of Conduct.
New contributor
Karen Danielyan is a new contributor to this site. Take care in asking for clarification, commenting, and answering.
Check out our Code of Conduct.
Karen Danielyan is a new contributor to this site. Take care in asking for clarification, commenting, and answering.
Check out our Code of Conduct.
$begingroup$
thanks for answering, what about "Create dummy variable(one hot encoding) for all branch ids,but since i have 600 unique branch ids my features will go up-to 600+rest_of_features." ?
$endgroup$
– mashraf
yesterday
$begingroup$
Regarding 600 dummy variables: it can practically work with some regressors if you have large enough number of observations. Probably except from decision tree regressors. The typical rule of thumb for the multiple linear regression is usually that the number of observations should be at least 5 times more than the number of variables, otherwise you will have completely insignificant estimates. For your case, I think if you have quite large dataset, then you can try it out, also try different ML algorithms to see which one goes well.
$endgroup$
– Karen Danielyan
11 hours ago
add a comment |
$begingroup$
thanks for answering, what about "Create dummy variable(one hot encoding) for all branch ids,but since i have 600 unique branch ids my features will go up-to 600+rest_of_features." ?
$endgroup$
– mashraf
yesterday
$begingroup$
Regarding 600 dummy variables: it can practically work with some regressors if you have large enough number of observations. Probably except from decision tree regressors. The typical rule of thumb for the multiple linear regression is usually that the number of observations should be at least 5 times more than the number of variables, otherwise you will have completely insignificant estimates. For your case, I think if you have quite large dataset, then you can try it out, also try different ML algorithms to see which one goes well.
$endgroup$
– Karen Danielyan
11 hours ago
$begingroup$
thanks for answering, what about "Create dummy variable(one hot encoding) for all branch ids,but since i have 600 unique branch ids my features will go up-to 600+rest_of_features." ?
$endgroup$
– mashraf
yesterday
$begingroup$
thanks for answering, what about "Create dummy variable(one hot encoding) for all branch ids,but since i have 600 unique branch ids my features will go up-to 600+rest_of_features." ?
$endgroup$
– mashraf
yesterday
$begingroup$
Regarding 600 dummy variables: it can practically work with some regressors if you have large enough number of observations. Probably except from decision tree regressors. The typical rule of thumb for the multiple linear regression is usually that the number of observations should be at least 5 times more than the number of variables, otherwise you will have completely insignificant estimates. For your case, I think if you have quite large dataset, then you can try it out, also try different ML algorithms to see which one goes well.
$endgroup$
– Karen Danielyan
11 hours ago
$begingroup$
Regarding 600 dummy variables: it can practically work with some regressors if you have large enough number of observations. Probably except from decision tree regressors. The typical rule of thumb for the multiple linear regression is usually that the number of observations should be at least 5 times more than the number of variables, otherwise you will have completely insignificant estimates. For your case, I think if you have quite large dataset, then you can try it out, also try different ML algorithms to see which one goes well.
$endgroup$
– Karen Danielyan
11 hours ago
add a comment |
$begingroup$
branch_id
in this case is a categorical variable, and you can treat is just like you would other categoricals (like city: "Seattle", "San Diego", "Austin"). You just need to be sure you use an algorithm that can treat it as categorical. LightGBM uses a method that sorts and optimally splits the histogram of the categorical integers, which is faster than OHE. CatBoost can leverage a few different methods.
In addition to regression, you can similarly convert the customer counts into ranges or histogram bins and use a classification algorithm to predict the bin.
New contributor
wwwslinger is a new contributor to this site. Take care in asking for clarification, commenting, and answering.
Check out our Code of Conduct.
$endgroup$
add a comment |
$begingroup$
branch_id
in this case is a categorical variable, and you can treat is just like you would other categoricals (like city: "Seattle", "San Diego", "Austin"). You just need to be sure you use an algorithm that can treat it as categorical. LightGBM uses a method that sorts and optimally splits the histogram of the categorical integers, which is faster than OHE. CatBoost can leverage a few different methods.
In addition to regression, you can similarly convert the customer counts into ranges or histogram bins and use a classification algorithm to predict the bin.
New contributor
wwwslinger is a new contributor to this site. Take care in asking for clarification, commenting, and answering.
Check out our Code of Conduct.
$endgroup$
add a comment |
$begingroup$
branch_id
in this case is a categorical variable, and you can treat is just like you would other categoricals (like city: "Seattle", "San Diego", "Austin"). You just need to be sure you use an algorithm that can treat it as categorical. LightGBM uses a method that sorts and optimally splits the histogram of the categorical integers, which is faster than OHE. CatBoost can leverage a few different methods.
In addition to regression, you can similarly convert the customer counts into ranges or histogram bins and use a classification algorithm to predict the bin.
New contributor
wwwslinger is a new contributor to this site. Take care in asking for clarification, commenting, and answering.
Check out our Code of Conduct.
$endgroup$
branch_id
in this case is a categorical variable, and you can treat is just like you would other categoricals (like city: "Seattle", "San Diego", "Austin"). You just need to be sure you use an algorithm that can treat it as categorical. LightGBM uses a method that sorts and optimally splits the histogram of the categorical integers, which is faster than OHE. CatBoost can leverage a few different methods.
In addition to regression, you can similarly convert the customer counts into ranges or histogram bins and use a classification algorithm to predict the bin.
New contributor
wwwslinger is a new contributor to this site. Take care in asking for clarification, commenting, and answering.
Check out our Code of Conduct.
New contributor
wwwslinger is a new contributor to this site. Take care in asking for clarification, commenting, and answering.
Check out our Code of Conduct.
answered 2 days ago
wwwslingerwwwslinger
1183
1183
New contributor
wwwslinger is a new contributor to this site. Take care in asking for clarification, commenting, and answering.
Check out our Code of Conduct.
New contributor
wwwslinger is a new contributor to this site. Take care in asking for clarification, commenting, and answering.
Check out our Code of Conduct.
wwwslinger is a new contributor to this site. Take care in asking for clarification, commenting, and answering.
Check out our Code of Conduct.
add a comment |
add a comment |
mashraf is a new contributor. Be nice, and check out our Code of Conduct.
mashraf is a new contributor. Be nice, and check out our Code of Conduct.
mashraf is a new contributor. Be nice, and check out our Code of Conduct.
mashraf is a new contributor. Be nice, and check out our Code of Conduct.
Thanks for contributing an answer to Data Science Stack Exchange!
- Please be sure to answer the question. Provide details and share your research!
But avoid …
- Asking for help, clarification, or responding to other answers.
- Making statements based on opinion; back them up with references or personal experience.
Use MathJax to format equations. MathJax reference.
To learn more, see our tips on writing great answers.
Sign up or log in
StackExchange.ready(function ()
StackExchange.helpers.onClickDraftSave('#login-link');
);
Sign up using Google
Sign up using Facebook
Sign up using Email and Password
Post as a guest
Required, but never shown
StackExchange.ready(
function ()
StackExchange.openid.initPostLogin('.new-post-login', 'https%3a%2f%2fdatascience.stackexchange.com%2fquestions%2f47388%2fincluding-identifier-in-machine-learning-model-as-feature-vs-separate-model-for%23new-answer', 'question_page');
);
Post as a guest
Required, but never shown
Sign up or log in
StackExchange.ready(function ()
StackExchange.helpers.onClickDraftSave('#login-link');
);
Sign up using Google
Sign up using Facebook
Sign up using Email and Password
Post as a guest
Required, but never shown
Sign up or log in
StackExchange.ready(function ()
StackExchange.helpers.onClickDraftSave('#login-link');
);
Sign up using Google
Sign up using Facebook
Sign up using Email and Password
Post as a guest
Required, but never shown
Sign up or log in
StackExchange.ready(function ()
StackExchange.helpers.onClickDraftSave('#login-link');
);
Sign up using Google
Sign up using Facebook
Sign up using Email and Password
Sign up using Google
Sign up using Facebook
Sign up using Email and Password
Post as a guest
Required, but never shown
Required, but never shown
Required, but never shown
Required, but never shown
Required, but never shown
Required, but never shown
Required, but never shown
Required, but never shown
Required, but never shown
6tRlYq