K.gradients gives type error where both arguments are tensors Announcing the arrival of Valued Associate #679: Cesar Manara Planned maintenance scheduled April 23, 2019 at 23:30 UTC (7:30pm US/Eastern) 2019 Moderator Election Q&A - Questionnaire 2019 Community Moderator Election ResultsWhich type auto encoder gives best results for textArchitecture for multivariate multi-time-series model where some features are TS specific and some features are globalHow to deal with classification where all target classes are independent (keras & image recognition)
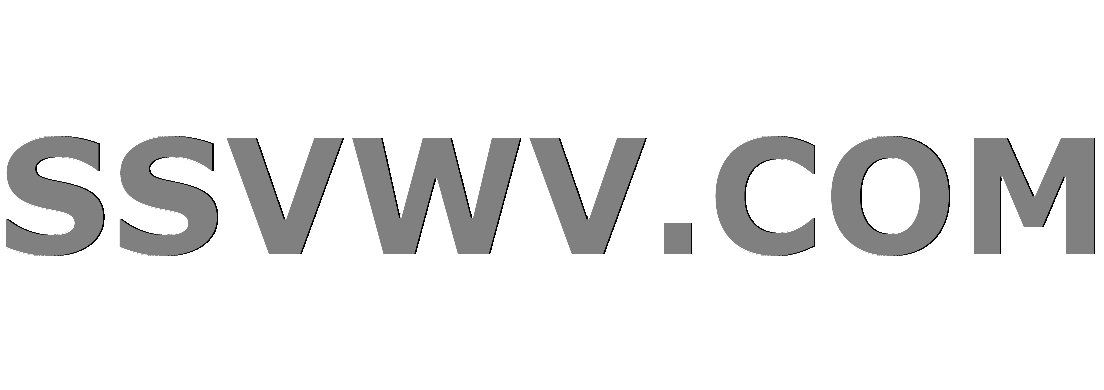
Multi tool use
How do you write "wild blueberries flavored"?
In musical terms, what properties are varied by the human voice to produce different words / syllables?
Did any compiler fully use 80-bit floating point?
How does the body cool itself in a stillsuit?
Noise in Eigenvalues plot
Was the pager message from Nick Fury to Captain Marvel unnecessary?
Inverse square law not accurate for non-point masses?
New Order #6: Easter Egg
What is "Lambda" in Heston's original paper on stochastic volatility models?
By what mechanism was the 2017 UK General Election called?
Where did Ptolemy compare the Earth to the distance of fixed stars?
How to make an animal which can only breed for a certain number of generations?
Why can't fire hurt Daenerys but it did to Jon Snow in season 1?
What did Turing mean when saying that "machines cannot give rise to surprises" is due to a fallacy?
Does the universe have a fixed centre of mass?
An isoperimetric-type inequality inside a cube
Why did Bronn offer to be Tyrion Lannister's champion in trial by combat?
Understanding piped commands in GNU/Linux
Did John Wesley plagiarize Matthew Henry...?
Flight departed from the gate 5 min before scheduled departure time. Refund options
Vertical ranges of Column Plots in 12
Why is there so little support for joining EFTA in the British parliament?
Calculation of line of sight system gain
The test team as an enemy of development? And how can this be avoided?
K.gradients gives type error where both arguments are tensors
Announcing the arrival of Valued Associate #679: Cesar Manara
Planned maintenance scheduled April 23, 2019 at 23:30 UTC (7:30pm US/Eastern)
2019 Moderator Election Q&A - Questionnaire
2019 Community Moderator Election ResultsWhich type auto encoder gives best results for textArchitecture for multivariate multi-time-series model where some features are TS specific and some features are globalHow to deal with classification where all target classes are independent (keras & image recognition)
$begingroup$
On lines 142 and 143 of: https://github.com/nyck33/openai_spinup_my_implements/blob/master/continuous/mountaincar/my_ddpg_ac.py
I have:
self.get_action_gradients = K.function(inputs=[self.model.input[0], self.model.input[1],
K.learning_phase()], outputs=[action_gradients])
Which tells me:line 143, in build_model
K.learning_phase()], outputs=[action_gradients])
TypeError: Can not convert a list into a Tensor or Operation.
action_gradients
are calculated on line 140 via:
action_gradients = K.gradients(Q_value, actions)
so I did not think that is a problem but when I take the brackets off of the output argument for K.function
like so:
self.get_action_gradients = K.function(inputs=[*self.model.input,
K.learning_phase()], outputs=action_gradients)
Now I get a slightly different error mentioning Nonetype
rather than list
:
rning-copied/_1my_imps/continuous/mountaincar/my_ddpg_ac.py", line 143, in build_model
TypeError: Can not convert a NoneType into a Tensor or Operation.
Printing out the Q_values and actions show they are tensors:
Q_values Tensor("q_values/BiasAdd:0", shape=(?, 1), dtype=float32) actions Tensor("actions:0", shape=(?, 1), dtype=float32)
But printing out the action_gradients
and type(action_gradients)
just confuses me more:
action_gradients [None]
action_gradients type <class 'list'>
calling: K.gradients()
on two tensors should work shouldn't it?
This DDPG code is originally from here: https://github.com/nyck33/autonomous_quadcopter
and I am trying to adapt it for MountainCarContinuous-v0
keras
$endgroup$
add a comment |
$begingroup$
On lines 142 and 143 of: https://github.com/nyck33/openai_spinup_my_implements/blob/master/continuous/mountaincar/my_ddpg_ac.py
I have:
self.get_action_gradients = K.function(inputs=[self.model.input[0], self.model.input[1],
K.learning_phase()], outputs=[action_gradients])
Which tells me:line 143, in build_model
K.learning_phase()], outputs=[action_gradients])
TypeError: Can not convert a list into a Tensor or Operation.
action_gradients
are calculated on line 140 via:
action_gradients = K.gradients(Q_value, actions)
so I did not think that is a problem but when I take the brackets off of the output argument for K.function
like so:
self.get_action_gradients = K.function(inputs=[*self.model.input,
K.learning_phase()], outputs=action_gradients)
Now I get a slightly different error mentioning Nonetype
rather than list
:
rning-copied/_1my_imps/continuous/mountaincar/my_ddpg_ac.py", line 143, in build_model
TypeError: Can not convert a NoneType into a Tensor or Operation.
Printing out the Q_values and actions show they are tensors:
Q_values Tensor("q_values/BiasAdd:0", shape=(?, 1), dtype=float32) actions Tensor("actions:0", shape=(?, 1), dtype=float32)
But printing out the action_gradients
and type(action_gradients)
just confuses me more:
action_gradients [None]
action_gradients type <class 'list'>
calling: K.gradients()
on two tensors should work shouldn't it?
This DDPG code is originally from here: https://github.com/nyck33/autonomous_quadcopter
and I am trying to adapt it for MountainCarContinuous-v0
keras
$endgroup$
add a comment |
$begingroup$
On lines 142 and 143 of: https://github.com/nyck33/openai_spinup_my_implements/blob/master/continuous/mountaincar/my_ddpg_ac.py
I have:
self.get_action_gradients = K.function(inputs=[self.model.input[0], self.model.input[1],
K.learning_phase()], outputs=[action_gradients])
Which tells me:line 143, in build_model
K.learning_phase()], outputs=[action_gradients])
TypeError: Can not convert a list into a Tensor or Operation.
action_gradients
are calculated on line 140 via:
action_gradients = K.gradients(Q_value, actions)
so I did not think that is a problem but when I take the brackets off of the output argument for K.function
like so:
self.get_action_gradients = K.function(inputs=[*self.model.input,
K.learning_phase()], outputs=action_gradients)
Now I get a slightly different error mentioning Nonetype
rather than list
:
rning-copied/_1my_imps/continuous/mountaincar/my_ddpg_ac.py", line 143, in build_model
TypeError: Can not convert a NoneType into a Tensor or Operation.
Printing out the Q_values and actions show they are tensors:
Q_values Tensor("q_values/BiasAdd:0", shape=(?, 1), dtype=float32) actions Tensor("actions:0", shape=(?, 1), dtype=float32)
But printing out the action_gradients
and type(action_gradients)
just confuses me more:
action_gradients [None]
action_gradients type <class 'list'>
calling: K.gradients()
on two tensors should work shouldn't it?
This DDPG code is originally from here: https://github.com/nyck33/autonomous_quadcopter
and I am trying to adapt it for MountainCarContinuous-v0
keras
$endgroup$
On lines 142 and 143 of: https://github.com/nyck33/openai_spinup_my_implements/blob/master/continuous/mountaincar/my_ddpg_ac.py
I have:
self.get_action_gradients = K.function(inputs=[self.model.input[0], self.model.input[1],
K.learning_phase()], outputs=[action_gradients])
Which tells me:line 143, in build_model
K.learning_phase()], outputs=[action_gradients])
TypeError: Can not convert a list into a Tensor or Operation.
action_gradients
are calculated on line 140 via:
action_gradients = K.gradients(Q_value, actions)
so I did not think that is a problem but when I take the brackets off of the output argument for K.function
like so:
self.get_action_gradients = K.function(inputs=[*self.model.input,
K.learning_phase()], outputs=action_gradients)
Now I get a slightly different error mentioning Nonetype
rather than list
:
rning-copied/_1my_imps/continuous/mountaincar/my_ddpg_ac.py", line 143, in build_model
TypeError: Can not convert a NoneType into a Tensor or Operation.
Printing out the Q_values and actions show they are tensors:
Q_values Tensor("q_values/BiasAdd:0", shape=(?, 1), dtype=float32) actions Tensor("actions:0", shape=(?, 1), dtype=float32)
But printing out the action_gradients
and type(action_gradients)
just confuses me more:
action_gradients [None]
action_gradients type <class 'list'>
calling: K.gradients()
on two tensors should work shouldn't it?
This DDPG code is originally from here: https://github.com/nyck33/autonomous_quadcopter
and I am trying to adapt it for MountainCarContinuous-v0
keras
keras
edited Apr 4 at 12:39
mLstudent33
asked Mar 29 at 17:30
mLstudent33mLstudent33
507
507
add a comment |
add a comment |
1 Answer
1
active
oldest
votes
$begingroup$
I am very sorry but this was a careless error. In the critic network I had different pathways for state
and action
, joining them right before the Q-value output layer but...
I actually had state
as the input for both pathways rather than action
for the action pathway.
So of course, if the action
is not present in a network, there is no way to use the chain rule to get the dQ(s,a)/da gradient of output w.r.t. actions.
Here is the code for clarification:
def build_model(self):
#lrelu = LeakyReLU(alpha=0.1)
#Define input layers
states = layers.Input(shape=(self.state_size,), name="states")
actions = layers.Input(shape=(self.action_size,), name="actions")
#Add hidden layers for state pathway
net_states = layers.Dense(units=32, use_bias=False)(states)
net_states = layers.BatchNormalization()(net_states)
net_states = layers.LeakyReLU(alpha=0.1)(net_states)
net_states = layers.Dense(units=64)(net_states)
net_states = layers.BatchNormalization()(net_states)
net_states = layers.LeakyReLU(alpha=0.1)(net_states)
#hidden layers for action
net_actions = layers.Dense(units=32)(actions) #had (states) here instead
net_actions = layers.BatchNormalization()(net_actions)
net_actions = layers.LeakyReLU(alpha=0.1)(net_actions)
net_actions = layers.Dense(units=64)(net_actions)
net_actions = layers.BatchNormalization()(net_actions)
net_actions = layers.LeakyReLU(alpha=0.1)(net_actions)
and the output to console shows that it is no longer a None
type:
action_gradients [<tf.Tensor 'gradients_3/dense_13/MatMul_grad/MatMul:0' shape=(?, 1) dtype=float32>]
Edit: The code works for MtnCarContinuous-v0 environment of openai gym with a modified reward function (incremental based on car position because the final reward is so sparse), over 90 seems to be achieved in 11 episodes. I rendered so actually saw the car make it to the top on the attempts where score is over 100 below.
Here are the results:
episode: 0 score: -41.59034754863541 mean: -41.59 std: 0.0
episode: 1 score: -75.87242108451743 mean: -58.73 std: 17.14
episode: 2 score: 32.01844640263133 mean: -28.48 std: 45.01
episode: 3 score: 132.904319261567 mean: 11.86 std: 80.02
episode: 4 score: 125.81198290946529 mean: 34.65 std: 84.85
episode: 5 score: 84.26163480413017 mean: 42.92 std: 79.63
episode: 6 score: 126.89684490110164 mean: 54.92 std: 79.37
episode: 7 score: 139.18190524840517 mean: 65.45 std: 79.3
episode: 8 score: 100.24481691450521 mean: 69.32 std: 75.56
episode: 9 score: 165.80286734425076 mean: 78.97 std: 77.31
episode: 10 score: 109.29507292352991 mean: 94.05 std: 66.24
episode: 11 score: 209.07900825070152 mean: 122.55 std: 44.84
Source code here: https://github.com/nyck33/openai_my_implements/tree/master/continuous/mountaincar
$endgroup$
add a comment |
Your Answer
StackExchange.ready(function()
var channelOptions =
tags: "".split(" "),
id: "557"
;
initTagRenderer("".split(" "), "".split(" "), channelOptions);
StackExchange.using("externalEditor", function()
// Have to fire editor after snippets, if snippets enabled
if (StackExchange.settings.snippets.snippetsEnabled)
StackExchange.using("snippets", function()
createEditor();
);
else
createEditor();
);
function createEditor()
StackExchange.prepareEditor(
heartbeatType: 'answer',
autoActivateHeartbeat: false,
convertImagesToLinks: false,
noModals: true,
showLowRepImageUploadWarning: true,
reputationToPostImages: null,
bindNavPrevention: true,
postfix: "",
imageUploader:
brandingHtml: "Powered by u003ca class="icon-imgur-white" href="https://imgur.com/"u003eu003c/au003e",
contentPolicyHtml: "User contributions licensed under u003ca href="https://creativecommons.org/licenses/by-sa/3.0/"u003ecc by-sa 3.0 with attribution requiredu003c/au003e u003ca href="https://stackoverflow.com/legal/content-policy"u003e(content policy)u003c/au003e",
allowUrls: true
,
onDemand: true,
discardSelector: ".discard-answer"
,immediatelyShowMarkdownHelp:true
);
);
Sign up or log in
StackExchange.ready(function ()
StackExchange.helpers.onClickDraftSave('#login-link');
);
Sign up using Google
Sign up using Facebook
Sign up using Email and Password
Post as a guest
Required, but never shown
StackExchange.ready(
function ()
StackExchange.openid.initPostLogin('.new-post-login', 'https%3a%2f%2fdatascience.stackexchange.com%2fquestions%2f48228%2fk-gradients-gives-type-error-where-both-arguments-are-tensors%23new-answer', 'question_page');
);
Post as a guest
Required, but never shown
1 Answer
1
active
oldest
votes
1 Answer
1
active
oldest
votes
active
oldest
votes
active
oldest
votes
$begingroup$
I am very sorry but this was a careless error. In the critic network I had different pathways for state
and action
, joining them right before the Q-value output layer but...
I actually had state
as the input for both pathways rather than action
for the action pathway.
So of course, if the action
is not present in a network, there is no way to use the chain rule to get the dQ(s,a)/da gradient of output w.r.t. actions.
Here is the code for clarification:
def build_model(self):
#lrelu = LeakyReLU(alpha=0.1)
#Define input layers
states = layers.Input(shape=(self.state_size,), name="states")
actions = layers.Input(shape=(self.action_size,), name="actions")
#Add hidden layers for state pathway
net_states = layers.Dense(units=32, use_bias=False)(states)
net_states = layers.BatchNormalization()(net_states)
net_states = layers.LeakyReLU(alpha=0.1)(net_states)
net_states = layers.Dense(units=64)(net_states)
net_states = layers.BatchNormalization()(net_states)
net_states = layers.LeakyReLU(alpha=0.1)(net_states)
#hidden layers for action
net_actions = layers.Dense(units=32)(actions) #had (states) here instead
net_actions = layers.BatchNormalization()(net_actions)
net_actions = layers.LeakyReLU(alpha=0.1)(net_actions)
net_actions = layers.Dense(units=64)(net_actions)
net_actions = layers.BatchNormalization()(net_actions)
net_actions = layers.LeakyReLU(alpha=0.1)(net_actions)
and the output to console shows that it is no longer a None
type:
action_gradients [<tf.Tensor 'gradients_3/dense_13/MatMul_grad/MatMul:0' shape=(?, 1) dtype=float32>]
Edit: The code works for MtnCarContinuous-v0 environment of openai gym with a modified reward function (incremental based on car position because the final reward is so sparse), over 90 seems to be achieved in 11 episodes. I rendered so actually saw the car make it to the top on the attempts where score is over 100 below.
Here are the results:
episode: 0 score: -41.59034754863541 mean: -41.59 std: 0.0
episode: 1 score: -75.87242108451743 mean: -58.73 std: 17.14
episode: 2 score: 32.01844640263133 mean: -28.48 std: 45.01
episode: 3 score: 132.904319261567 mean: 11.86 std: 80.02
episode: 4 score: 125.81198290946529 mean: 34.65 std: 84.85
episode: 5 score: 84.26163480413017 mean: 42.92 std: 79.63
episode: 6 score: 126.89684490110164 mean: 54.92 std: 79.37
episode: 7 score: 139.18190524840517 mean: 65.45 std: 79.3
episode: 8 score: 100.24481691450521 mean: 69.32 std: 75.56
episode: 9 score: 165.80286734425076 mean: 78.97 std: 77.31
episode: 10 score: 109.29507292352991 mean: 94.05 std: 66.24
episode: 11 score: 209.07900825070152 mean: 122.55 std: 44.84
Source code here: https://github.com/nyck33/openai_my_implements/tree/master/continuous/mountaincar
$endgroup$
add a comment |
$begingroup$
I am very sorry but this was a careless error. In the critic network I had different pathways for state
and action
, joining them right before the Q-value output layer but...
I actually had state
as the input for both pathways rather than action
for the action pathway.
So of course, if the action
is not present in a network, there is no way to use the chain rule to get the dQ(s,a)/da gradient of output w.r.t. actions.
Here is the code for clarification:
def build_model(self):
#lrelu = LeakyReLU(alpha=0.1)
#Define input layers
states = layers.Input(shape=(self.state_size,), name="states")
actions = layers.Input(shape=(self.action_size,), name="actions")
#Add hidden layers for state pathway
net_states = layers.Dense(units=32, use_bias=False)(states)
net_states = layers.BatchNormalization()(net_states)
net_states = layers.LeakyReLU(alpha=0.1)(net_states)
net_states = layers.Dense(units=64)(net_states)
net_states = layers.BatchNormalization()(net_states)
net_states = layers.LeakyReLU(alpha=0.1)(net_states)
#hidden layers for action
net_actions = layers.Dense(units=32)(actions) #had (states) here instead
net_actions = layers.BatchNormalization()(net_actions)
net_actions = layers.LeakyReLU(alpha=0.1)(net_actions)
net_actions = layers.Dense(units=64)(net_actions)
net_actions = layers.BatchNormalization()(net_actions)
net_actions = layers.LeakyReLU(alpha=0.1)(net_actions)
and the output to console shows that it is no longer a None
type:
action_gradients [<tf.Tensor 'gradients_3/dense_13/MatMul_grad/MatMul:0' shape=(?, 1) dtype=float32>]
Edit: The code works for MtnCarContinuous-v0 environment of openai gym with a modified reward function (incremental based on car position because the final reward is so sparse), over 90 seems to be achieved in 11 episodes. I rendered so actually saw the car make it to the top on the attempts where score is over 100 below.
Here are the results:
episode: 0 score: -41.59034754863541 mean: -41.59 std: 0.0
episode: 1 score: -75.87242108451743 mean: -58.73 std: 17.14
episode: 2 score: 32.01844640263133 mean: -28.48 std: 45.01
episode: 3 score: 132.904319261567 mean: 11.86 std: 80.02
episode: 4 score: 125.81198290946529 mean: 34.65 std: 84.85
episode: 5 score: 84.26163480413017 mean: 42.92 std: 79.63
episode: 6 score: 126.89684490110164 mean: 54.92 std: 79.37
episode: 7 score: 139.18190524840517 mean: 65.45 std: 79.3
episode: 8 score: 100.24481691450521 mean: 69.32 std: 75.56
episode: 9 score: 165.80286734425076 mean: 78.97 std: 77.31
episode: 10 score: 109.29507292352991 mean: 94.05 std: 66.24
episode: 11 score: 209.07900825070152 mean: 122.55 std: 44.84
Source code here: https://github.com/nyck33/openai_my_implements/tree/master/continuous/mountaincar
$endgroup$
add a comment |
$begingroup$
I am very sorry but this was a careless error. In the critic network I had different pathways for state
and action
, joining them right before the Q-value output layer but...
I actually had state
as the input for both pathways rather than action
for the action pathway.
So of course, if the action
is not present in a network, there is no way to use the chain rule to get the dQ(s,a)/da gradient of output w.r.t. actions.
Here is the code for clarification:
def build_model(self):
#lrelu = LeakyReLU(alpha=0.1)
#Define input layers
states = layers.Input(shape=(self.state_size,), name="states")
actions = layers.Input(shape=(self.action_size,), name="actions")
#Add hidden layers for state pathway
net_states = layers.Dense(units=32, use_bias=False)(states)
net_states = layers.BatchNormalization()(net_states)
net_states = layers.LeakyReLU(alpha=0.1)(net_states)
net_states = layers.Dense(units=64)(net_states)
net_states = layers.BatchNormalization()(net_states)
net_states = layers.LeakyReLU(alpha=0.1)(net_states)
#hidden layers for action
net_actions = layers.Dense(units=32)(actions) #had (states) here instead
net_actions = layers.BatchNormalization()(net_actions)
net_actions = layers.LeakyReLU(alpha=0.1)(net_actions)
net_actions = layers.Dense(units=64)(net_actions)
net_actions = layers.BatchNormalization()(net_actions)
net_actions = layers.LeakyReLU(alpha=0.1)(net_actions)
and the output to console shows that it is no longer a None
type:
action_gradients [<tf.Tensor 'gradients_3/dense_13/MatMul_grad/MatMul:0' shape=(?, 1) dtype=float32>]
Edit: The code works for MtnCarContinuous-v0 environment of openai gym with a modified reward function (incremental based on car position because the final reward is so sparse), over 90 seems to be achieved in 11 episodes. I rendered so actually saw the car make it to the top on the attempts where score is over 100 below.
Here are the results:
episode: 0 score: -41.59034754863541 mean: -41.59 std: 0.0
episode: 1 score: -75.87242108451743 mean: -58.73 std: 17.14
episode: 2 score: 32.01844640263133 mean: -28.48 std: 45.01
episode: 3 score: 132.904319261567 mean: 11.86 std: 80.02
episode: 4 score: 125.81198290946529 mean: 34.65 std: 84.85
episode: 5 score: 84.26163480413017 mean: 42.92 std: 79.63
episode: 6 score: 126.89684490110164 mean: 54.92 std: 79.37
episode: 7 score: 139.18190524840517 mean: 65.45 std: 79.3
episode: 8 score: 100.24481691450521 mean: 69.32 std: 75.56
episode: 9 score: 165.80286734425076 mean: 78.97 std: 77.31
episode: 10 score: 109.29507292352991 mean: 94.05 std: 66.24
episode: 11 score: 209.07900825070152 mean: 122.55 std: 44.84
Source code here: https://github.com/nyck33/openai_my_implements/tree/master/continuous/mountaincar
$endgroup$
I am very sorry but this was a careless error. In the critic network I had different pathways for state
and action
, joining them right before the Q-value output layer but...
I actually had state
as the input for both pathways rather than action
for the action pathway.
So of course, if the action
is not present in a network, there is no way to use the chain rule to get the dQ(s,a)/da gradient of output w.r.t. actions.
Here is the code for clarification:
def build_model(self):
#lrelu = LeakyReLU(alpha=0.1)
#Define input layers
states = layers.Input(shape=(self.state_size,), name="states")
actions = layers.Input(shape=(self.action_size,), name="actions")
#Add hidden layers for state pathway
net_states = layers.Dense(units=32, use_bias=False)(states)
net_states = layers.BatchNormalization()(net_states)
net_states = layers.LeakyReLU(alpha=0.1)(net_states)
net_states = layers.Dense(units=64)(net_states)
net_states = layers.BatchNormalization()(net_states)
net_states = layers.LeakyReLU(alpha=0.1)(net_states)
#hidden layers for action
net_actions = layers.Dense(units=32)(actions) #had (states) here instead
net_actions = layers.BatchNormalization()(net_actions)
net_actions = layers.LeakyReLU(alpha=0.1)(net_actions)
net_actions = layers.Dense(units=64)(net_actions)
net_actions = layers.BatchNormalization()(net_actions)
net_actions = layers.LeakyReLU(alpha=0.1)(net_actions)
and the output to console shows that it is no longer a None
type:
action_gradients [<tf.Tensor 'gradients_3/dense_13/MatMul_grad/MatMul:0' shape=(?, 1) dtype=float32>]
Edit: The code works for MtnCarContinuous-v0 environment of openai gym with a modified reward function (incremental based on car position because the final reward is so sparse), over 90 seems to be achieved in 11 episodes. I rendered so actually saw the car make it to the top on the attempts where score is over 100 below.
Here are the results:
episode: 0 score: -41.59034754863541 mean: -41.59 std: 0.0
episode: 1 score: -75.87242108451743 mean: -58.73 std: 17.14
episode: 2 score: 32.01844640263133 mean: -28.48 std: 45.01
episode: 3 score: 132.904319261567 mean: 11.86 std: 80.02
episode: 4 score: 125.81198290946529 mean: 34.65 std: 84.85
episode: 5 score: 84.26163480413017 mean: 42.92 std: 79.63
episode: 6 score: 126.89684490110164 mean: 54.92 std: 79.37
episode: 7 score: 139.18190524840517 mean: 65.45 std: 79.3
episode: 8 score: 100.24481691450521 mean: 69.32 std: 75.56
episode: 9 score: 165.80286734425076 mean: 78.97 std: 77.31
episode: 10 score: 109.29507292352991 mean: 94.05 std: 66.24
episode: 11 score: 209.07900825070152 mean: 122.55 std: 44.84
Source code here: https://github.com/nyck33/openai_my_implements/tree/master/continuous/mountaincar
edited Apr 5 at 6:56
answered Apr 5 at 5:37
mLstudent33mLstudent33
507
507
add a comment |
add a comment |
Thanks for contributing an answer to Data Science Stack Exchange!
- Please be sure to answer the question. Provide details and share your research!
But avoid …
- Asking for help, clarification, or responding to other answers.
- Making statements based on opinion; back them up with references or personal experience.
Use MathJax to format equations. MathJax reference.
To learn more, see our tips on writing great answers.
Sign up or log in
StackExchange.ready(function ()
StackExchange.helpers.onClickDraftSave('#login-link');
);
Sign up using Google
Sign up using Facebook
Sign up using Email and Password
Post as a guest
Required, but never shown
StackExchange.ready(
function ()
StackExchange.openid.initPostLogin('.new-post-login', 'https%3a%2f%2fdatascience.stackexchange.com%2fquestions%2f48228%2fk-gradients-gives-type-error-where-both-arguments-are-tensors%23new-answer', 'question_page');
);
Post as a guest
Required, but never shown
Sign up or log in
StackExchange.ready(function ()
StackExchange.helpers.onClickDraftSave('#login-link');
);
Sign up using Google
Sign up using Facebook
Sign up using Email and Password
Post as a guest
Required, but never shown
Sign up or log in
StackExchange.ready(function ()
StackExchange.helpers.onClickDraftSave('#login-link');
);
Sign up using Google
Sign up using Facebook
Sign up using Email and Password
Post as a guest
Required, but never shown
Sign up or log in
StackExchange.ready(function ()
StackExchange.helpers.onClickDraftSave('#login-link');
);
Sign up using Google
Sign up using Facebook
Sign up using Email and Password
Sign up using Google
Sign up using Facebook
Sign up using Email and Password
Post as a guest
Required, but never shown
Required, but never shown
Required, but never shown
Required, but never shown
Required, but never shown
Required, but never shown
Required, but never shown
Required, but never shown
Required, but never shown
2,gw u6tqp8kCpPj41mOr1llxeV9P4IUn4WB4