Why does CV yield lower score? Announcing the arrival of Valued Associate #679: Cesar Manara Planned maintenance scheduled April 23, 2019 at 23:30 UTC (7:30pm US/Eastern) 2019 Moderator Election Q&A - Questionnaire 2019 Community Moderator Election ResultsVariance in cross validation score / model selectionConsistently inconsistent cross-validation results that are wildly different from original model accuracyHow does k fold cross validation work?Why is cross-validation score so low?Titanic Kaggle Data: Why am I getting lower accuracy on Kaggle submissions than on held-out data?Validation accuracy is always close to training accuracyDoes a precision score increasing with a higher number of folds mean the model will improve with more data?Validition score while training lower than on final model with xgboostPCA, SMOTE and cross validation- how to combine them together?
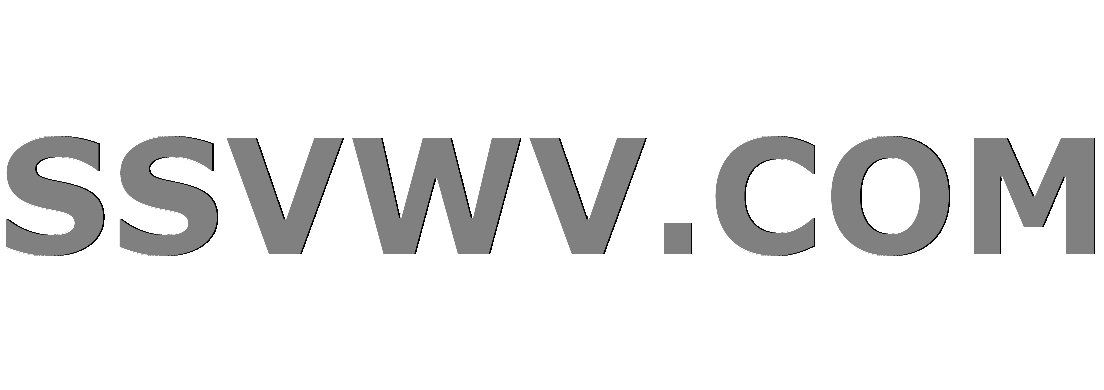
Multi tool use
How do you write "wild blueberries flavored"?
Did any compiler fully use 80-bit floating point?
How to name indistinguishable henchmen in a screenplay?
Adapting the Chinese Remainder Theorem (CRT) for integers to polynomials
Can gravitational waves pass through a black hole?
Table formatting with tabularx?
Found this skink in my tomato plant bucket. Is he trapped? Or could he leave if he wanted?
Vertical ranges of Column Plots in 12
What are some likely causes to domain member PC losing contact to domain controller?
3D Masyu - A Die
newbie Q : How to read an output file in one command line
Weaponising the Grasp-at-a-Distance spell
How do Java 8 default methods hеlp with lambdas?
How do I say "this must not happen"?
In musical terms, what properties are varied by the human voice to produce different words / syllables?
Did pre-Columbian Americans know the spherical shape of the Earth?
Plotting a Maclaurin series
Is there a spell that can create a permanent fire?
Flight departed from the gate 5 min before scheduled departure time. Refund options
Was the pager message from Nick Fury to Captain Marvel unnecessary?
Diophantine equation 3^a+1=3^b+5^c
Is it OK to use the testing sample to compare algorithms?
NIntegrate on a solution of a matrix ODE
Keep at all times, the minus sign above aligned with minus sign below
Why does CV yield lower score?
Announcing the arrival of Valued Associate #679: Cesar Manara
Planned maintenance scheduled April 23, 2019 at 23:30 UTC (7:30pm US/Eastern)
2019 Moderator Election Q&A - Questionnaire
2019 Community Moderator Election ResultsVariance in cross validation score / model selectionConsistently inconsistent cross-validation results that are wildly different from original model accuracyHow does k fold cross validation work?Why is cross-validation score so low?Titanic Kaggle Data: Why am I getting lower accuracy on Kaggle submissions than on held-out data?Validation accuracy is always close to training accuracyDoes a precision score increasing with a higher number of folds mean the model will improve with more data?Validition score while training lower than on final model with xgboostPCA, SMOTE and cross validation- how to combine them together?
$begingroup$
My training accuracy was better than my test accuracy, hence I thought my model was over-fitted and tried Cross-validation. The model further degraded. Is that my input data need to be sanitised further and of better quality?
Please share your thoughts what could be getting wrong here.
My data distribution:
Code snippets...
My function get_score
:
def get_score(model, X_train, X_test, y_train, y_test):
model.fit(X_train, y_train.values.ravel())
pred0 = model.predict(X_test)
return accuracy_score(y_test, pred0)
Logic:
print('*TRAIN* Accuracy Score => '+str(accuracy_score(y_train, m.predict(X_train)))) # LinearSVC() used
print('*TEST* Accuracy Score => '+str(accuracy_score(y_test, pred))) # LinearSVC() used
print("... Cross Validation begins...")
y0 = pd.DataFrame(y)
y0.reset_index(drop=True, inplace=True)
print(X.shape)
print(y0.shape)
kf = KFold(n_splits = 10)
e = []
for train_index, test_index in kf.split(X):
X_train, X_test, y_train, y_test = X.iloc[train_index], X.iloc[test_index],y0.iloc[train_index], y0.iloc[test_index]
print(train_index, test_index)
e.append(get_score(LinearSVC(random_state=777),X_train, X_test, y_train, y_test))
print("Finally :: "+str(np.mean(e)))
Output:
*TRAIN* Accuracy Score => 0.9451327433628318
*TEST* Accuracy Score => 0.6597345132743363
... Cross Validation begins...
(9040, 6458)
(9040, 1)
[ 904 905 906 ... 9037 9038 9039] [ 0 1 2 ... 901 902 903]
[ 0 1 2 ... 9037 9038 9039] [ 904 905 906 ... 1805 1806 1807]
[ 0 1 2 ... 9037 9038 9039] [1808 1809 1810 ... 2709 2710 2711]
[ 0 1 2 ... 9037 9038 9039] [2712 2713 2714 ... 3613 3614 3615]
[ 0 1 2 ... 9037 9038 9039] [3616 3617 3618 ... 4517 4518 4519]
[ 0 1 2 ... 9037 9038 9039] [4520 4521 4522 ... 5421 5422 5423]
[ 0 1 2 ... 9037 9038 9039] [5424 5425 5426 ... 6325 6326 6327]
[ 0 1 2 ... 9037 9038 9039] [6328 6329 6330 ... 7229 7230 7231]
[ 0 1 2 ... 9037 9038 9039] [7232 7233 7234 ... 8133 8134 8135]
[ 0 1 2 ... 8133 8134 8135] [8136 8137 8138 ... 9037 9038 9039]
Finally :: 0.32499999999999996
>>>
Edit -1- Adding values of "e"
[0.08075221238938053, 0.413716814159292, 0.05752212389380531, 0.15376106194690264, 0.14712389380530974, 0.4668141592920354, 0.6946902654867256, 0.7112831858407079, 0.33738938053097345, 0.18694690265486727]
Edit -2- Adding shuffle=True
parameter to KFold()
Result:
[ 0 1 2 ... 9037 9038 9039] [ 4 5 10 ... 9007 9011 9024]
[ 0 1 2 ... 9037 9038 9039] [ 21 43 44 ... 9018 9035 9036]
[ 0 2 3 ... 9037 9038 9039] [ 1 20 60 ... 9023 9031 9034]
[ 0 1 2 ... 9036 9037 9038] [ 6 25 27 ... 9010 9025 9039]
[ 0 1 2 ... 9037 9038 9039] [ 15 16 28 ... 9029 9030 9033]
[ 0 1 2 ... 9037 9038 9039] [ 3 12 40 ... 9015 9017 9028]
[ 0 1 2 ... 9037 9038 9039] [ 7 8 23 ... 9013 9014 9027]
[ 0 1 3 ... 9035 9036 9039] [ 2 18 19 ... 9019 9037 9038]
[ 0 1 2 ... 9037 9038 9039] [ 24 37 39 ... 9012 9016 9026]
[ 1 2 3 ... 9037 9038 9039] [ 0 9 14 ... 9020 9021 9032]
[0.6504424778761062, 0.6736725663716814, 0.6969026548672567, 0.6692477876106194, 0.6769911504424779, 0.6382743362831859, 0.6692477876106194, 0.6648230088495575, 0.6648230088495575, 0.6814159292035398]
Finally :: 0.6685840707964601
cross-validation multiclass-classification prediction
$endgroup$
|
show 7 more comments
$begingroup$
My training accuracy was better than my test accuracy, hence I thought my model was over-fitted and tried Cross-validation. The model further degraded. Is that my input data need to be sanitised further and of better quality?
Please share your thoughts what could be getting wrong here.
My data distribution:
Code snippets...
My function get_score
:
def get_score(model, X_train, X_test, y_train, y_test):
model.fit(X_train, y_train.values.ravel())
pred0 = model.predict(X_test)
return accuracy_score(y_test, pred0)
Logic:
print('*TRAIN* Accuracy Score => '+str(accuracy_score(y_train, m.predict(X_train)))) # LinearSVC() used
print('*TEST* Accuracy Score => '+str(accuracy_score(y_test, pred))) # LinearSVC() used
print("... Cross Validation begins...")
y0 = pd.DataFrame(y)
y0.reset_index(drop=True, inplace=True)
print(X.shape)
print(y0.shape)
kf = KFold(n_splits = 10)
e = []
for train_index, test_index in kf.split(X):
X_train, X_test, y_train, y_test = X.iloc[train_index], X.iloc[test_index],y0.iloc[train_index], y0.iloc[test_index]
print(train_index, test_index)
e.append(get_score(LinearSVC(random_state=777),X_train, X_test, y_train, y_test))
print("Finally :: "+str(np.mean(e)))
Output:
*TRAIN* Accuracy Score => 0.9451327433628318
*TEST* Accuracy Score => 0.6597345132743363
... Cross Validation begins...
(9040, 6458)
(9040, 1)
[ 904 905 906 ... 9037 9038 9039] [ 0 1 2 ... 901 902 903]
[ 0 1 2 ... 9037 9038 9039] [ 904 905 906 ... 1805 1806 1807]
[ 0 1 2 ... 9037 9038 9039] [1808 1809 1810 ... 2709 2710 2711]
[ 0 1 2 ... 9037 9038 9039] [2712 2713 2714 ... 3613 3614 3615]
[ 0 1 2 ... 9037 9038 9039] [3616 3617 3618 ... 4517 4518 4519]
[ 0 1 2 ... 9037 9038 9039] [4520 4521 4522 ... 5421 5422 5423]
[ 0 1 2 ... 9037 9038 9039] [5424 5425 5426 ... 6325 6326 6327]
[ 0 1 2 ... 9037 9038 9039] [6328 6329 6330 ... 7229 7230 7231]
[ 0 1 2 ... 9037 9038 9039] [7232 7233 7234 ... 8133 8134 8135]
[ 0 1 2 ... 8133 8134 8135] [8136 8137 8138 ... 9037 9038 9039]
Finally :: 0.32499999999999996
>>>
Edit -1- Adding values of "e"
[0.08075221238938053, 0.413716814159292, 0.05752212389380531, 0.15376106194690264, 0.14712389380530974, 0.4668141592920354, 0.6946902654867256, 0.7112831858407079, 0.33738938053097345, 0.18694690265486727]
Edit -2- Adding shuffle=True
parameter to KFold()
Result:
[ 0 1 2 ... 9037 9038 9039] [ 4 5 10 ... 9007 9011 9024]
[ 0 1 2 ... 9037 9038 9039] [ 21 43 44 ... 9018 9035 9036]
[ 0 2 3 ... 9037 9038 9039] [ 1 20 60 ... 9023 9031 9034]
[ 0 1 2 ... 9036 9037 9038] [ 6 25 27 ... 9010 9025 9039]
[ 0 1 2 ... 9037 9038 9039] [ 15 16 28 ... 9029 9030 9033]
[ 0 1 2 ... 9037 9038 9039] [ 3 12 40 ... 9015 9017 9028]
[ 0 1 2 ... 9037 9038 9039] [ 7 8 23 ... 9013 9014 9027]
[ 0 1 3 ... 9035 9036 9039] [ 2 18 19 ... 9019 9037 9038]
[ 0 1 2 ... 9037 9038 9039] [ 24 37 39 ... 9012 9016 9026]
[ 1 2 3 ... 9037 9038 9039] [ 0 9 14 ... 9020 9021 9032]
[0.6504424778761062, 0.6736725663716814, 0.6969026548672567, 0.6692477876106194, 0.6769911504424779, 0.6382743362831859, 0.6692477876106194, 0.6648230088495575, 0.6648230088495575, 0.6814159292035398]
Finally :: 0.6685840707964601
cross-validation multiclass-classification prediction
$endgroup$
1
$begingroup$
Could you report all ten values in e?
$endgroup$
– Ben Reiniger
Apr 4 at 2:03
2
$begingroup$
I would like to add one point (just realized) - The input data is sorted based on their output classes. Ex. say output class 'A' is set of records indices 1-40, class 'B' is record indices from 41-67, class 'C' is record indices from 68-118, etc. Should I take that with shuffle?
$endgroup$
– ranit.b
Apr 4 at 10:59
3
$begingroup$
Wow! I just addedshuffle=True
parameter toKFold()
and the prediction accuracy became 66.85%. I'll add details to bottom of my post.
$endgroup$
– ranit.b
Apr 4 at 11:12
2
$begingroup$
I was thinking the same thing, especially when I saw the wildly varying scores across folds. I think you should post this as an answer.
$endgroup$
– Ben Reiniger
Apr 4 at 11:38
2
$begingroup$
Your comment above suggests you are doing multi-class classification. If that's the case then you should perform stratified cross-validation (i.e. useStratifiedKFold
).
$endgroup$
– bradS
Apr 4 at 11:58
|
show 7 more comments
$begingroup$
My training accuracy was better than my test accuracy, hence I thought my model was over-fitted and tried Cross-validation. The model further degraded. Is that my input data need to be sanitised further and of better quality?
Please share your thoughts what could be getting wrong here.
My data distribution:
Code snippets...
My function get_score
:
def get_score(model, X_train, X_test, y_train, y_test):
model.fit(X_train, y_train.values.ravel())
pred0 = model.predict(X_test)
return accuracy_score(y_test, pred0)
Logic:
print('*TRAIN* Accuracy Score => '+str(accuracy_score(y_train, m.predict(X_train)))) # LinearSVC() used
print('*TEST* Accuracy Score => '+str(accuracy_score(y_test, pred))) # LinearSVC() used
print("... Cross Validation begins...")
y0 = pd.DataFrame(y)
y0.reset_index(drop=True, inplace=True)
print(X.shape)
print(y0.shape)
kf = KFold(n_splits = 10)
e = []
for train_index, test_index in kf.split(X):
X_train, X_test, y_train, y_test = X.iloc[train_index], X.iloc[test_index],y0.iloc[train_index], y0.iloc[test_index]
print(train_index, test_index)
e.append(get_score(LinearSVC(random_state=777),X_train, X_test, y_train, y_test))
print("Finally :: "+str(np.mean(e)))
Output:
*TRAIN* Accuracy Score => 0.9451327433628318
*TEST* Accuracy Score => 0.6597345132743363
... Cross Validation begins...
(9040, 6458)
(9040, 1)
[ 904 905 906 ... 9037 9038 9039] [ 0 1 2 ... 901 902 903]
[ 0 1 2 ... 9037 9038 9039] [ 904 905 906 ... 1805 1806 1807]
[ 0 1 2 ... 9037 9038 9039] [1808 1809 1810 ... 2709 2710 2711]
[ 0 1 2 ... 9037 9038 9039] [2712 2713 2714 ... 3613 3614 3615]
[ 0 1 2 ... 9037 9038 9039] [3616 3617 3618 ... 4517 4518 4519]
[ 0 1 2 ... 9037 9038 9039] [4520 4521 4522 ... 5421 5422 5423]
[ 0 1 2 ... 9037 9038 9039] [5424 5425 5426 ... 6325 6326 6327]
[ 0 1 2 ... 9037 9038 9039] [6328 6329 6330 ... 7229 7230 7231]
[ 0 1 2 ... 9037 9038 9039] [7232 7233 7234 ... 8133 8134 8135]
[ 0 1 2 ... 8133 8134 8135] [8136 8137 8138 ... 9037 9038 9039]
Finally :: 0.32499999999999996
>>>
Edit -1- Adding values of "e"
[0.08075221238938053, 0.413716814159292, 0.05752212389380531, 0.15376106194690264, 0.14712389380530974, 0.4668141592920354, 0.6946902654867256, 0.7112831858407079, 0.33738938053097345, 0.18694690265486727]
Edit -2- Adding shuffle=True
parameter to KFold()
Result:
[ 0 1 2 ... 9037 9038 9039] [ 4 5 10 ... 9007 9011 9024]
[ 0 1 2 ... 9037 9038 9039] [ 21 43 44 ... 9018 9035 9036]
[ 0 2 3 ... 9037 9038 9039] [ 1 20 60 ... 9023 9031 9034]
[ 0 1 2 ... 9036 9037 9038] [ 6 25 27 ... 9010 9025 9039]
[ 0 1 2 ... 9037 9038 9039] [ 15 16 28 ... 9029 9030 9033]
[ 0 1 2 ... 9037 9038 9039] [ 3 12 40 ... 9015 9017 9028]
[ 0 1 2 ... 9037 9038 9039] [ 7 8 23 ... 9013 9014 9027]
[ 0 1 3 ... 9035 9036 9039] [ 2 18 19 ... 9019 9037 9038]
[ 0 1 2 ... 9037 9038 9039] [ 24 37 39 ... 9012 9016 9026]
[ 1 2 3 ... 9037 9038 9039] [ 0 9 14 ... 9020 9021 9032]
[0.6504424778761062, 0.6736725663716814, 0.6969026548672567, 0.6692477876106194, 0.6769911504424779, 0.6382743362831859, 0.6692477876106194, 0.6648230088495575, 0.6648230088495575, 0.6814159292035398]
Finally :: 0.6685840707964601
cross-validation multiclass-classification prediction
$endgroup$
My training accuracy was better than my test accuracy, hence I thought my model was over-fitted and tried Cross-validation. The model further degraded. Is that my input data need to be sanitised further and of better quality?
Please share your thoughts what could be getting wrong here.
My data distribution:
Code snippets...
My function get_score
:
def get_score(model, X_train, X_test, y_train, y_test):
model.fit(X_train, y_train.values.ravel())
pred0 = model.predict(X_test)
return accuracy_score(y_test, pred0)
Logic:
print('*TRAIN* Accuracy Score => '+str(accuracy_score(y_train, m.predict(X_train)))) # LinearSVC() used
print('*TEST* Accuracy Score => '+str(accuracy_score(y_test, pred))) # LinearSVC() used
print("... Cross Validation begins...")
y0 = pd.DataFrame(y)
y0.reset_index(drop=True, inplace=True)
print(X.shape)
print(y0.shape)
kf = KFold(n_splits = 10)
e = []
for train_index, test_index in kf.split(X):
X_train, X_test, y_train, y_test = X.iloc[train_index], X.iloc[test_index],y0.iloc[train_index], y0.iloc[test_index]
print(train_index, test_index)
e.append(get_score(LinearSVC(random_state=777),X_train, X_test, y_train, y_test))
print("Finally :: "+str(np.mean(e)))
Output:
*TRAIN* Accuracy Score => 0.9451327433628318
*TEST* Accuracy Score => 0.6597345132743363
... Cross Validation begins...
(9040, 6458)
(9040, 1)
[ 904 905 906 ... 9037 9038 9039] [ 0 1 2 ... 901 902 903]
[ 0 1 2 ... 9037 9038 9039] [ 904 905 906 ... 1805 1806 1807]
[ 0 1 2 ... 9037 9038 9039] [1808 1809 1810 ... 2709 2710 2711]
[ 0 1 2 ... 9037 9038 9039] [2712 2713 2714 ... 3613 3614 3615]
[ 0 1 2 ... 9037 9038 9039] [3616 3617 3618 ... 4517 4518 4519]
[ 0 1 2 ... 9037 9038 9039] [4520 4521 4522 ... 5421 5422 5423]
[ 0 1 2 ... 9037 9038 9039] [5424 5425 5426 ... 6325 6326 6327]
[ 0 1 2 ... 9037 9038 9039] [6328 6329 6330 ... 7229 7230 7231]
[ 0 1 2 ... 9037 9038 9039] [7232 7233 7234 ... 8133 8134 8135]
[ 0 1 2 ... 8133 8134 8135] [8136 8137 8138 ... 9037 9038 9039]
Finally :: 0.32499999999999996
>>>
Edit -1- Adding values of "e"
[0.08075221238938053, 0.413716814159292, 0.05752212389380531, 0.15376106194690264, 0.14712389380530974, 0.4668141592920354, 0.6946902654867256, 0.7112831858407079, 0.33738938053097345, 0.18694690265486727]
Edit -2- Adding shuffle=True
parameter to KFold()
Result:
[ 0 1 2 ... 9037 9038 9039] [ 4 5 10 ... 9007 9011 9024]
[ 0 1 2 ... 9037 9038 9039] [ 21 43 44 ... 9018 9035 9036]
[ 0 2 3 ... 9037 9038 9039] [ 1 20 60 ... 9023 9031 9034]
[ 0 1 2 ... 9036 9037 9038] [ 6 25 27 ... 9010 9025 9039]
[ 0 1 2 ... 9037 9038 9039] [ 15 16 28 ... 9029 9030 9033]
[ 0 1 2 ... 9037 9038 9039] [ 3 12 40 ... 9015 9017 9028]
[ 0 1 2 ... 9037 9038 9039] [ 7 8 23 ... 9013 9014 9027]
[ 0 1 3 ... 9035 9036 9039] [ 2 18 19 ... 9019 9037 9038]
[ 0 1 2 ... 9037 9038 9039] [ 24 37 39 ... 9012 9016 9026]
[ 1 2 3 ... 9037 9038 9039] [ 0 9 14 ... 9020 9021 9032]
[0.6504424778761062, 0.6736725663716814, 0.6969026548672567, 0.6692477876106194, 0.6769911504424779, 0.6382743362831859, 0.6692477876106194, 0.6648230088495575, 0.6648230088495575, 0.6814159292035398]
Finally :: 0.6685840707964601
cross-validation multiclass-classification prediction
cross-validation multiclass-classification prediction
edited Apr 4 at 11:15
ranit.b
asked Apr 3 at 13:36


ranit.branit.b
808
808
1
$begingroup$
Could you report all ten values in e?
$endgroup$
– Ben Reiniger
Apr 4 at 2:03
2
$begingroup$
I would like to add one point (just realized) - The input data is sorted based on their output classes. Ex. say output class 'A' is set of records indices 1-40, class 'B' is record indices from 41-67, class 'C' is record indices from 68-118, etc. Should I take that with shuffle?
$endgroup$
– ranit.b
Apr 4 at 10:59
3
$begingroup$
Wow! I just addedshuffle=True
parameter toKFold()
and the prediction accuracy became 66.85%. I'll add details to bottom of my post.
$endgroup$
– ranit.b
Apr 4 at 11:12
2
$begingroup$
I was thinking the same thing, especially when I saw the wildly varying scores across folds. I think you should post this as an answer.
$endgroup$
– Ben Reiniger
Apr 4 at 11:38
2
$begingroup$
Your comment above suggests you are doing multi-class classification. If that's the case then you should perform stratified cross-validation (i.e. useStratifiedKFold
).
$endgroup$
– bradS
Apr 4 at 11:58
|
show 7 more comments
1
$begingroup$
Could you report all ten values in e?
$endgroup$
– Ben Reiniger
Apr 4 at 2:03
2
$begingroup$
I would like to add one point (just realized) - The input data is sorted based on their output classes. Ex. say output class 'A' is set of records indices 1-40, class 'B' is record indices from 41-67, class 'C' is record indices from 68-118, etc. Should I take that with shuffle?
$endgroup$
– ranit.b
Apr 4 at 10:59
3
$begingroup$
Wow! I just addedshuffle=True
parameter toKFold()
and the prediction accuracy became 66.85%. I'll add details to bottom of my post.
$endgroup$
– ranit.b
Apr 4 at 11:12
2
$begingroup$
I was thinking the same thing, especially when I saw the wildly varying scores across folds. I think you should post this as an answer.
$endgroup$
– Ben Reiniger
Apr 4 at 11:38
2
$begingroup$
Your comment above suggests you are doing multi-class classification. If that's the case then you should perform stratified cross-validation (i.e. useStratifiedKFold
).
$endgroup$
– bradS
Apr 4 at 11:58
1
1
$begingroup$
Could you report all ten values in e?
$endgroup$
– Ben Reiniger
Apr 4 at 2:03
$begingroup$
Could you report all ten values in e?
$endgroup$
– Ben Reiniger
Apr 4 at 2:03
2
2
$begingroup$
I would like to add one point (just realized) - The input data is sorted based on their output classes. Ex. say output class 'A' is set of records indices 1-40, class 'B' is record indices from 41-67, class 'C' is record indices from 68-118, etc. Should I take that with shuffle?
$endgroup$
– ranit.b
Apr 4 at 10:59
$begingroup$
I would like to add one point (just realized) - The input data is sorted based on their output classes. Ex. say output class 'A' is set of records indices 1-40, class 'B' is record indices from 41-67, class 'C' is record indices from 68-118, etc. Should I take that with shuffle?
$endgroup$
– ranit.b
Apr 4 at 10:59
3
3
$begingroup$
Wow! I just added
shuffle=True
parameter to KFold()
and the prediction accuracy became 66.85%. I'll add details to bottom of my post.$endgroup$
– ranit.b
Apr 4 at 11:12
$begingroup$
Wow! I just added
shuffle=True
parameter to KFold()
and the prediction accuracy became 66.85%. I'll add details to bottom of my post.$endgroup$
– ranit.b
Apr 4 at 11:12
2
2
$begingroup$
I was thinking the same thing, especially when I saw the wildly varying scores across folds. I think you should post this as an answer.
$endgroup$
– Ben Reiniger
Apr 4 at 11:38
$begingroup$
I was thinking the same thing, especially when I saw the wildly varying scores across folds. I think you should post this as an answer.
$endgroup$
– Ben Reiniger
Apr 4 at 11:38
2
2
$begingroup$
Your comment above suggests you are doing multi-class classification. If that's the case then you should perform stratified cross-validation (i.e. use
StratifiedKFold
).$endgroup$
– bradS
Apr 4 at 11:58
$begingroup$
Your comment above suggests you are doing multi-class classification. If that's the case then you should perform stratified cross-validation (i.e. use
StratifiedKFold
).$endgroup$
– bradS
Apr 4 at 11:58
|
show 7 more comments
0
active
oldest
votes
Your Answer
StackExchange.ready(function()
var channelOptions =
tags: "".split(" "),
id: "557"
;
initTagRenderer("".split(" "), "".split(" "), channelOptions);
StackExchange.using("externalEditor", function()
// Have to fire editor after snippets, if snippets enabled
if (StackExchange.settings.snippets.snippetsEnabled)
StackExchange.using("snippets", function()
createEditor();
);
else
createEditor();
);
function createEditor()
StackExchange.prepareEditor(
heartbeatType: 'answer',
autoActivateHeartbeat: false,
convertImagesToLinks: false,
noModals: true,
showLowRepImageUploadWarning: true,
reputationToPostImages: null,
bindNavPrevention: true,
postfix: "",
imageUploader:
brandingHtml: "Powered by u003ca class="icon-imgur-white" href="https://imgur.com/"u003eu003c/au003e",
contentPolicyHtml: "User contributions licensed under u003ca href="https://creativecommons.org/licenses/by-sa/3.0/"u003ecc by-sa 3.0 with attribution requiredu003c/au003e u003ca href="https://stackoverflow.com/legal/content-policy"u003e(content policy)u003c/au003e",
allowUrls: true
,
onDemand: true,
discardSelector: ".discard-answer"
,immediatelyShowMarkdownHelp:true
);
);
Sign up or log in
StackExchange.ready(function ()
StackExchange.helpers.onClickDraftSave('#login-link');
);
Sign up using Google
Sign up using Facebook
Sign up using Email and Password
Post as a guest
Required, but never shown
StackExchange.ready(
function ()
StackExchange.openid.initPostLogin('.new-post-login', 'https%3a%2f%2fdatascience.stackexchange.com%2fquestions%2f48525%2fwhy-does-cv-yield-lower-score%23new-answer', 'question_page');
);
Post as a guest
Required, but never shown
0
active
oldest
votes
0
active
oldest
votes
active
oldest
votes
active
oldest
votes
Thanks for contributing an answer to Data Science Stack Exchange!
- Please be sure to answer the question. Provide details and share your research!
But avoid …
- Asking for help, clarification, or responding to other answers.
- Making statements based on opinion; back them up with references or personal experience.
Use MathJax to format equations. MathJax reference.
To learn more, see our tips on writing great answers.
Sign up or log in
StackExchange.ready(function ()
StackExchange.helpers.onClickDraftSave('#login-link');
);
Sign up using Google
Sign up using Facebook
Sign up using Email and Password
Post as a guest
Required, but never shown
StackExchange.ready(
function ()
StackExchange.openid.initPostLogin('.new-post-login', 'https%3a%2f%2fdatascience.stackexchange.com%2fquestions%2f48525%2fwhy-does-cv-yield-lower-score%23new-answer', 'question_page');
);
Post as a guest
Required, but never shown
Sign up or log in
StackExchange.ready(function ()
StackExchange.helpers.onClickDraftSave('#login-link');
);
Sign up using Google
Sign up using Facebook
Sign up using Email and Password
Post as a guest
Required, but never shown
Sign up or log in
StackExchange.ready(function ()
StackExchange.helpers.onClickDraftSave('#login-link');
);
Sign up using Google
Sign up using Facebook
Sign up using Email and Password
Post as a guest
Required, but never shown
Sign up or log in
StackExchange.ready(function ()
StackExchange.helpers.onClickDraftSave('#login-link');
);
Sign up using Google
Sign up using Facebook
Sign up using Email and Password
Sign up using Google
Sign up using Facebook
Sign up using Email and Password
Post as a guest
Required, but never shown
Required, but never shown
Required, but never shown
Required, but never shown
Required, but never shown
Required, but never shown
Required, but never shown
Required, but never shown
Required, but never shown
e6bto2 BoyhKkwfVs2IOmx3XdDLUJa2fszuhNi cjnGrA4qOhAMMNBPb huWSR dTJv8UiDstkCOHv M088MdJBRRmSfEajd
1
$begingroup$
Could you report all ten values in e?
$endgroup$
– Ben Reiniger
Apr 4 at 2:03
2
$begingroup$
I would like to add one point (just realized) - The input data is sorted based on their output classes. Ex. say output class 'A' is set of records indices 1-40, class 'B' is record indices from 41-67, class 'C' is record indices from 68-118, etc. Should I take that with shuffle?
$endgroup$
– ranit.b
Apr 4 at 10:59
3
$begingroup$
Wow! I just added
shuffle=True
parameter toKFold()
and the prediction accuracy became 66.85%. I'll add details to bottom of my post.$endgroup$
– ranit.b
Apr 4 at 11:12
2
$begingroup$
I was thinking the same thing, especially when I saw the wildly varying scores across folds. I think you should post this as an answer.
$endgroup$
– Ben Reiniger
Apr 4 at 11:38
2
$begingroup$
Your comment above suggests you are doing multi-class classification. If that's the case then you should perform stratified cross-validation (i.e. use
StratifiedKFold
).$endgroup$
– bradS
Apr 4 at 11:58