Does gradient descent always converge to an optimum? Announcing the arrival of Valued Associate #679: Cesar Manara Planned maintenance scheduled April 23, 2019 at 23:30 UTC (7:30pm US/Eastern) 2019 Moderator Election Q&A - Questionnaire 2019 Community Moderator Election Resultslocal minima vs saddle points in deep learningHow to plot cost versus number of iterations in scikit learn?Does MLP always find local minimumWhat are the cases where it is fine to initialize all weights to zeroThe connection between optimization and generalizationMeaning of Perceptron optimal weightsWhy Gradient methods work in finding the parameters in Neural Networks?Optimization methods used in machine learningMinimization algorithm that can consider gradient close to solutionGradient Descent ConvergenceStochastic gradient descent in logistic regressionStochastic gradient descent and different approachesWhy is vanishing gradient a problem?Optimization methods used in machine learningAdam optimizer for projected gradient descentUsing Mean Squared Error in Gradient DescentShould the minimum value of a cost (loss) function be equal to zero?How to get out of local minimums on stochastic gradient descent?
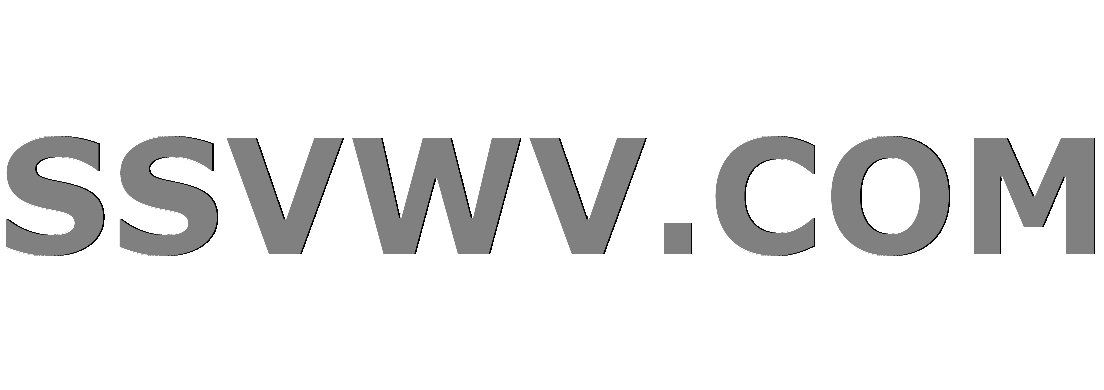
Multi tool use
"Destructive power" carried by a B-52?
Did any compiler fully use 80-bit floating point?
How could a hydrazine and N2O4 cloud (or it's reactants) show up in weather radar?
What is "Lambda" in Heston's original paper on stochastic volatility models?
Can two people see the same photon?
Table formatting with tabularx?
Does the main washing effect of soap come from foam?
How to name indistinguishable henchmen in a screenplay?
Did pre-Columbian Americans know the spherical shape of the Earth?
Understanding piped commands in GNU/Linux
Random body shuffle every night—can we still function?
Why does BitLocker not use RSA?
Determine whether an integer is a palindrome
Diophantine equation 3^a+1=3^b+5^c
How can I prevent/balance waiting and turtling as a response to cooldown mechanics
newbie Q : How to read an output file in one command line
Keep at all times, the minus sign above aligned with minus sign below
Where and when has Thucydides been studied?
Pointing to problems without suggesting solutions
Flight departed from the gate 5 min before scheduled departure time. Refund options
How to achieve cat-like agility?
My mentor says to set image to Fine instead of RAW — how is this different from JPG?
First paper to introduce the "principal-agent problem"
One-one communication
Does gradient descent always converge to an optimum?
Announcing the arrival of Valued Associate #679: Cesar Manara
Planned maintenance scheduled April 23, 2019 at 23:30 UTC (7:30pm US/Eastern)
2019 Moderator Election Q&A - Questionnaire
2019 Community Moderator Election Resultslocal minima vs saddle points in deep learningHow to plot cost versus number of iterations in scikit learn?Does MLP always find local minimumWhat are the cases where it is fine to initialize all weights to zeroThe connection between optimization and generalizationMeaning of Perceptron optimal weightsWhy Gradient methods work in finding the parameters in Neural Networks?Optimization methods used in machine learningMinimization algorithm that can consider gradient close to solutionGradient Descent ConvergenceStochastic gradient descent in logistic regressionStochastic gradient descent and different approachesWhy is vanishing gradient a problem?Optimization methods used in machine learningAdam optimizer for projected gradient descentUsing Mean Squared Error in Gradient DescentShould the minimum value of a cost (loss) function be equal to zero?How to get out of local minimums on stochastic gradient descent?
$begingroup$
I am wondering whether there is any scenario in which gradient descent does not converge to a minimum.
I am aware that gradient descent is not always guaranteed to converge to a global optimum. I am also aware that it might diverge from an optimum if, say, the step size is too big. However, it seems to me that, if it diverges from some optimum, then it will eventually go to another optimum.
Hence, gradient descent would be guaranteed to converge to a local or global optimum. Is that right? If not, could you please provide a rough counterexample?
machine-learning neural-network deep-learning optimization gradient-descent
$endgroup$
add a comment |
$begingroup$
I am wondering whether there is any scenario in which gradient descent does not converge to a minimum.
I am aware that gradient descent is not always guaranteed to converge to a global optimum. I am also aware that it might diverge from an optimum if, say, the step size is too big. However, it seems to me that, if it diverges from some optimum, then it will eventually go to another optimum.
Hence, gradient descent would be guaranteed to converge to a local or global optimum. Is that right? If not, could you please provide a rough counterexample?
machine-learning neural-network deep-learning optimization gradient-descent
$endgroup$
$begingroup$
Hope this link will help in future..datascience.stackexchange.com/a/28417/35644
$endgroup$
– Aditya
Mar 3 '18 at 17:31
$begingroup$
See this answer for 3 concrete and simple examples, including proofs, images and code that creates an animation of the gradient descent
$endgroup$
– Oren Milman
Aug 27 '18 at 7:38
add a comment |
$begingroup$
I am wondering whether there is any scenario in which gradient descent does not converge to a minimum.
I am aware that gradient descent is not always guaranteed to converge to a global optimum. I am also aware that it might diverge from an optimum if, say, the step size is too big. However, it seems to me that, if it diverges from some optimum, then it will eventually go to another optimum.
Hence, gradient descent would be guaranteed to converge to a local or global optimum. Is that right? If not, could you please provide a rough counterexample?
machine-learning neural-network deep-learning optimization gradient-descent
$endgroup$
I am wondering whether there is any scenario in which gradient descent does not converge to a minimum.
I am aware that gradient descent is not always guaranteed to converge to a global optimum. I am also aware that it might diverge from an optimum if, say, the step size is too big. However, it seems to me that, if it diverges from some optimum, then it will eventually go to another optimum.
Hence, gradient descent would be guaranteed to converge to a local or global optimum. Is that right? If not, could you please provide a rough counterexample?
machine-learning neural-network deep-learning optimization gradient-descent
machine-learning neural-network deep-learning optimization gradient-descent
edited Jan 10 '18 at 16:55


Vaalizaadeh
7,65562265
7,65562265
asked Nov 9 '17 at 16:41
wit221wit221
183115
183115
$begingroup$
Hope this link will help in future..datascience.stackexchange.com/a/28417/35644
$endgroup$
– Aditya
Mar 3 '18 at 17:31
$begingroup$
See this answer for 3 concrete and simple examples, including proofs, images and code that creates an animation of the gradient descent
$endgroup$
– Oren Milman
Aug 27 '18 at 7:38
add a comment |
$begingroup$
Hope this link will help in future..datascience.stackexchange.com/a/28417/35644
$endgroup$
– Aditya
Mar 3 '18 at 17:31
$begingroup$
See this answer for 3 concrete and simple examples, including proofs, images and code that creates an animation of the gradient descent
$endgroup$
– Oren Milman
Aug 27 '18 at 7:38
$begingroup$
Hope this link will help in future..datascience.stackexchange.com/a/28417/35644
$endgroup$
– Aditya
Mar 3 '18 at 17:31
$begingroup$
Hope this link will help in future..datascience.stackexchange.com/a/28417/35644
$endgroup$
– Aditya
Mar 3 '18 at 17:31
$begingroup$
See this answer for 3 concrete and simple examples, including proofs, images and code that creates an animation of the gradient descent
$endgroup$
– Oren Milman
Aug 27 '18 at 7:38
$begingroup$
See this answer for 3 concrete and simple examples, including proofs, images and code that creates an animation of the gradient descent
$endgroup$
– Oren Milman
Aug 27 '18 at 7:38
add a comment |
4 Answers
4
active
oldest
votes
$begingroup$
Gradient Descent is an algorithm which is designed to find the optimal points, but these optimal points are not necessarily global. And yes if it happens that it diverges from a local location it may converge to another optimal point but its probability is not too much. The reason is that the step size might be too large that prompts it recede one optimal point and the probability that it oscillates is much more than convergence.
About gradient descent there are two main perspectives, machine learning era and deep learning era. During machine learning era it was considered that gradient descent will find the local/global optimum but in deep learning era where the dimension of input features are too much it is shown in practice that the probability that all of the features be located in there optimal value at a single point is not too much and rather seeing to have optimal locations in cost functions, most of the time saddle points are observed. This is one of the reasons that training with lots of data and training epochs cause the deep learning models outperform other algorithms. So if you train your model, it will find a detour or will find its way to go downhill and do not stuck in saddle points, but you have to have appropriate step sizes.
For more intuitions I suggest you referring here and here.
$endgroup$
1
$begingroup$
Exactly. These problems always pop up in theory, but rarely in actual practice. With so many dimensions, this isn't an issue. You'll have a local minima in one variable, but not in another. Furthermore, mini-batch or stochastic gradient descent ensures also help avoiding any local minima.
$endgroup$
– Ricardo Cruz
Nov 16 '17 at 17:38
2
$begingroup$
@RicardoCruz yes, I do agree sir
$endgroup$
– Vaalizaadeh
Nov 16 '17 at 20:30
add a comment |
$begingroup$
Asides from the points you mentioned (convergence to non-global minimums, and large step sizes possibly leading to non-convergent algorithms), "inflection ranges" might be a problem too.
Consider the following "recliner chair" type of function.
Obviously, this can be constructed so that there is a range in the middle where the gradient is the 0 vector. In this range, the algorithm can be stuck indefinitely. Inflection points are usually not considered local extrema.
$endgroup$
add a comment |
$begingroup$
Conjugate gradient is not guaranteed to reach a global optimum or a local optimum! There are points where the gradient is very small, that are not optima (inflection points, saddle points). Gradient Descent could converge to a point $x = 0$ for the function $f(x) = x^3$.
$endgroup$
add a comment |
$begingroup$
[Note 5 April 2019: A new version of the paper has been updated on arXiv with many new results. We introduce also backtracking versions of Momentum and NAG, and prove convergence under the same assumptions as for Backtracking Gradient Descent.
Source codes are available on GitHub at the link: https://github.com/hank-nguyen/MBT-optimizer
We improved the algorithms for applying to DNN, and obtain better performance than state-of-the-art algorithms such as MMT, NAG, Adam, Adamax, Adagrad,...
The most special feature of our algorithms are that they are automatic, you do not need to do manual fine-tuning of learning rates as common practice. Our automatic fine-tuning is different in nature from Adam, Adamax, Adagrad,... and so on. More details are in the paper.
]
Based on very recent results: In my joint work in this paper https://arxiv.org/abs/1808.05160
We showed that backtracking gradient descent, when applied to an arbitrary C^1 function $f$, with only a countable number of critical points, will always either converge to a critical point or diverge to infinity. This condition is satisfied for a generic function, for example for all Morse functions. We also showed that in a sense it is very rare for the limit point to be a saddle point. So if all of your critical points are non-degenerate, then in a certain sense the limit points are all minimums. [Please see also references in the cited paper for the known results in the case of the standard gradient descent.]
Based on the above, we proposed a new method in deep learning which is on par with current state-of-the-art methods and does not need manual fine-tuning of the learning rates. (In a nutshell, the idea is that you run backtracking gradient descent a certain amount of time, until you see that the learning rates, which change with each iteration, become stabilise. We expect this stabilisation, in particular at a critical point which is C^2 and is non-degenerate, because of the convergence result I mentioned above. At that point, you switch to the standard gradient descent method. Please see the cited paper for more detail. This method can also be applied to other optimal algorithms.)
P.S. Regarding your original question about the standard gradient descent method, to my knowledge only in the case where the derivative of the map is globally Lipschitz and the learning rate is small enough that the standard gradient descent method is proven to converge. [If these conditions are not satisfied, there are simple counter-examples showing that no convergence result is possible, see the cited paper for some.] In the paper cited above, we argued that in the long run the backtracking gradient descent method will become the standard gradient descent method, which gives an explanation why the standard gradient descent method usually works well in practice.
$endgroup$
add a comment |
Your Answer
StackExchange.ready(function()
var channelOptions =
tags: "".split(" "),
id: "557"
;
initTagRenderer("".split(" "), "".split(" "), channelOptions);
StackExchange.using("externalEditor", function()
// Have to fire editor after snippets, if snippets enabled
if (StackExchange.settings.snippets.snippetsEnabled)
StackExchange.using("snippets", function()
createEditor();
);
else
createEditor();
);
function createEditor()
StackExchange.prepareEditor(
heartbeatType: 'answer',
autoActivateHeartbeat: false,
convertImagesToLinks: false,
noModals: true,
showLowRepImageUploadWarning: true,
reputationToPostImages: null,
bindNavPrevention: true,
postfix: "",
imageUploader:
brandingHtml: "Powered by u003ca class="icon-imgur-white" href="https://imgur.com/"u003eu003c/au003e",
contentPolicyHtml: "User contributions licensed under u003ca href="https://creativecommons.org/licenses/by-sa/3.0/"u003ecc by-sa 3.0 with attribution requiredu003c/au003e u003ca href="https://stackoverflow.com/legal/content-policy"u003e(content policy)u003c/au003e",
allowUrls: true
,
onDemand: true,
discardSelector: ".discard-answer"
,immediatelyShowMarkdownHelp:true
);
);
Sign up or log in
StackExchange.ready(function ()
StackExchange.helpers.onClickDraftSave('#login-link');
);
Sign up using Google
Sign up using Facebook
Sign up using Email and Password
Post as a guest
Required, but never shown
StackExchange.ready(
function ()
StackExchange.openid.initPostLogin('.new-post-login', 'https%3a%2f%2fdatascience.stackexchange.com%2fquestions%2f24534%2fdoes-gradient-descent-always-converge-to-an-optimum%23new-answer', 'question_page');
);
Post as a guest
Required, but never shown
4 Answers
4
active
oldest
votes
4 Answers
4
active
oldest
votes
active
oldest
votes
active
oldest
votes
$begingroup$
Gradient Descent is an algorithm which is designed to find the optimal points, but these optimal points are not necessarily global. And yes if it happens that it diverges from a local location it may converge to another optimal point but its probability is not too much. The reason is that the step size might be too large that prompts it recede one optimal point and the probability that it oscillates is much more than convergence.
About gradient descent there are two main perspectives, machine learning era and deep learning era. During machine learning era it was considered that gradient descent will find the local/global optimum but in deep learning era where the dimension of input features are too much it is shown in practice that the probability that all of the features be located in there optimal value at a single point is not too much and rather seeing to have optimal locations in cost functions, most of the time saddle points are observed. This is one of the reasons that training with lots of data and training epochs cause the deep learning models outperform other algorithms. So if you train your model, it will find a detour or will find its way to go downhill and do not stuck in saddle points, but you have to have appropriate step sizes.
For more intuitions I suggest you referring here and here.
$endgroup$
1
$begingroup$
Exactly. These problems always pop up in theory, but rarely in actual practice. With so many dimensions, this isn't an issue. You'll have a local minima in one variable, but not in another. Furthermore, mini-batch or stochastic gradient descent ensures also help avoiding any local minima.
$endgroup$
– Ricardo Cruz
Nov 16 '17 at 17:38
2
$begingroup$
@RicardoCruz yes, I do agree sir
$endgroup$
– Vaalizaadeh
Nov 16 '17 at 20:30
add a comment |
$begingroup$
Gradient Descent is an algorithm which is designed to find the optimal points, but these optimal points are not necessarily global. And yes if it happens that it diverges from a local location it may converge to another optimal point but its probability is not too much. The reason is that the step size might be too large that prompts it recede one optimal point and the probability that it oscillates is much more than convergence.
About gradient descent there are two main perspectives, machine learning era and deep learning era. During machine learning era it was considered that gradient descent will find the local/global optimum but in deep learning era where the dimension of input features are too much it is shown in practice that the probability that all of the features be located in there optimal value at a single point is not too much and rather seeing to have optimal locations in cost functions, most of the time saddle points are observed. This is one of the reasons that training with lots of data and training epochs cause the deep learning models outperform other algorithms. So if you train your model, it will find a detour or will find its way to go downhill and do not stuck in saddle points, but you have to have appropriate step sizes.
For more intuitions I suggest you referring here and here.
$endgroup$
1
$begingroup$
Exactly. These problems always pop up in theory, but rarely in actual practice. With so many dimensions, this isn't an issue. You'll have a local minima in one variable, but not in another. Furthermore, mini-batch or stochastic gradient descent ensures also help avoiding any local minima.
$endgroup$
– Ricardo Cruz
Nov 16 '17 at 17:38
2
$begingroup$
@RicardoCruz yes, I do agree sir
$endgroup$
– Vaalizaadeh
Nov 16 '17 at 20:30
add a comment |
$begingroup$
Gradient Descent is an algorithm which is designed to find the optimal points, but these optimal points are not necessarily global. And yes if it happens that it diverges from a local location it may converge to another optimal point but its probability is not too much. The reason is that the step size might be too large that prompts it recede one optimal point and the probability that it oscillates is much more than convergence.
About gradient descent there are two main perspectives, machine learning era and deep learning era. During machine learning era it was considered that gradient descent will find the local/global optimum but in deep learning era where the dimension of input features are too much it is shown in practice that the probability that all of the features be located in there optimal value at a single point is not too much and rather seeing to have optimal locations in cost functions, most of the time saddle points are observed. This is one of the reasons that training with lots of data and training epochs cause the deep learning models outperform other algorithms. So if you train your model, it will find a detour or will find its way to go downhill and do not stuck in saddle points, but you have to have appropriate step sizes.
For more intuitions I suggest you referring here and here.
$endgroup$
Gradient Descent is an algorithm which is designed to find the optimal points, but these optimal points are not necessarily global. And yes if it happens that it diverges from a local location it may converge to another optimal point but its probability is not too much. The reason is that the step size might be too large that prompts it recede one optimal point and the probability that it oscillates is much more than convergence.
About gradient descent there are two main perspectives, machine learning era and deep learning era. During machine learning era it was considered that gradient descent will find the local/global optimum but in deep learning era where the dimension of input features are too much it is shown in practice that the probability that all of the features be located in there optimal value at a single point is not too much and rather seeing to have optimal locations in cost functions, most of the time saddle points are observed. This is one of the reasons that training with lots of data and training epochs cause the deep learning models outperform other algorithms. So if you train your model, it will find a detour or will find its way to go downhill and do not stuck in saddle points, but you have to have appropriate step sizes.
For more intuitions I suggest you referring here and here.
edited Nov 9 '17 at 18:07
answered Nov 9 '17 at 17:56


VaalizaadehVaalizaadeh
7,65562265
7,65562265
1
$begingroup$
Exactly. These problems always pop up in theory, but rarely in actual practice. With so many dimensions, this isn't an issue. You'll have a local minima in one variable, but not in another. Furthermore, mini-batch or stochastic gradient descent ensures also help avoiding any local minima.
$endgroup$
– Ricardo Cruz
Nov 16 '17 at 17:38
2
$begingroup$
@RicardoCruz yes, I do agree sir
$endgroup$
– Vaalizaadeh
Nov 16 '17 at 20:30
add a comment |
1
$begingroup$
Exactly. These problems always pop up in theory, but rarely in actual practice. With so many dimensions, this isn't an issue. You'll have a local minima in one variable, but not in another. Furthermore, mini-batch or stochastic gradient descent ensures also help avoiding any local minima.
$endgroup$
– Ricardo Cruz
Nov 16 '17 at 17:38
2
$begingroup$
@RicardoCruz yes, I do agree sir
$endgroup$
– Vaalizaadeh
Nov 16 '17 at 20:30
1
1
$begingroup$
Exactly. These problems always pop up in theory, but rarely in actual practice. With so many dimensions, this isn't an issue. You'll have a local minima in one variable, but not in another. Furthermore, mini-batch or stochastic gradient descent ensures also help avoiding any local minima.
$endgroup$
– Ricardo Cruz
Nov 16 '17 at 17:38
$begingroup$
Exactly. These problems always pop up in theory, but rarely in actual practice. With so many dimensions, this isn't an issue. You'll have a local minima in one variable, but not in another. Furthermore, mini-batch or stochastic gradient descent ensures also help avoiding any local minima.
$endgroup$
– Ricardo Cruz
Nov 16 '17 at 17:38
2
2
$begingroup$
@RicardoCruz yes, I do agree sir
$endgroup$
– Vaalizaadeh
Nov 16 '17 at 20:30
$begingroup$
@RicardoCruz yes, I do agree sir
$endgroup$
– Vaalizaadeh
Nov 16 '17 at 20:30
add a comment |
$begingroup$
Asides from the points you mentioned (convergence to non-global minimums, and large step sizes possibly leading to non-convergent algorithms), "inflection ranges" might be a problem too.
Consider the following "recliner chair" type of function.
Obviously, this can be constructed so that there is a range in the middle where the gradient is the 0 vector. In this range, the algorithm can be stuck indefinitely. Inflection points are usually not considered local extrema.
$endgroup$
add a comment |
$begingroup$
Asides from the points you mentioned (convergence to non-global minimums, and large step sizes possibly leading to non-convergent algorithms), "inflection ranges" might be a problem too.
Consider the following "recliner chair" type of function.
Obviously, this can be constructed so that there is a range in the middle where the gradient is the 0 vector. In this range, the algorithm can be stuck indefinitely. Inflection points are usually not considered local extrema.
$endgroup$
add a comment |
$begingroup$
Asides from the points you mentioned (convergence to non-global minimums, and large step sizes possibly leading to non-convergent algorithms), "inflection ranges" might be a problem too.
Consider the following "recliner chair" type of function.
Obviously, this can be constructed so that there is a range in the middle where the gradient is the 0 vector. In this range, the algorithm can be stuck indefinitely. Inflection points are usually not considered local extrema.
$endgroup$
Asides from the points you mentioned (convergence to non-global minimums, and large step sizes possibly leading to non-convergent algorithms), "inflection ranges" might be a problem too.
Consider the following "recliner chair" type of function.
Obviously, this can be constructed so that there is a range in the middle where the gradient is the 0 vector. In this range, the algorithm can be stuck indefinitely. Inflection points are usually not considered local extrema.
answered Nov 9 '17 at 17:36


Ami TavoryAmi Tavory
58848
58848
add a comment |
add a comment |
$begingroup$
Conjugate gradient is not guaranteed to reach a global optimum or a local optimum! There are points where the gradient is very small, that are not optima (inflection points, saddle points). Gradient Descent could converge to a point $x = 0$ for the function $f(x) = x^3$.
$endgroup$
add a comment |
$begingroup$
Conjugate gradient is not guaranteed to reach a global optimum or a local optimum! There are points where the gradient is very small, that are not optima (inflection points, saddle points). Gradient Descent could converge to a point $x = 0$ for the function $f(x) = x^3$.
$endgroup$
add a comment |
$begingroup$
Conjugate gradient is not guaranteed to reach a global optimum or a local optimum! There are points where the gradient is very small, that are not optima (inflection points, saddle points). Gradient Descent could converge to a point $x = 0$ for the function $f(x) = x^3$.
$endgroup$
Conjugate gradient is not guaranteed to reach a global optimum or a local optimum! There are points where the gradient is very small, that are not optima (inflection points, saddle points). Gradient Descent could converge to a point $x = 0$ for the function $f(x) = x^3$.
edited Jun 1 '18 at 12:47


Stephen Rauch♦
1,52551330
1,52551330
answered Jun 1 '18 at 9:27
Herbert KnieriemHerbert Knieriem
211
211
add a comment |
add a comment |
$begingroup$
[Note 5 April 2019: A new version of the paper has been updated on arXiv with many new results. We introduce also backtracking versions of Momentum and NAG, and prove convergence under the same assumptions as for Backtracking Gradient Descent.
Source codes are available on GitHub at the link: https://github.com/hank-nguyen/MBT-optimizer
We improved the algorithms for applying to DNN, and obtain better performance than state-of-the-art algorithms such as MMT, NAG, Adam, Adamax, Adagrad,...
The most special feature of our algorithms are that they are automatic, you do not need to do manual fine-tuning of learning rates as common practice. Our automatic fine-tuning is different in nature from Adam, Adamax, Adagrad,... and so on. More details are in the paper.
]
Based on very recent results: In my joint work in this paper https://arxiv.org/abs/1808.05160
We showed that backtracking gradient descent, when applied to an arbitrary C^1 function $f$, with only a countable number of critical points, will always either converge to a critical point or diverge to infinity. This condition is satisfied for a generic function, for example for all Morse functions. We also showed that in a sense it is very rare for the limit point to be a saddle point. So if all of your critical points are non-degenerate, then in a certain sense the limit points are all minimums. [Please see also references in the cited paper for the known results in the case of the standard gradient descent.]
Based on the above, we proposed a new method in deep learning which is on par with current state-of-the-art methods and does not need manual fine-tuning of the learning rates. (In a nutshell, the idea is that you run backtracking gradient descent a certain amount of time, until you see that the learning rates, which change with each iteration, become stabilise. We expect this stabilisation, in particular at a critical point which is C^2 and is non-degenerate, because of the convergence result I mentioned above. At that point, you switch to the standard gradient descent method. Please see the cited paper for more detail. This method can also be applied to other optimal algorithms.)
P.S. Regarding your original question about the standard gradient descent method, to my knowledge only in the case where the derivative of the map is globally Lipschitz and the learning rate is small enough that the standard gradient descent method is proven to converge. [If these conditions are not satisfied, there are simple counter-examples showing that no convergence result is possible, see the cited paper for some.] In the paper cited above, we argued that in the long run the backtracking gradient descent method will become the standard gradient descent method, which gives an explanation why the standard gradient descent method usually works well in practice.
$endgroup$
add a comment |
$begingroup$
[Note 5 April 2019: A new version of the paper has been updated on arXiv with many new results. We introduce also backtracking versions of Momentum and NAG, and prove convergence under the same assumptions as for Backtracking Gradient Descent.
Source codes are available on GitHub at the link: https://github.com/hank-nguyen/MBT-optimizer
We improved the algorithms for applying to DNN, and obtain better performance than state-of-the-art algorithms such as MMT, NAG, Adam, Adamax, Adagrad,...
The most special feature of our algorithms are that they are automatic, you do not need to do manual fine-tuning of learning rates as common practice. Our automatic fine-tuning is different in nature from Adam, Adamax, Adagrad,... and so on. More details are in the paper.
]
Based on very recent results: In my joint work in this paper https://arxiv.org/abs/1808.05160
We showed that backtracking gradient descent, when applied to an arbitrary C^1 function $f$, with only a countable number of critical points, will always either converge to a critical point or diverge to infinity. This condition is satisfied for a generic function, for example for all Morse functions. We also showed that in a sense it is very rare for the limit point to be a saddle point. So if all of your critical points are non-degenerate, then in a certain sense the limit points are all minimums. [Please see also references in the cited paper for the known results in the case of the standard gradient descent.]
Based on the above, we proposed a new method in deep learning which is on par with current state-of-the-art methods and does not need manual fine-tuning of the learning rates. (In a nutshell, the idea is that you run backtracking gradient descent a certain amount of time, until you see that the learning rates, which change with each iteration, become stabilise. We expect this stabilisation, in particular at a critical point which is C^2 and is non-degenerate, because of the convergence result I mentioned above. At that point, you switch to the standard gradient descent method. Please see the cited paper for more detail. This method can also be applied to other optimal algorithms.)
P.S. Regarding your original question about the standard gradient descent method, to my knowledge only in the case where the derivative of the map is globally Lipschitz and the learning rate is small enough that the standard gradient descent method is proven to converge. [If these conditions are not satisfied, there are simple counter-examples showing that no convergence result is possible, see the cited paper for some.] In the paper cited above, we argued that in the long run the backtracking gradient descent method will become the standard gradient descent method, which gives an explanation why the standard gradient descent method usually works well in practice.
$endgroup$
add a comment |
$begingroup$
[Note 5 April 2019: A new version of the paper has been updated on arXiv with many new results. We introduce also backtracking versions of Momentum and NAG, and prove convergence under the same assumptions as for Backtracking Gradient Descent.
Source codes are available on GitHub at the link: https://github.com/hank-nguyen/MBT-optimizer
We improved the algorithms for applying to DNN, and obtain better performance than state-of-the-art algorithms such as MMT, NAG, Adam, Adamax, Adagrad,...
The most special feature of our algorithms are that they are automatic, you do not need to do manual fine-tuning of learning rates as common practice. Our automatic fine-tuning is different in nature from Adam, Adamax, Adagrad,... and so on. More details are in the paper.
]
Based on very recent results: In my joint work in this paper https://arxiv.org/abs/1808.05160
We showed that backtracking gradient descent, when applied to an arbitrary C^1 function $f$, with only a countable number of critical points, will always either converge to a critical point or diverge to infinity. This condition is satisfied for a generic function, for example for all Morse functions. We also showed that in a sense it is very rare for the limit point to be a saddle point. So if all of your critical points are non-degenerate, then in a certain sense the limit points are all minimums. [Please see also references in the cited paper for the known results in the case of the standard gradient descent.]
Based on the above, we proposed a new method in deep learning which is on par with current state-of-the-art methods and does not need manual fine-tuning of the learning rates. (In a nutshell, the idea is that you run backtracking gradient descent a certain amount of time, until you see that the learning rates, which change with each iteration, become stabilise. We expect this stabilisation, in particular at a critical point which is C^2 and is non-degenerate, because of the convergence result I mentioned above. At that point, you switch to the standard gradient descent method. Please see the cited paper for more detail. This method can also be applied to other optimal algorithms.)
P.S. Regarding your original question about the standard gradient descent method, to my knowledge only in the case where the derivative of the map is globally Lipschitz and the learning rate is small enough that the standard gradient descent method is proven to converge. [If these conditions are not satisfied, there are simple counter-examples showing that no convergence result is possible, see the cited paper for some.] In the paper cited above, we argued that in the long run the backtracking gradient descent method will become the standard gradient descent method, which gives an explanation why the standard gradient descent method usually works well in practice.
$endgroup$
[Note 5 April 2019: A new version of the paper has been updated on arXiv with many new results. We introduce also backtracking versions of Momentum and NAG, and prove convergence under the same assumptions as for Backtracking Gradient Descent.
Source codes are available on GitHub at the link: https://github.com/hank-nguyen/MBT-optimizer
We improved the algorithms for applying to DNN, and obtain better performance than state-of-the-art algorithms such as MMT, NAG, Adam, Adamax, Adagrad,...
The most special feature of our algorithms are that they are automatic, you do not need to do manual fine-tuning of learning rates as common practice. Our automatic fine-tuning is different in nature from Adam, Adamax, Adagrad,... and so on. More details are in the paper.
]
Based on very recent results: In my joint work in this paper https://arxiv.org/abs/1808.05160
We showed that backtracking gradient descent, when applied to an arbitrary C^1 function $f$, with only a countable number of critical points, will always either converge to a critical point or diverge to infinity. This condition is satisfied for a generic function, for example for all Morse functions. We also showed that in a sense it is very rare for the limit point to be a saddle point. So if all of your critical points are non-degenerate, then in a certain sense the limit points are all minimums. [Please see also references in the cited paper for the known results in the case of the standard gradient descent.]
Based on the above, we proposed a new method in deep learning which is on par with current state-of-the-art methods and does not need manual fine-tuning of the learning rates. (In a nutshell, the idea is that you run backtracking gradient descent a certain amount of time, until you see that the learning rates, which change with each iteration, become stabilise. We expect this stabilisation, in particular at a critical point which is C^2 and is non-degenerate, because of the convergence result I mentioned above. At that point, you switch to the standard gradient descent method. Please see the cited paper for more detail. This method can also be applied to other optimal algorithms.)
P.S. Regarding your original question about the standard gradient descent method, to my knowledge only in the case where the derivative of the map is globally Lipschitz and the learning rate is small enough that the standard gradient descent method is proven to converge. [If these conditions are not satisfied, there are simple counter-examples showing that no convergence result is possible, see the cited paper for some.] In the paper cited above, we argued that in the long run the backtracking gradient descent method will become the standard gradient descent method, which gives an explanation why the standard gradient descent method usually works well in practice.
edited Apr 8 at 3:45
answered Nov 3 '18 at 0:41
TuyenTuyen
313
313
add a comment |
add a comment |
Thanks for contributing an answer to Data Science Stack Exchange!
- Please be sure to answer the question. Provide details and share your research!
But avoid …
- Asking for help, clarification, or responding to other answers.
- Making statements based on opinion; back them up with references or personal experience.
Use MathJax to format equations. MathJax reference.
To learn more, see our tips on writing great answers.
Sign up or log in
StackExchange.ready(function ()
StackExchange.helpers.onClickDraftSave('#login-link');
);
Sign up using Google
Sign up using Facebook
Sign up using Email and Password
Post as a guest
Required, but never shown
StackExchange.ready(
function ()
StackExchange.openid.initPostLogin('.new-post-login', 'https%3a%2f%2fdatascience.stackexchange.com%2fquestions%2f24534%2fdoes-gradient-descent-always-converge-to-an-optimum%23new-answer', 'question_page');
);
Post as a guest
Required, but never shown
Sign up or log in
StackExchange.ready(function ()
StackExchange.helpers.onClickDraftSave('#login-link');
);
Sign up using Google
Sign up using Facebook
Sign up using Email and Password
Post as a guest
Required, but never shown
Sign up or log in
StackExchange.ready(function ()
StackExchange.helpers.onClickDraftSave('#login-link');
);
Sign up using Google
Sign up using Facebook
Sign up using Email and Password
Post as a guest
Required, but never shown
Sign up or log in
StackExchange.ready(function ()
StackExchange.helpers.onClickDraftSave('#login-link');
);
Sign up using Google
Sign up using Facebook
Sign up using Email and Password
Sign up using Google
Sign up using Facebook
Sign up using Email and Password
Post as a guest
Required, but never shown
Required, but never shown
Required, but never shown
Required, but never shown
Required, but never shown
Required, but never shown
Required, but never shown
Required, but never shown
Required, but never shown
sFIjUgW o20ynExKI5aSc65NdfWDx
$begingroup$
Hope this link will help in future..datascience.stackexchange.com/a/28417/35644
$endgroup$
– Aditya
Mar 3 '18 at 17:31
$begingroup$
See this answer for 3 concrete and simple examples, including proofs, images and code that creates an animation of the gradient descent
$endgroup$
– Oren Milman
Aug 27 '18 at 7:38