Extract most informative parts of text from documentsShould I use regex or machine learning?NLP to recognize the meaning of a paragraphUnsupervised feature learning for NERHow do you compare term counts between two different periods, with different underlying corpus sizes, without bias?How to extract paragraphs from text document?How to extract titles from documents?How to extract specific parts of text from a string?Text understanding and mappingWhere can I find a dataset for long sequence text chunking?Are word embeddings further updated during training for document classification?Apply Labeled LDA on large data
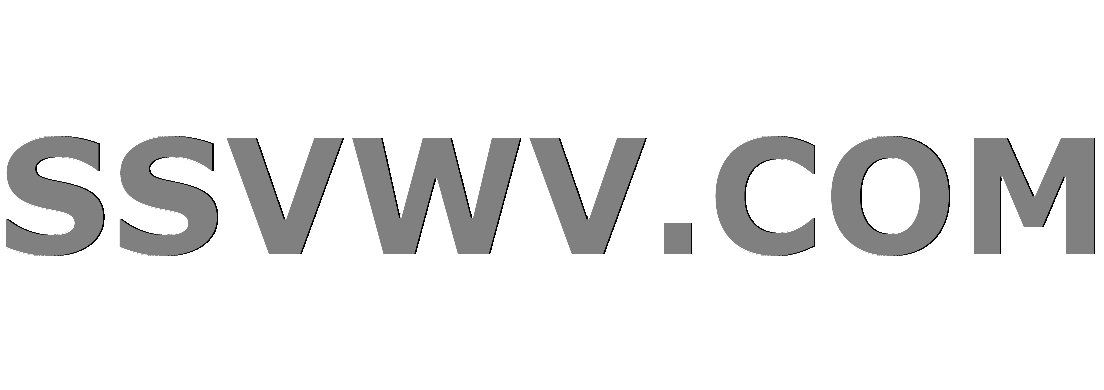
Multi tool use
Angel of Condemnation - Exile creature with second ability
Can I still be respawned if I die by falling off the map?
Calculate sum of polynomial roots
Unexpected behavior of the procedure `Area` on the object 'Polygon'
Why should universal income be universal?
Is there an injective, monotonically increasing, strictly concave function from the reals, to the reals?
Why did the EU agree to delay the Brexit deadline?
When were female captains banned from Starfleet?
What does "Scientists rise up against statistical significance" mean? (Comment in Nature)
What is Cash Advance APR?
15% tax on $7.5k earnings. Is that right?
Can I say "fingers" when referring to toes?
Why Shazam when there is already Superman?
What exact color does ozone gas have?
Hero deduces identity of a killer
Do the primes contain an infinite almost arithmetic progression?
Can a College of Swords bard use a Blade Flourish option on an opportunity attack provoked by their own Dissonant Whispers spell?
It grows, but water kills it
How do you make your own symbol when Detexify fails?
Can disgust be a key component of horror?
Electoral considerations aside, what are potential benefits, for the US, of policy changes proposed by the tweet recognizing Golan annexation?
Is there a RAID 0 Equivalent for RAM?
Plot of a tornado-shaped surface
Does Doodling or Improvising on the Piano Have Any Benefits?
Extract most informative parts of text from documents
Should I use regex or machine learning?NLP to recognize the meaning of a paragraphUnsupervised feature learning for NERHow do you compare term counts between two different periods, with different underlying corpus sizes, without bias?How to extract paragraphs from text document?How to extract titles from documents?How to extract specific parts of text from a string?Text understanding and mappingWhere can I find a dataset for long sequence text chunking?Are word embeddings further updated during training for document classification?Apply Labeled LDA on large data
$begingroup$
Are there any articles or discussions about extracting part of text that holds the most of information about current document.
For example, I have a large corpus of documents from the same domain. There are parts of text that hold the key information what single document talks about. I want to extract some of those parts and use them as kind of a summary of the text. Is there any useful documentation about how to achieve something like this.
It would be really helpful if someone could point me into the right direction what I should search for or read to get some insight in work that might have already been done in this field of Natural language processing.
nlp text-mining
$endgroup$
add a comment |
$begingroup$
Are there any articles or discussions about extracting part of text that holds the most of information about current document.
For example, I have a large corpus of documents from the same domain. There are parts of text that hold the key information what single document talks about. I want to extract some of those parts and use them as kind of a summary of the text. Is there any useful documentation about how to achieve something like this.
It would be really helpful if someone could point me into the right direction what I should search for or read to get some insight in work that might have already been done in this field of Natural language processing.
nlp text-mining
$endgroup$
add a comment |
$begingroup$
Are there any articles or discussions about extracting part of text that holds the most of information about current document.
For example, I have a large corpus of documents from the same domain. There are parts of text that hold the key information what single document talks about. I want to extract some of those parts and use them as kind of a summary of the text. Is there any useful documentation about how to achieve something like this.
It would be really helpful if someone could point me into the right direction what I should search for or read to get some insight in work that might have already been done in this field of Natural language processing.
nlp text-mining
$endgroup$
Are there any articles or discussions about extracting part of text that holds the most of information about current document.
For example, I have a large corpus of documents from the same domain. There are parts of text that hold the key information what single document talks about. I want to extract some of those parts and use them as kind of a summary of the text. Is there any useful documentation about how to achieve something like this.
It would be really helpful if someone could point me into the right direction what I should search for or read to get some insight in work that might have already been done in this field of Natural language processing.
nlp text-mining
nlp text-mining
asked Dec 8 '14 at 14:51


MaticDibaMaticDiba
4211410
4211410
add a comment |
add a comment |
2 Answers
2
active
oldest
votes
$begingroup$
What you're describing is often achieved using a simple combination of TF-IDF and extractive summarization.
In a nutshell, TF-IDF tells you the relative importance of each word in each document, in comparison to the rest of your corpus. At this point, you have a score for each word in each document approximating its "importance." Then you can use these individual word scores to compute a composite score for each sentence by summing the scores of each word in each sentence. Finally, simply take the top-N scoring sentences from each document as its summary.
Earlier this year, I put together an iPython Notebook that culminates with an implementation of this in Python using NLTK and Scikit-learn: A Smattering of NLP in Python.
$endgroup$
2
$begingroup$
Yes, that would probably be it. I could also add additional weights to some words, that I already know that are informative. Thanks for your help and useful links.
$endgroup$
– MaticDiba
Dec 9 '14 at 9:33
$begingroup$
So can i use this on a pdf? :)
$endgroup$
– Adam
Apr 14 '17 at 22:25
$begingroup$
Yes, you can use this on the text in a PDF, assuming you've already extracted the plain text from the PDF using something like pdftotext.
$endgroup$
– Charlie Greenbacker
Apr 15 '17 at 23:26
add a comment |
$begingroup$
Lots of keyword extraction techniques out there depend on factors like:
- Grammatical quality of text
- Length of text
- Whether you are looking for a single keyword or phrasal keyword etc.
But in general, if you have a long text and you want to extract keywords automatically from that, I would recommend you to go through follow articles:
TextRank
RAKE [Rapid Automatic Keyword Extraction]
Topica
Also to extract custom (special) keywords which is not coming through the above techniques, have a look at the post below:
Extract Custom Keywords using NLTK POS tagger in python
New contributor
anindya is a new contributor to this site. Take care in asking for clarification, commenting, and answering.
Check out our Code of Conduct.
$endgroup$
add a comment |
Your Answer
StackExchange.ifUsing("editor", function ()
return StackExchange.using("mathjaxEditing", function ()
StackExchange.MarkdownEditor.creationCallbacks.add(function (editor, postfix)
StackExchange.mathjaxEditing.prepareWmdForMathJax(editor, postfix, [["$", "$"], ["\\(","\\)"]]);
);
);
, "mathjax-editing");
StackExchange.ready(function()
var channelOptions =
tags: "".split(" "),
id: "557"
;
initTagRenderer("".split(" "), "".split(" "), channelOptions);
StackExchange.using("externalEditor", function()
// Have to fire editor after snippets, if snippets enabled
if (StackExchange.settings.snippets.snippetsEnabled)
StackExchange.using("snippets", function()
createEditor();
);
else
createEditor();
);
function createEditor()
StackExchange.prepareEditor(
heartbeatType: 'answer',
autoActivateHeartbeat: false,
convertImagesToLinks: false,
noModals: true,
showLowRepImageUploadWarning: true,
reputationToPostImages: null,
bindNavPrevention: true,
postfix: "",
imageUploader:
brandingHtml: "Powered by u003ca class="icon-imgur-white" href="https://imgur.com/"u003eu003c/au003e",
contentPolicyHtml: "User contributions licensed under u003ca href="https://creativecommons.org/licenses/by-sa/3.0/"u003ecc by-sa 3.0 with attribution requiredu003c/au003e u003ca href="https://stackoverflow.com/legal/content-policy"u003e(content policy)u003c/au003e",
allowUrls: true
,
onDemand: true,
discardSelector: ".discard-answer"
,immediatelyShowMarkdownHelp:true
);
);
Sign up or log in
StackExchange.ready(function ()
StackExchange.helpers.onClickDraftSave('#login-link');
);
Sign up using Google
Sign up using Facebook
Sign up using Email and Password
Post as a guest
Required, but never shown
StackExchange.ready(
function ()
StackExchange.openid.initPostLogin('.new-post-login', 'https%3a%2f%2fdatascience.stackexchange.com%2fquestions%2f2646%2fextract-most-informative-parts-of-text-from-documents%23new-answer', 'question_page');
);
Post as a guest
Required, but never shown
2 Answers
2
active
oldest
votes
2 Answers
2
active
oldest
votes
active
oldest
votes
active
oldest
votes
$begingroup$
What you're describing is often achieved using a simple combination of TF-IDF and extractive summarization.
In a nutshell, TF-IDF tells you the relative importance of each word in each document, in comparison to the rest of your corpus. At this point, you have a score for each word in each document approximating its "importance." Then you can use these individual word scores to compute a composite score for each sentence by summing the scores of each word in each sentence. Finally, simply take the top-N scoring sentences from each document as its summary.
Earlier this year, I put together an iPython Notebook that culminates with an implementation of this in Python using NLTK and Scikit-learn: A Smattering of NLP in Python.
$endgroup$
2
$begingroup$
Yes, that would probably be it. I could also add additional weights to some words, that I already know that are informative. Thanks for your help and useful links.
$endgroup$
– MaticDiba
Dec 9 '14 at 9:33
$begingroup$
So can i use this on a pdf? :)
$endgroup$
– Adam
Apr 14 '17 at 22:25
$begingroup$
Yes, you can use this on the text in a PDF, assuming you've already extracted the plain text from the PDF using something like pdftotext.
$endgroup$
– Charlie Greenbacker
Apr 15 '17 at 23:26
add a comment |
$begingroup$
What you're describing is often achieved using a simple combination of TF-IDF and extractive summarization.
In a nutshell, TF-IDF tells you the relative importance of each word in each document, in comparison to the rest of your corpus. At this point, you have a score for each word in each document approximating its "importance." Then you can use these individual word scores to compute a composite score for each sentence by summing the scores of each word in each sentence. Finally, simply take the top-N scoring sentences from each document as its summary.
Earlier this year, I put together an iPython Notebook that culminates with an implementation of this in Python using NLTK and Scikit-learn: A Smattering of NLP in Python.
$endgroup$
2
$begingroup$
Yes, that would probably be it. I could also add additional weights to some words, that I already know that are informative. Thanks for your help and useful links.
$endgroup$
– MaticDiba
Dec 9 '14 at 9:33
$begingroup$
So can i use this on a pdf? :)
$endgroup$
– Adam
Apr 14 '17 at 22:25
$begingroup$
Yes, you can use this on the text in a PDF, assuming you've already extracted the plain text from the PDF using something like pdftotext.
$endgroup$
– Charlie Greenbacker
Apr 15 '17 at 23:26
add a comment |
$begingroup$
What you're describing is often achieved using a simple combination of TF-IDF and extractive summarization.
In a nutshell, TF-IDF tells you the relative importance of each word in each document, in comparison to the rest of your corpus. At this point, you have a score for each word in each document approximating its "importance." Then you can use these individual word scores to compute a composite score for each sentence by summing the scores of each word in each sentence. Finally, simply take the top-N scoring sentences from each document as its summary.
Earlier this year, I put together an iPython Notebook that culminates with an implementation of this in Python using NLTK and Scikit-learn: A Smattering of NLP in Python.
$endgroup$
What you're describing is often achieved using a simple combination of TF-IDF and extractive summarization.
In a nutshell, TF-IDF tells you the relative importance of each word in each document, in comparison to the rest of your corpus. At this point, you have a score for each word in each document approximating its "importance." Then you can use these individual word scores to compute a composite score for each sentence by summing the scores of each word in each sentence. Finally, simply take the top-N scoring sentences from each document as its summary.
Earlier this year, I put together an iPython Notebook that culminates with an implementation of this in Python using NLTK and Scikit-learn: A Smattering of NLP in Python.
answered Dec 8 '14 at 15:48
Charlie GreenbackerCharlie Greenbacker
1,32159
1,32159
2
$begingroup$
Yes, that would probably be it. I could also add additional weights to some words, that I already know that are informative. Thanks for your help and useful links.
$endgroup$
– MaticDiba
Dec 9 '14 at 9:33
$begingroup$
So can i use this on a pdf? :)
$endgroup$
– Adam
Apr 14 '17 at 22:25
$begingroup$
Yes, you can use this on the text in a PDF, assuming you've already extracted the plain text from the PDF using something like pdftotext.
$endgroup$
– Charlie Greenbacker
Apr 15 '17 at 23:26
add a comment |
2
$begingroup$
Yes, that would probably be it. I could also add additional weights to some words, that I already know that are informative. Thanks for your help and useful links.
$endgroup$
– MaticDiba
Dec 9 '14 at 9:33
$begingroup$
So can i use this on a pdf? :)
$endgroup$
– Adam
Apr 14 '17 at 22:25
$begingroup$
Yes, you can use this on the text in a PDF, assuming you've already extracted the plain text from the PDF using something like pdftotext.
$endgroup$
– Charlie Greenbacker
Apr 15 '17 at 23:26
2
2
$begingroup$
Yes, that would probably be it. I could also add additional weights to some words, that I already know that are informative. Thanks for your help and useful links.
$endgroup$
– MaticDiba
Dec 9 '14 at 9:33
$begingroup$
Yes, that would probably be it. I could also add additional weights to some words, that I already know that are informative. Thanks for your help and useful links.
$endgroup$
– MaticDiba
Dec 9 '14 at 9:33
$begingroup$
So can i use this on a pdf? :)
$endgroup$
– Adam
Apr 14 '17 at 22:25
$begingroup$
So can i use this on a pdf? :)
$endgroup$
– Adam
Apr 14 '17 at 22:25
$begingroup$
Yes, you can use this on the text in a PDF, assuming you've already extracted the plain text from the PDF using something like pdftotext.
$endgroup$
– Charlie Greenbacker
Apr 15 '17 at 23:26
$begingroup$
Yes, you can use this on the text in a PDF, assuming you've already extracted the plain text from the PDF using something like pdftotext.
$endgroup$
– Charlie Greenbacker
Apr 15 '17 at 23:26
add a comment |
$begingroup$
Lots of keyword extraction techniques out there depend on factors like:
- Grammatical quality of text
- Length of text
- Whether you are looking for a single keyword or phrasal keyword etc.
But in general, if you have a long text and you want to extract keywords automatically from that, I would recommend you to go through follow articles:
TextRank
RAKE [Rapid Automatic Keyword Extraction]
Topica
Also to extract custom (special) keywords which is not coming through the above techniques, have a look at the post below:
Extract Custom Keywords using NLTK POS tagger in python
New contributor
anindya is a new contributor to this site. Take care in asking for clarification, commenting, and answering.
Check out our Code of Conduct.
$endgroup$
add a comment |
$begingroup$
Lots of keyword extraction techniques out there depend on factors like:
- Grammatical quality of text
- Length of text
- Whether you are looking for a single keyword or phrasal keyword etc.
But in general, if you have a long text and you want to extract keywords automatically from that, I would recommend you to go through follow articles:
TextRank
RAKE [Rapid Automatic Keyword Extraction]
Topica
Also to extract custom (special) keywords which is not coming through the above techniques, have a look at the post below:
Extract Custom Keywords using NLTK POS tagger in python
New contributor
anindya is a new contributor to this site. Take care in asking for clarification, commenting, and answering.
Check out our Code of Conduct.
$endgroup$
add a comment |
$begingroup$
Lots of keyword extraction techniques out there depend on factors like:
- Grammatical quality of text
- Length of text
- Whether you are looking for a single keyword or phrasal keyword etc.
But in general, if you have a long text and you want to extract keywords automatically from that, I would recommend you to go through follow articles:
TextRank
RAKE [Rapid Automatic Keyword Extraction]
Topica
Also to extract custom (special) keywords which is not coming through the above techniques, have a look at the post below:
Extract Custom Keywords using NLTK POS tagger in python
New contributor
anindya is a new contributor to this site. Take care in asking for clarification, commenting, and answering.
Check out our Code of Conduct.
$endgroup$
Lots of keyword extraction techniques out there depend on factors like:
- Grammatical quality of text
- Length of text
- Whether you are looking for a single keyword or phrasal keyword etc.
But in general, if you have a long text and you want to extract keywords automatically from that, I would recommend you to go through follow articles:
TextRank
RAKE [Rapid Automatic Keyword Extraction]
Topica
Also to extract custom (special) keywords which is not coming through the above techniques, have a look at the post below:
Extract Custom Keywords using NLTK POS tagger in python
New contributor
anindya is a new contributor to this site. Take care in asking for clarification, commenting, and answering.
Check out our Code of Conduct.
edited Mar 19 at 15:01


Glorfindel
129119
129119
New contributor
anindya is a new contributor to this site. Take care in asking for clarification, commenting, and answering.
Check out our Code of Conduct.
answered Mar 19 at 9:09
anindyaanindya
1
1
New contributor
anindya is a new contributor to this site. Take care in asking for clarification, commenting, and answering.
Check out our Code of Conduct.
New contributor
anindya is a new contributor to this site. Take care in asking for clarification, commenting, and answering.
Check out our Code of Conduct.
anindya is a new contributor to this site. Take care in asking for clarification, commenting, and answering.
Check out our Code of Conduct.
add a comment |
add a comment |
Thanks for contributing an answer to Data Science Stack Exchange!
- Please be sure to answer the question. Provide details and share your research!
But avoid …
- Asking for help, clarification, or responding to other answers.
- Making statements based on opinion; back them up with references or personal experience.
Use MathJax to format equations. MathJax reference.
To learn more, see our tips on writing great answers.
Sign up or log in
StackExchange.ready(function ()
StackExchange.helpers.onClickDraftSave('#login-link');
);
Sign up using Google
Sign up using Facebook
Sign up using Email and Password
Post as a guest
Required, but never shown
StackExchange.ready(
function ()
StackExchange.openid.initPostLogin('.new-post-login', 'https%3a%2f%2fdatascience.stackexchange.com%2fquestions%2f2646%2fextract-most-informative-parts-of-text-from-documents%23new-answer', 'question_page');
);
Post as a guest
Required, but never shown
Sign up or log in
StackExchange.ready(function ()
StackExchange.helpers.onClickDraftSave('#login-link');
);
Sign up using Google
Sign up using Facebook
Sign up using Email and Password
Post as a guest
Required, but never shown
Sign up or log in
StackExchange.ready(function ()
StackExchange.helpers.onClickDraftSave('#login-link');
);
Sign up using Google
Sign up using Facebook
Sign up using Email and Password
Post as a guest
Required, but never shown
Sign up or log in
StackExchange.ready(function ()
StackExchange.helpers.onClickDraftSave('#login-link');
);
Sign up using Google
Sign up using Facebook
Sign up using Email and Password
Sign up using Google
Sign up using Facebook
Sign up using Email and Password
Post as a guest
Required, but never shown
Required, but never shown
Required, but never shown
Required, but never shown
Required, but never shown
Required, but never shown
Required, but never shown
Required, but never shown
Required, but never shown
7 HXBv8WDtFBvvIaIQyWbMXQ7 YnHkqPx OV,EHqaS8XYJSXdEdC6,sM,8